Digital twin-assisted intelligent anomaly detection system for Internet of Things
Ad Hoc Networks(2024)
摘要
The proliferation of Internet of Things (IoT) devices within the cyber–physical infrastructure has raised privacy and security concerns. This is because processing large amounts of data and extracting anomalous data cannot be imprecisely identified with the limited storage and processing capabilities of IoT devices. Against this backdrop, edge computing is a networking paradigm to reshape the next generation of IoT-based applications, which brings computation and data storage closer to the data sources. However, since edge servers are difficult to obtain security protections like in centralized data centers, this makes them more vulnerable to security attacks. To address this issue, we propose a novel digital twin system architecture in edge computing-based Internet of Things (IoT) networks, which allows efficient data processing for anomaly detection at the network edge. Digital twin is a replica of the real world in a virtual environment with continuous information exchange. Our digital twin-assisted solution gives a data-driven strategy against the cell outage and simulates the behavior and patterns of the physical assets in the digital world. Powered by data processing capabilities at the network edge, the proposed digital twin-assisted model can help extract features and behaviors, reduce noise from data generated in the network physical model, and filter out redundant data for a better characterization of the network physical model in the anomaly detection service. To this extent, the contributions in this paper are threefold: We first propose a novel digital twin-assisted anomaly detection framework that processes high-dimensional data efficiently and improves the system performance despite the imbalanced classes. Second, we define an autoencoder-based threshold algorithm and evaluate the eXtreme Gradient Boosting (XGBoost) classifier to implement self-learning capability into the digital twin for an accurate prediction. Third, we extract the features, dependencies, and relationships to apply the learned model to the new and unseen dataset for real-time monitoring and optimization and evaluate the performance of the proposed model with both real-time and historical data. Finally, we analyze the performance of different classifiers to validate our model. We use four different datasets and demonstrate that our solution outperforms the state-of-the-art techniques in terms of accuracy performance, AUC score, and ROC curve.
更多查看译文
关键词
Internet of Things,Anomaly detection,Digital twin,Edge computing,Self-organizing networks,Self-healing networks
AI 理解论文
溯源树
样例
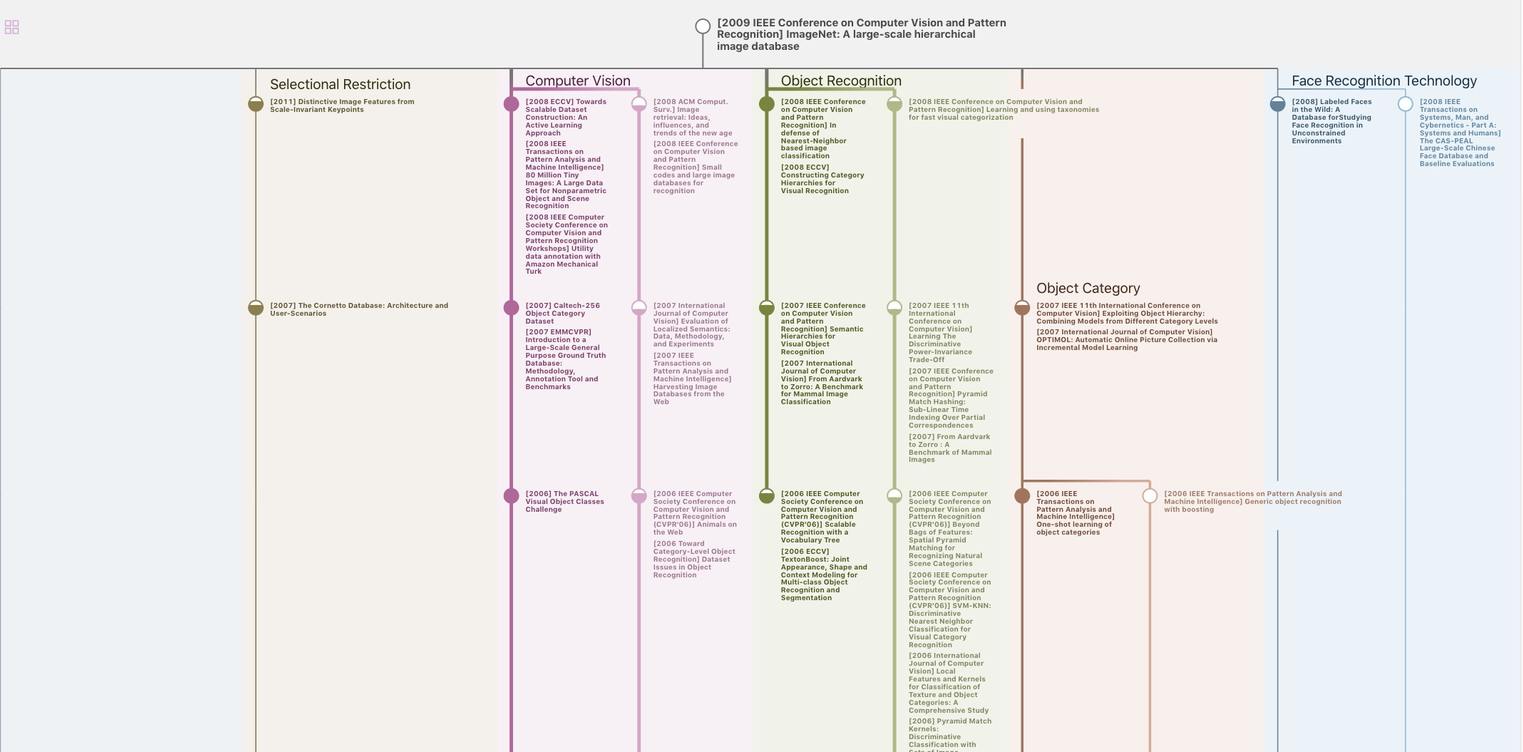
生成溯源树,研究论文发展脉络
Chat Paper
正在生成论文摘要