Geometry-Guided Domain Generalization for Monocular 3D Object Detection
AAAI 2024(2024)
摘要
Monocular 3D object detection (M3OD) is important for autonomous driving. However, existing deep learning-based methods easily suffer from performance degradation in real-world scenarios due to the substantial domain gap between training and testing. M3OD's domain gaps are complex, including camera intrinsic parameters, extrinsic parameters, image appearance, etc. Existing works primarily focus on the domain gaps of camera intrinsic parameters, ignoring other key factors. Moreover, at the feature level, conventional domain invariant learning methods generally cause the negative transfer issue, due to the ignorance of dependency between geometry tasks and domains. To tackle these issues, in this paper, we propose MonoGDG, a geometry-guided domain generalization framework for M3OD, which effectively addresses the domain gap at both camera and feature levels. Specifically, MonoGDG consists of two major components. One is geometry-based image reprojection, which mitigates the impact of camera discrepancy by unifying intrinsic parameters, randomizing camera orientations, and unifying the field of view range. The other is geometry-dependent feature disentanglement, which overcomes the negative transfer problems by incorporating domain-shared and domain-specific features. Additionally, we leverage a depth-disentangled domain discriminator and a domain-aware geometry regression attention mechanism to account for the geometry-domain dependency. Extensive experiments on multiple autonomous driving benchmarks demonstrate that our method achieves state-of-the-art performance in domain generalization for M3OD.
更多查看译文
关键词
CV: 3D Computer Vision,ML: Transfer, Domain Adaptation, Multi-Task Learning
AI 理解论文
溯源树
样例
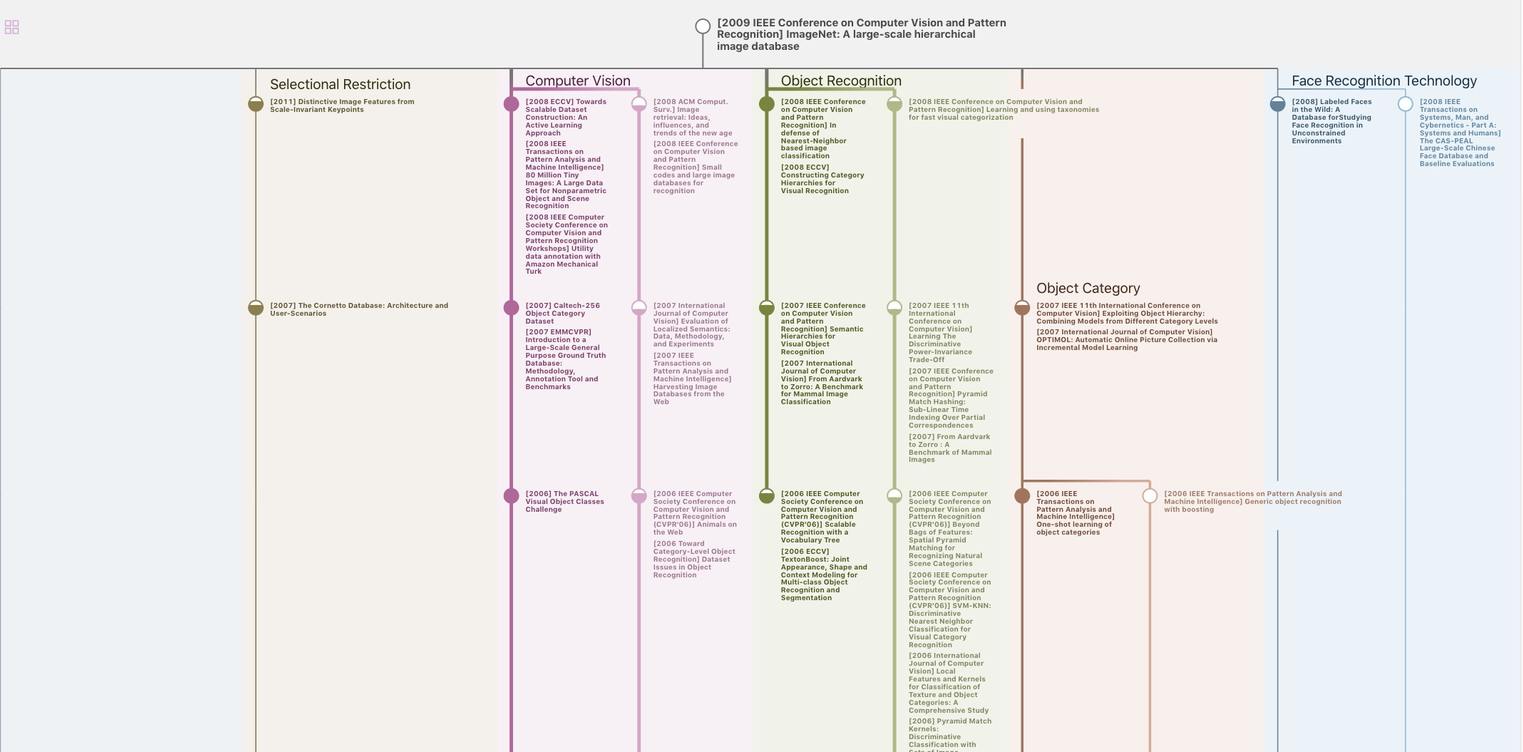
生成溯源树,研究论文发展脉络
Chat Paper
正在生成论文摘要