Representative and Back-In-Time Sampling from Real-world Hypergraphs
ACM Transactions on Knowledge Discovery from Data(2024)
摘要
Graphs are widely used for representing pairwise interactions in complex systems. Since such real-world graphs are large and often evergrowing, sampling subgraphs is useful for various purposes, including simulation, visualization, stream processing, representation learning, and crawling. However, many complex systems consist of group interactions (e.g., collaborations of researchers and discussions on online Q&A platforms) and thus are represented more naturally and accurately by hypergraphs than by ordinary graphs. Motivated by the prevalence of large-scale hypergraphs, we study the problem of sampling from real-world hypergraphs, aiming at answering (Q1) how can we measure the goodness of sub-hypergraphs, and (Q2) how can we efficiently find a “good” sub-hypergraph. Regarding Q1, we distinguish between two goals: (a) representative sampling , which aims at capturing the characteristics of the input hypergraph, and (b) back-in-time sampling , which aims at closely approximating a past snapshot of the input time-evolving hypergraph. To evaluate the similarity of the sampled sub-hypergraph to the target (i.e., the input hypergraph or its past snapshot), we consider 10 graph-level, hyperedge-level, and node-level statistics. Regarding Q2, we first conduct a thorough analysis of various intuitive approaches using 11 real-world hypergraphs. Then, based on this analysis, we propose MiDaS and MiDaS-B , designed for representative sampling and back-in-time sampling, respectively. Regarding representative sampling, we demonstrate through extensive experiments that MiDaS , which employs a sampling bias toward high-degree nodes in hyperedge selection, is (a) Representative : finding overall the most representative samples among 15 considered approaches, (b) Fast : several orders of magnitude faster than the strongest competitors, and (c) Automatic : automatically tuning the degree of sampling bias. Regarding back-in-time sampling, we demonstrate that MiDaS-B inherits the strengths of MiDaS despite an additional challenge—the unavailability of the target (i.e., past snapshot). It effectively handles this challenge by focusing on replicating universal evolutionary patterns, rather than directly replicating the target.
更多查看译文
关键词
Hypergraph,sampling,structural property,higher-order network
AI 理解论文
溯源树
样例
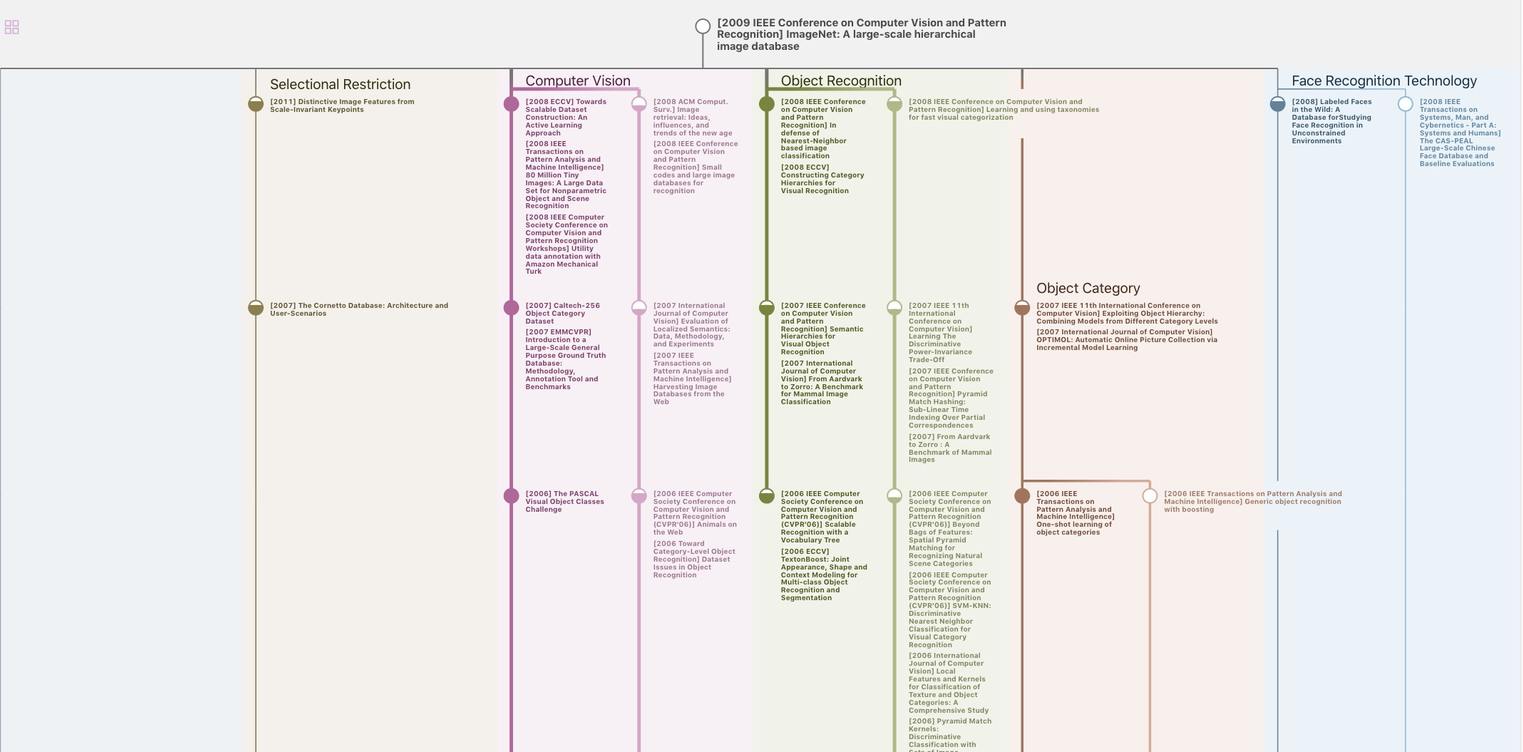
生成溯源树,研究论文发展脉络
Chat Paper
正在生成论文摘要