CutFreq: Cut-and-Swap Frequency Components for Low-Level Vision Augmentation
AAAI 2024(2024)
摘要
Low-level vision plays a crucial role in a wide range of imaging quality and image recognition applications. However, the limited size, quality, and diversity of datasets often pose significant challenges for low-level tasks. Data augmentation is the most effective and practical way of sample expansion, but the commonly used augmentation methods in high-level tasks have limited improvement in the low-level due to the boundary effects or the non-realistic context information. In this paper, we propose the Cut-and-Swap Frequency Components (CutFreq) method for low-level vision, which aims to preserve high-level representations with directionality and improve image synthesis quality. Observing the significant frequency domain differences between reconstructed images and real ones, in CutFreq, we propose to transform the input and real images separately in the frequency domain, then define two stages for the model training process, and finally swap the specified frequency bands respectively and inversely transform to generate augmented samples. The experimental results show the superior performance of CutFreq on five low-level vision tasks. Moreover, we demonstrate the effectiveness of CutFreq in the low-data regime. Code is available at https://github.com/DreamerCCC/CutFreq.
更多查看译文
关键词
CV: Low Level & Physics-based Vision,CV: Computational Photography, Image & Video Synthesis,CV: Representation Learning for Vision
AI 理解论文
溯源树
样例
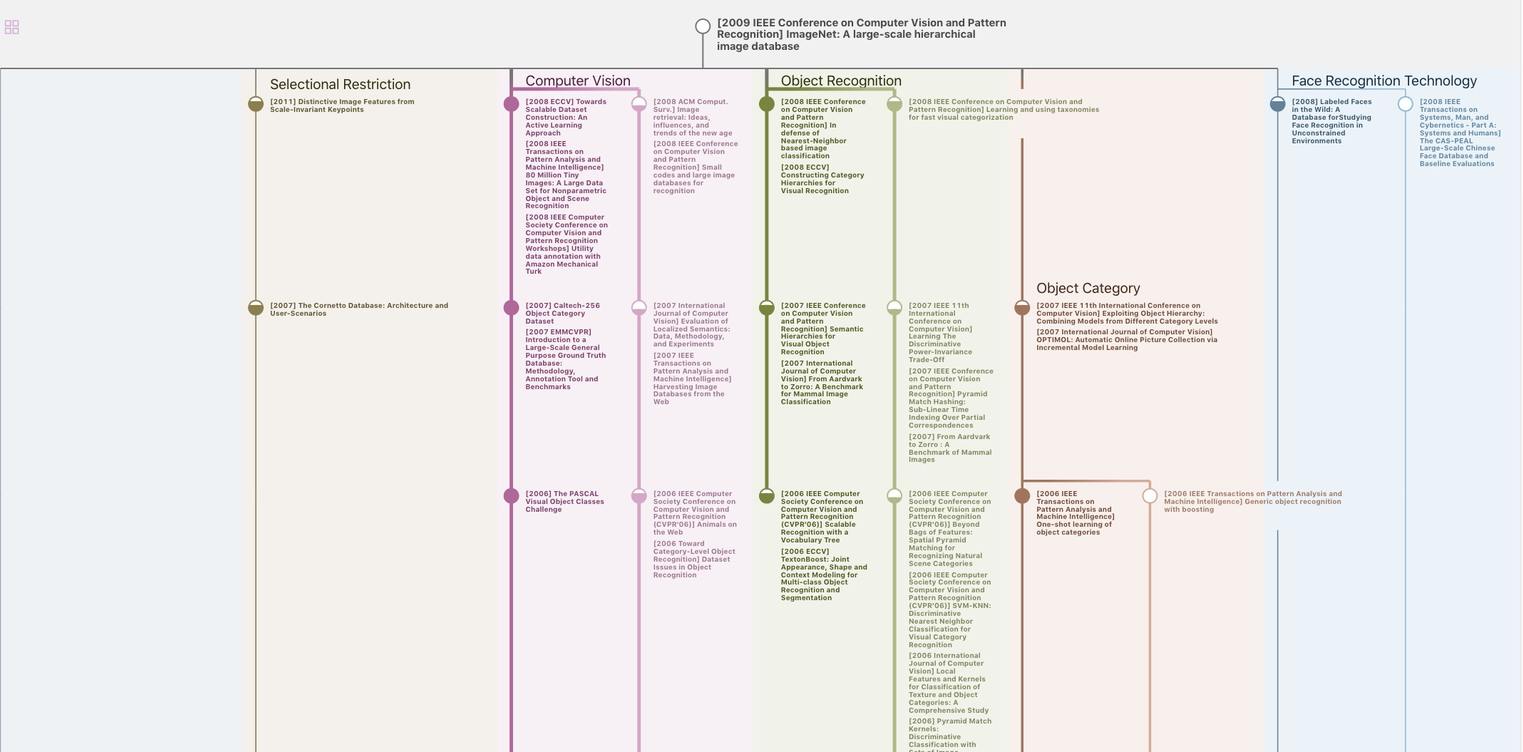
生成溯源树,研究论文发展脉络
Chat Paper
正在生成论文摘要