Taming the Elephants: Affordable Flow Length Prediction in the Data Plane
Proceedings of the ACM on Networking(2024)
摘要
Machine Learning (ML) shows promising potential for enhancing networking tasks by providing early traffic predictions. However, implementing an ML-enabled system is a challenging task due to network devices limited resources. While previous works have shown the feasibility of running simple ML models in the data plane, integrating them into a practical end-to-end system is not an easy task. It requires addressing issues related to resource management and model maintenance to ensure that the performance improvement justifies the system overhead. In this work, we propose DUMBO, a versatile end-to-end system to generate and exploit early flow size predictions at line rate. Our system seamlessly integrates and maintains a simple ML model that offers early coarse-grain flow size prediction in the data plane. We evaluate the proposed system on flow scheduling, per-flow packet inter-arrival time distribution, and flow size estimation using real traffic traces, and perform experiments using an FPGA prototype running on an AMD(R)-Xilinx(R) Alveo U280 SmartNIC. Our results show that DUMBO outperforms traditional state-of-the-art approaches by equipping network devices data planes with a lightweight ML model. Code is available at https://github.com/cpt-harlock/DUMBO.
更多查看译文
关键词
data plane,in-network machine learning,per-flow monitoring
AI 理解论文
溯源树
样例
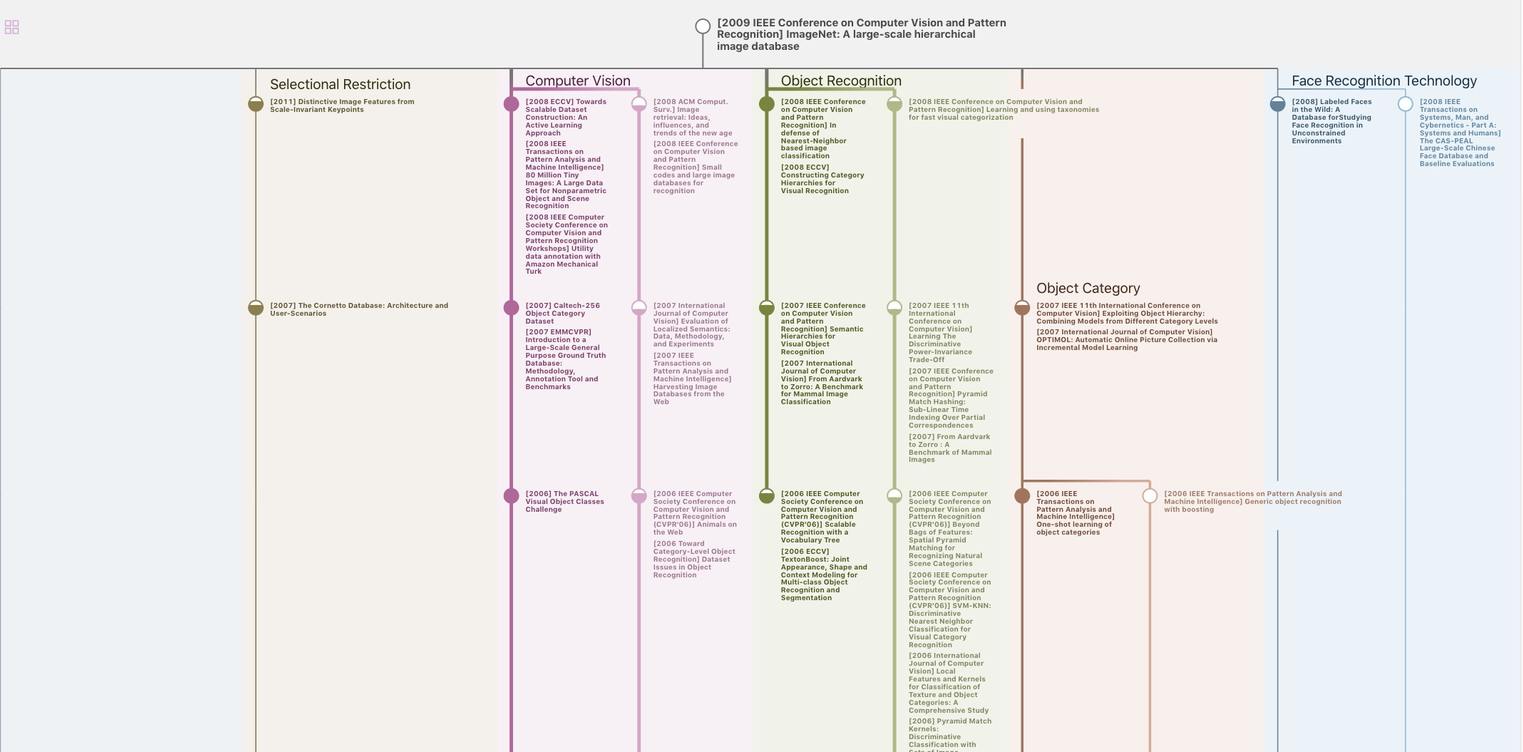
生成溯源树,研究论文发展脉络
Chat Paper
正在生成论文摘要