Heterogeneous Graph Contrastive Learning With Meta-Path Contexts and Adaptively Weighted Negative Samples
IEEE Transactions on Knowledge and Data Engineering(2024)
摘要
Heterogeneous graph contrastive learning has received wide attention recently. Some existing methods use meta-paths, which are sequences of object types that capture semantic relationships between objects, to construct contrastive views. However, most of them ignore the rich meta-path context information that describes how two objects are connected by meta-paths. Further, they fail to distinguish negative samples, which could adversely affect the model performance. To address the problems, we propose MEOW, which considers both meta-path contexts and weighted negative samples. Specifically, MEOW constructs a coarse view and a fine-grained view for contrast. The former reflects which objects are connected by meta-paths, while the latter uses meta-path contexts and characterizes details on how the objects are connected. Then, we theoretically analyze the InfoNCE loss and recognize its limitations for computing gradients of negative samples. To better distinguish negative samples, we learn hard-valued weights for them based on node clustering and use prototypical contrastive learning to pull close embeddings of nodes in the same cluster. In addition, we propose a variant model AdaMEOW that adaptively learns soft-valued weights of negative samples to further improve node representation. Finally, we conduct extensive experiments to show the superiority of MEOW and AdaMEOW against other state-of-the-art methods.
更多查看译文
关键词
Contrastive learning,heterogeneous information network,representation learning
AI 理解论文
溯源树
样例
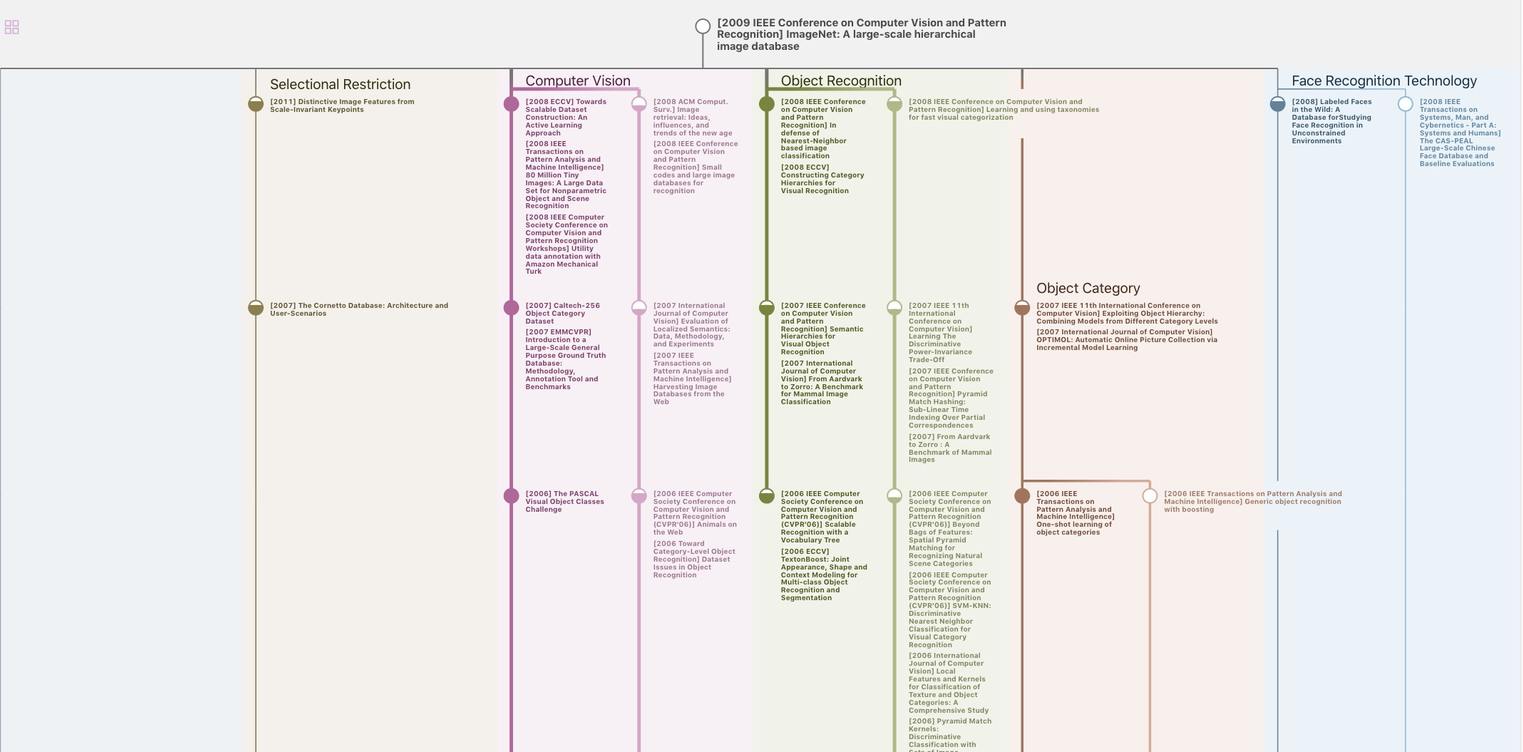
生成溯源树,研究论文发展脉络
Chat Paper
正在生成论文摘要