Influential Exemplar Replay for Incremental Learning in Recommender Systems
AAAI 2024(2024)
摘要
Personalized recommender systems have found widespread applications for effective information filtering. Conventional models engage in knowledge mining within the static setting to reconstruct singular historical data. Nonetheless, the dynamics of real-world environments are in a constant state of flux, rendering acquired model knowledge inadequate for accommodating emergent trends and thus leading to notable recommendation performance decline. Given the typically prohibitive cost of exhaustive model retraining, it has emerged to study incremental learning for recommender systems with ever-growing data. In this paper, we propose an effective model-agnostic framework, namely INFluential Exemplar Replay (INFER). INFER facilitates recommender models in retaining the earlier assimilated knowledge, e.g., users' enduring preferences, while concurrently accommodating evolving trends manifested in users' new interaction behaviors. We commence with a vanilla implementation that centers on identifying the most representative data samples for effective consolidation of early knowledge. Subsequently, we propose an advanced solution, namely INFERONCE, to optimize the computational overhead associated with the vanilla implementation. Extensive experiments on four prototypical backbone models, two classic recommendation tasks, and four widely used benchmarks consistently demonstrate the effectiveness of our method as well as its compatibility for extending to several incremental recommender models.
更多查看译文
关键词
DMKM: Applications,DMKM: Recommender Systems,DMKM: Web,ML: Applications
AI 理解论文
溯源树
样例
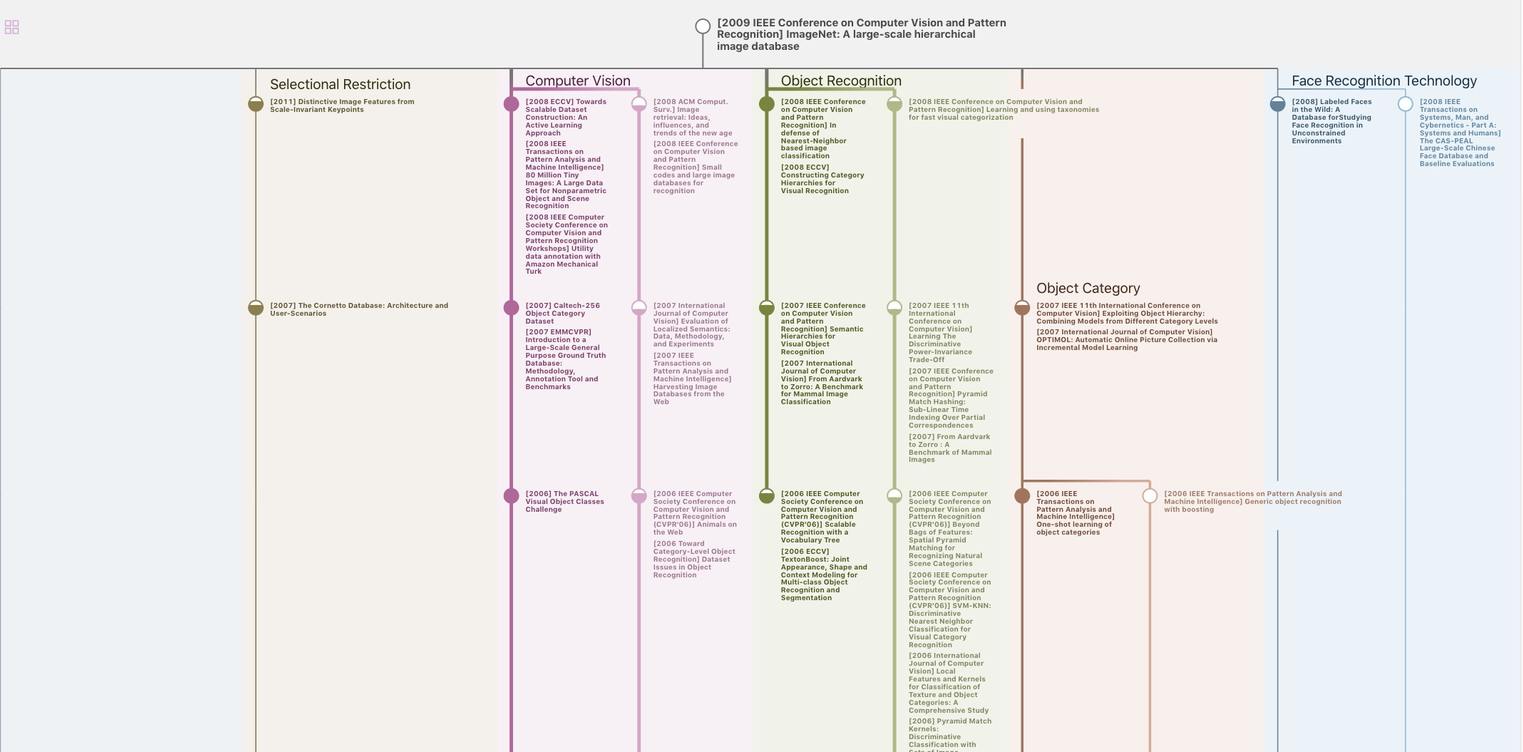
生成溯源树,研究论文发展脉络
Chat Paper
正在生成论文摘要