DASA: Delay-Adaptive Multi-Agent Stochastic Approximation
CoRR(2024)
摘要
We consider a setting in which N agents aim to speedup a common Stochastic
Approximation (SA) problem by acting in parallel and communicating with a
central server. We assume that the up-link transmissions to the server are
subject to asynchronous and potentially unbounded time-varying delays. To
mitigate the effect of delays and stragglers while reaping the benefits of
distributed computation, we propose , a Delay-Adaptive algorithm
for multi-agent Stochastic Approximation. We provide a finite-time analysis of
assuming that the agents' stochastic observation processes are
independent Markov chains. Significantly advancing existing results,
is the first algorithm whose convergence rate depends only on the
mixing time and on the average delay τ_avg while jointly
achieving an N-fold convergence speedup under Markovian sampling. Our work is
relevant for various SA applications, including multi-agent and distributed
temporal difference (TD) learning, Q-learning and stochastic optimization with
correlated data.
更多查看译文
AI 理解论文
溯源树
样例
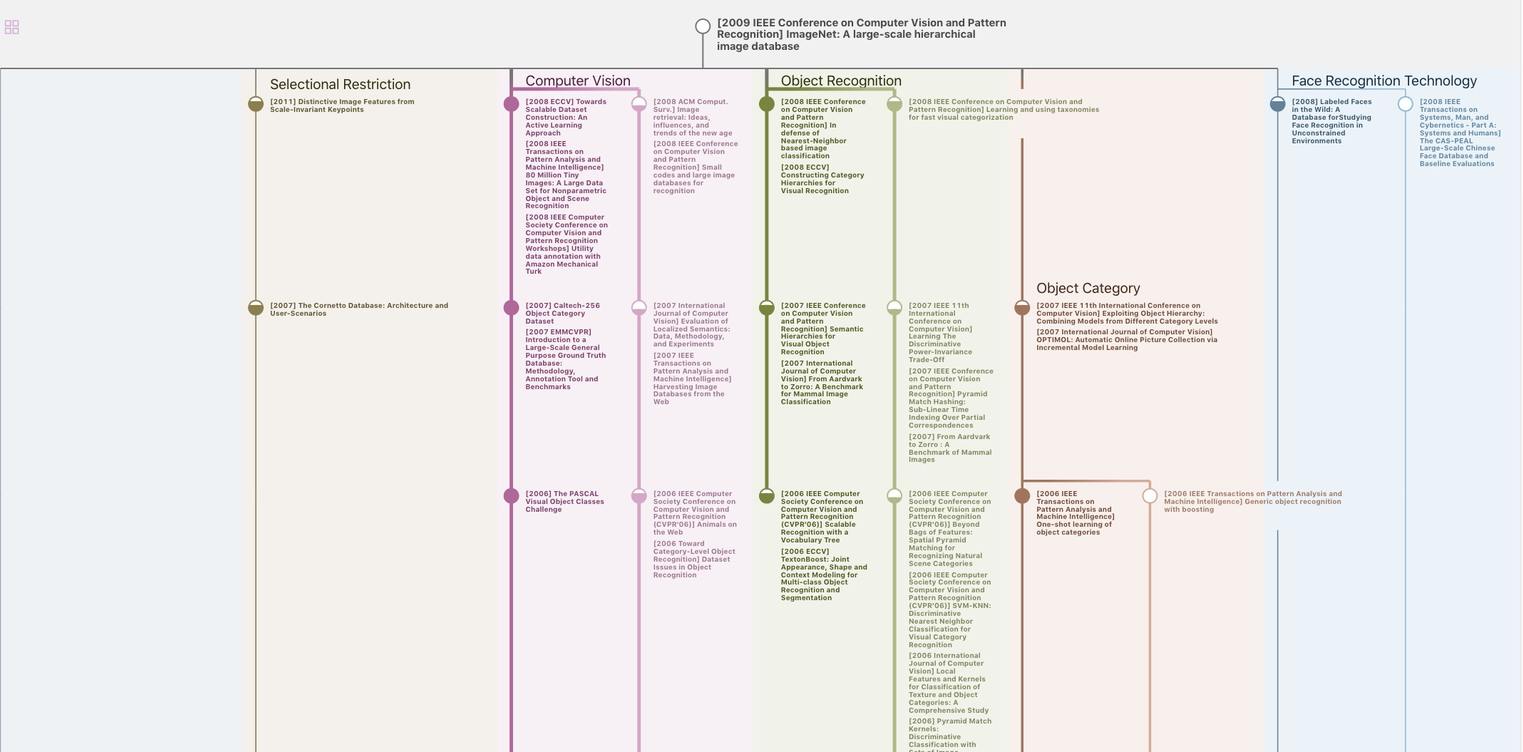
生成溯源树,研究论文发展脉络
Chat Paper
正在生成论文摘要