Multi-scale hash encoding based neural geometry representation
Computational Visual Media(2024)
摘要
Recently, neural implicit function-based representation has attracted more and more attention, and has been widely used to represent surfaces using differentiable neural networks. However, surface reconstruction from point clouds or multi-view images using existing neural geometry representations still suffer from slow computation and poor accuracy. To alleviate these issues, we propose a multi-scale hash encoding-based neural geometry representation which effectively and efficiently represents the surface as a signed distance field. Our novel neural network structure carefully combines low-frequency Fourier position encoding with multi-scale hash encoding. The initialization of the geometry network and geometry features of the rendering module are accordingly redesigned. Our experiments demonstrate that the proposed representation is at least 10 times faster for reconstructing point clouds with millions of points. It also significantly improves speed and accuracy of multi-view reconstruction. Our code and models are available at https://github.com/Dengzhi-USTC/Neural-Geometry-Reconstruction .
更多查看译文
关键词
neural geometry representation,hash encoding,point cloud reconstruction,multi-view reconstruction
AI 理解论文
溯源树
样例
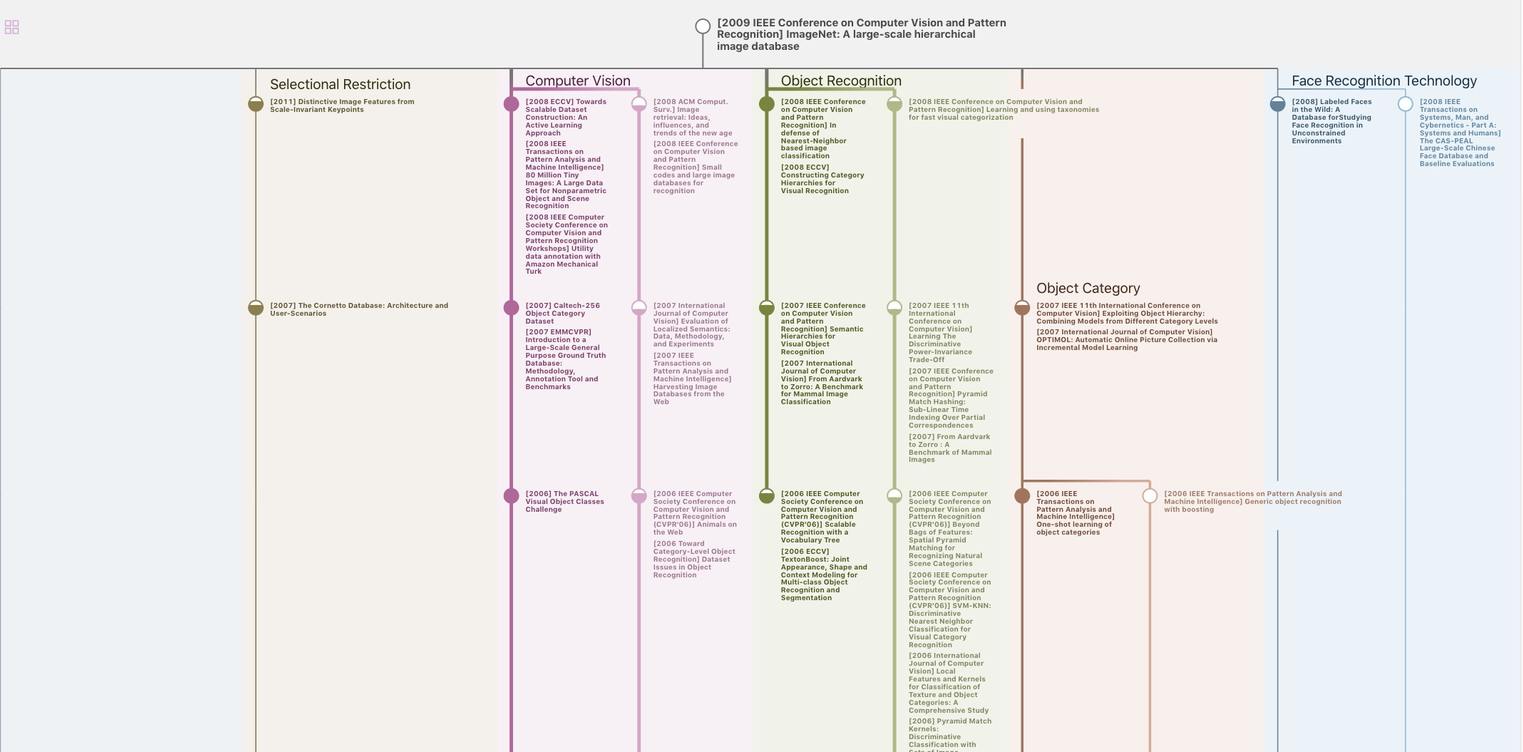
生成溯源树,研究论文发展脉络
Chat Paper
正在生成论文摘要