Zero-Shot Task Adaptation with Relevant Feature Information
AAAI 2024(2024)
摘要
We propose a method to learn prediction models such as classifiers for unseen target tasks where labeled and unlabeled data are absent but a few relevant input features for solving the tasks are given. Although machine learning requires data for training, data are often difficult to collect in practice. On the other hand, for many applications, a few relevant features would be more easily obtained. Although zero-shot learning or zero-shot domain adaptation use external knowledge to adapt to unseen classes or tasks without data, relevant features have not been used in existing studies. The proposed method improves the generalization performance on the target tasks, where there are no data but a few relevant features are given, by meta-learning from labeled data in related tasks. In the meta-learning phase, it is essential to simulate test phases on target tasks where prediction model learning is required without data. To this end, our neural network-based prediction model is meta-learned such that it correctly responds to perturbations of the relevant features on randomly generated synthetic data. By this modeling, the prediction model can explicitly learn the discriminability of the relevant features without real target data. When unlabeled training data are available in the target tasks, the proposed method can incorporate such data to boost the performance in a unified framework. Our experiments demonstrate that the proposed method outperforms various existing methods with four real-world datasets.
更多查看译文
关键词
ML: Transfer, Domain Adaptation, Multi-Task Learning,HAI: Human-in-the-loop Machine Learning,ML: Classification and Regression,ML: Semi-Supervised Learning
AI 理解论文
溯源树
样例
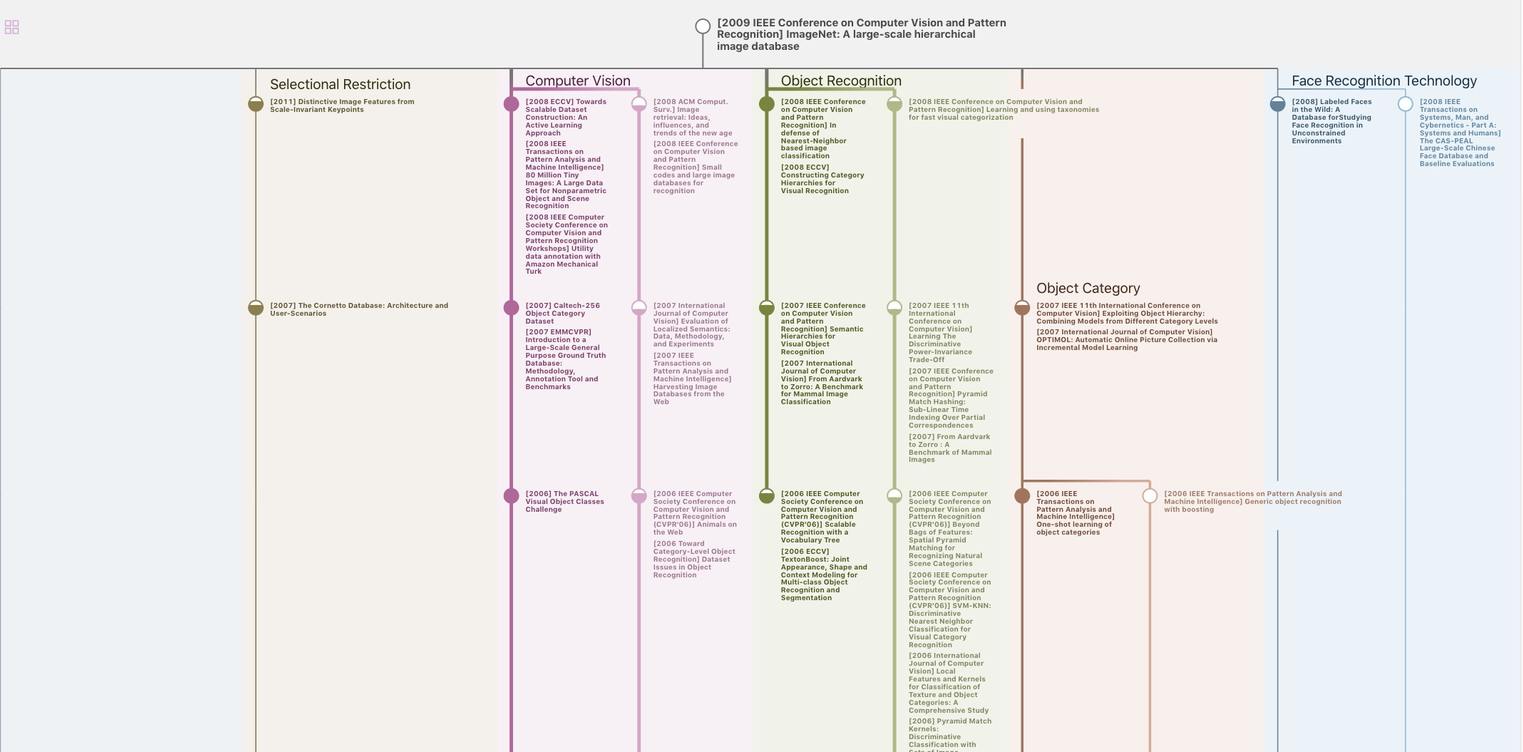
生成溯源树,研究论文发展脉络
Chat Paper
正在生成论文摘要