Non-Subtractive Dither for Squared Error Linearization of Lloyd-Max Quantizers.
Asilomar Conference on Signals, Systems and Computers(2023)
摘要
An adaptive non-subtractive dithering technique is proposed that makes the mean square quantization error of non-uniform Lloyd-Max quantizers constant over a given range of input values. This improves the error performance of analog-to-digital conversion systems by decorrelating the second moment of the quantization noise with the input signal. To do this we create a set of analytical conditions which any candidate dither distribution must satisfy to achieve this desired statistical decorrelation. We use this to derive bounds on the feasible mean square error of non-subtractively dither quantizers and solve for a solution class of dither distributions defined by a mixture of uniform and discrete random variables. Such distributions are particularly easy to generate in real-world hardware implementations. We then determine the analytically optimal parameters for this class of distributions to simultaneously minimize the average absolute first conditional quantization error moment and thus reduce the asymptotic bias of the quantizer. Finally we present numerical results verifying the efficacy of the proposed dither in linearizing the second conditional error moment of a Lloyd-Max quantizer over a wide input range while also reducing the first error moment, thereby improving the quantization error properties significantly.
更多查看译文
关键词
dithering,quantization,analog-to-digital conversion,linearization,lloyd-max
AI 理解论文
溯源树
样例
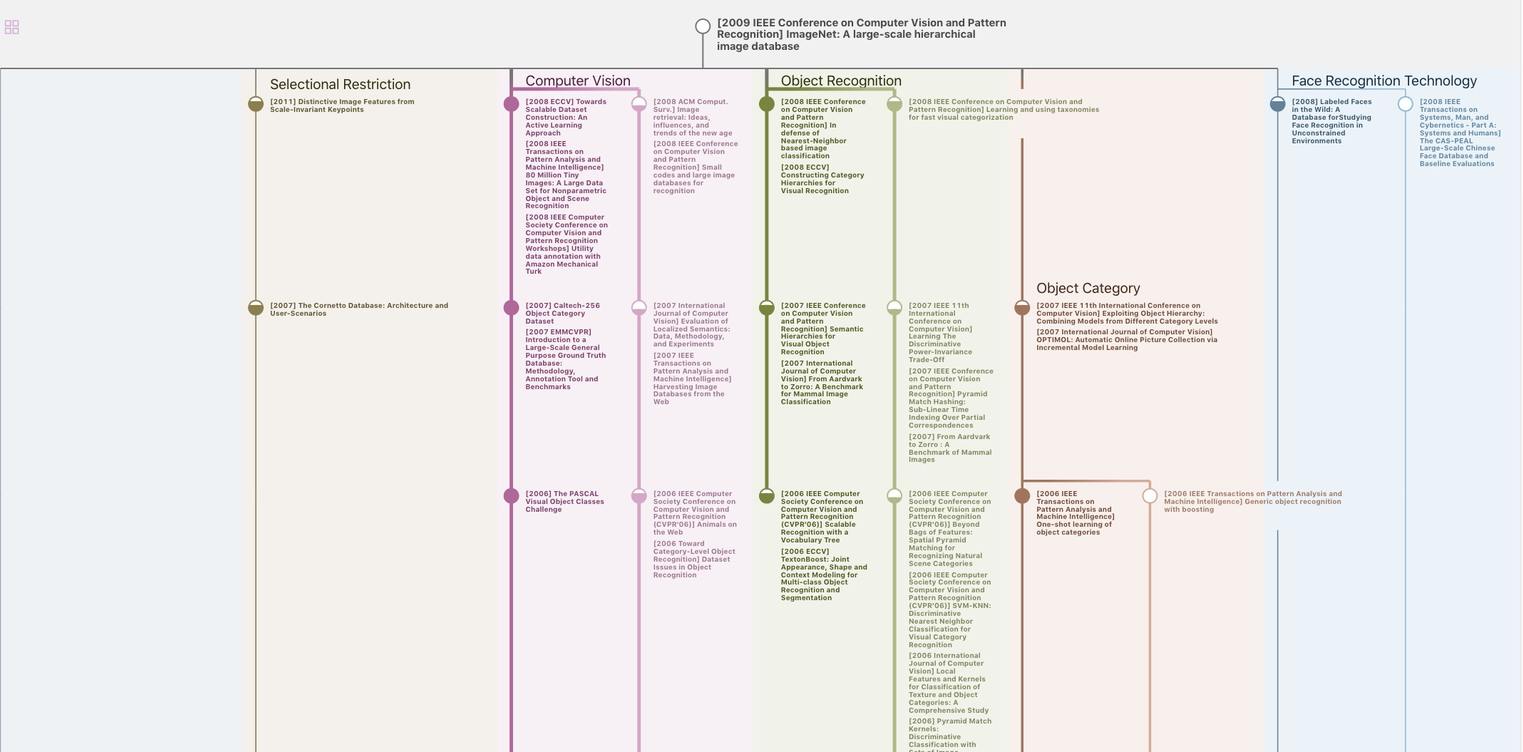
生成溯源树,研究论文发展脉络
Chat Paper
正在生成论文摘要