STaR-GATE: Teaching Language Models to Ask Clarifying Questions
CoRR(2024)
摘要
When prompting language models to complete a task, users often leave
important aspects unsaid. While asking questions could resolve this ambiguity
, models often struggle to ask good questions.
We explore a language model's ability to self-improve
by rewarding the model for generating useful
questions – a simple method we dub STaR-GATE. We generate a synthetic dataset
of 25,500 unique persona-task prompts to simulate conversations between a
pretrained language model – the – and a
whose preferences are unknown to the .
By asking questions, the elicits preferences from the
. The is iteratively finetuned on
questions that increase the probability of high-quality responses to the task,
which are generated by an with access to the
's latent preferences. After two iterations of
self-improvement, the asks better questions, allowing it to
generate responses that are preferred over responses from the initial model on
72% of tasks. Our results indicate that teaching a
language model to ask better questions leads to better personalized responses.
更多查看译文
AI 理解论文
溯源树
样例
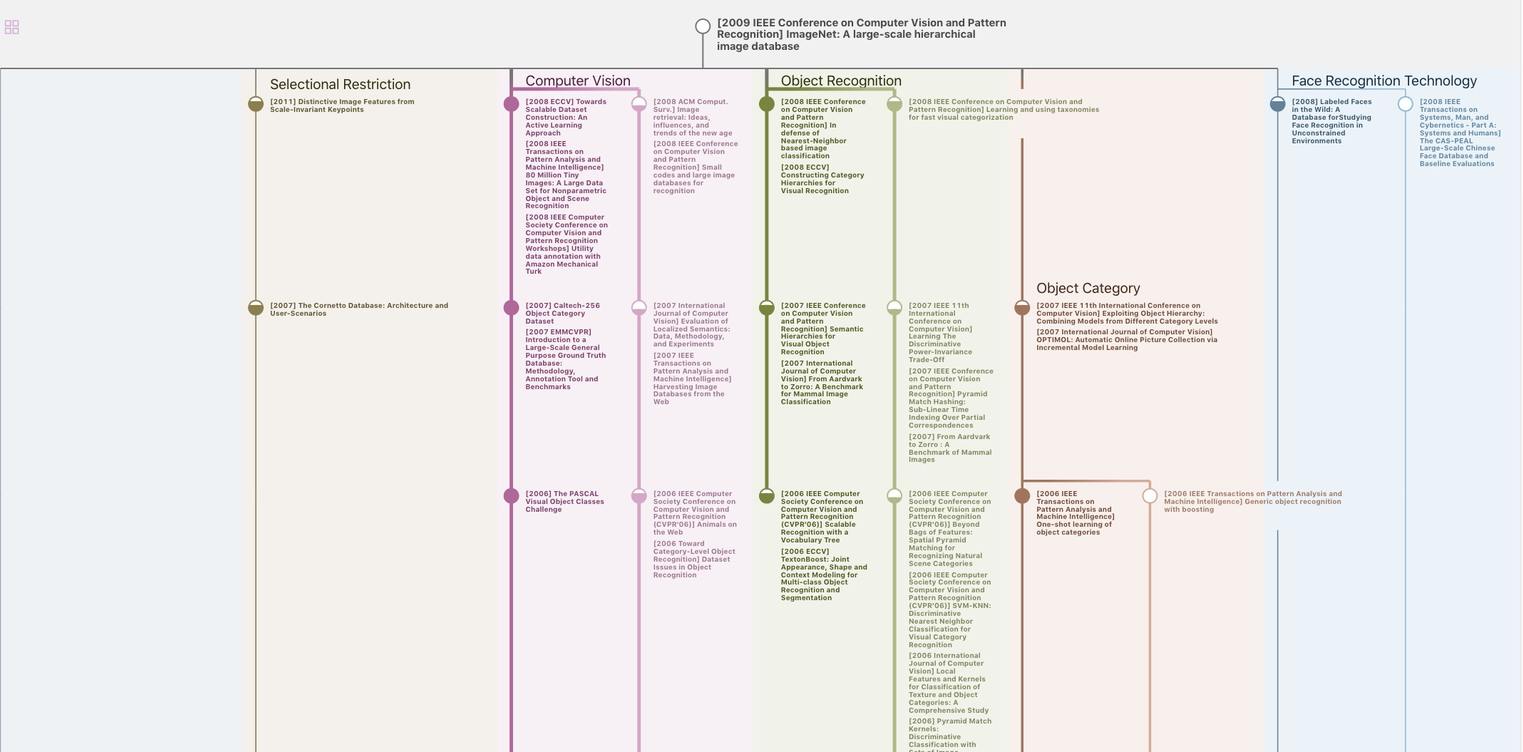
生成溯源树,研究论文发展脉络
Chat Paper
正在生成论文摘要