Nearly-tight Approximation Guarantees for the Improving Multi-Armed Bandits Problem
arxiv(2024)
摘要
We give nearly-tight upper and lower bounds for the improving multi-armed
bandits problem. An instance of this problem has k arms, each of whose reward
function is a concave and increasing function of the number of times that arm
has been pulled so far. We show that for any randomized online algorithm, there
exists an instance on which it must suffer at least an Ω(√(k))
approximation factor relative to the optimal reward. We then provide a
randomized online algorithm that guarantees an O(√(k)) approximation
factor, if it is told the maximum reward achievable by the optimal arm in
advance. We then show how to remove this assumption at the cost of an extra
O(log k) approximation factor, achieving an overall O(√(k)log k)
approximation relative to optimal.
更多查看译文
AI 理解论文
溯源树
样例
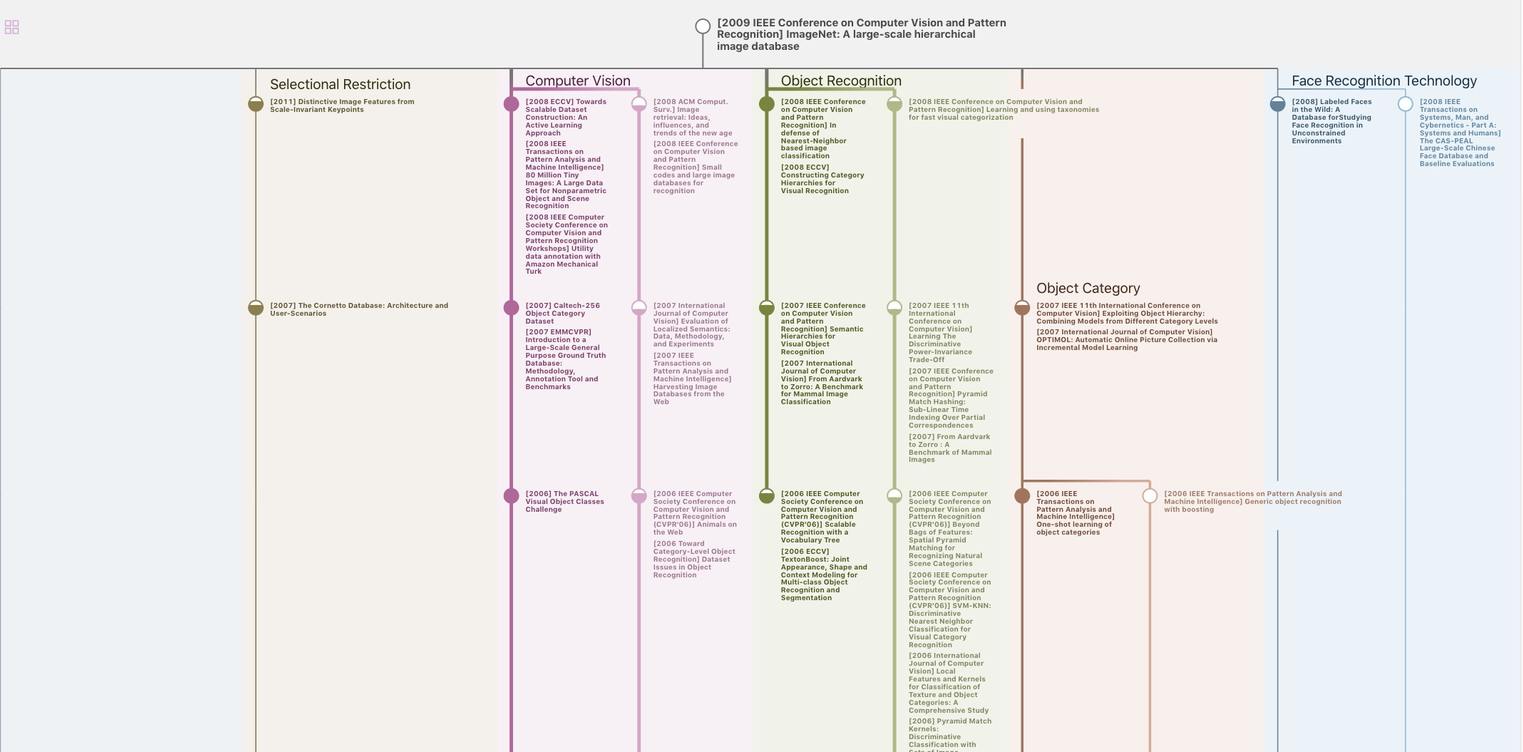
生成溯源树,研究论文发展脉络
Chat Paper
正在生成论文摘要