Energy Profiling of DNN Accelerators
2023 26TH EUROMICRO CONFERENCE ON DIGITAL SYSTEM DESIGN, DSD 2023(2023)
摘要
This paper introduces a novel methodology for assessing the energy efficiency of neural network accelerators at both layer and network granularity. The approach involves extracting per-layer timing reports from recorded power profiles. The power and energy consumption of three prominent neural network accelerators, namely the Intel Neural Compute Stick 2, the Coral Edge TPU, and the NXP i.MX8M Plus is evaluated for three different Deep Neural Networks (DNNs) using this method. The study investigates the relationship between decreasing sampling frequencies and the average error, as well as the detailed energy consumption of individual DNN layers and layer types. The findings reveal that latency outperforms the number of operations per layer as a predictor for both overall and dynamic energy, with errors of 10% and 100% respectively. The main conclusions are: a sampling frequency of 200 kHz is necessary to achieve an average error of 5%; the number of operations is an inadequate predictor of energy consumption; and specific hardware settings significantly influence power and energy consumption, emphasizing the need for their consideration in estimation.
更多查看译文
关键词
Power analysis,Deep Neural Networks,Hardware accelerators
AI 理解论文
溯源树
样例
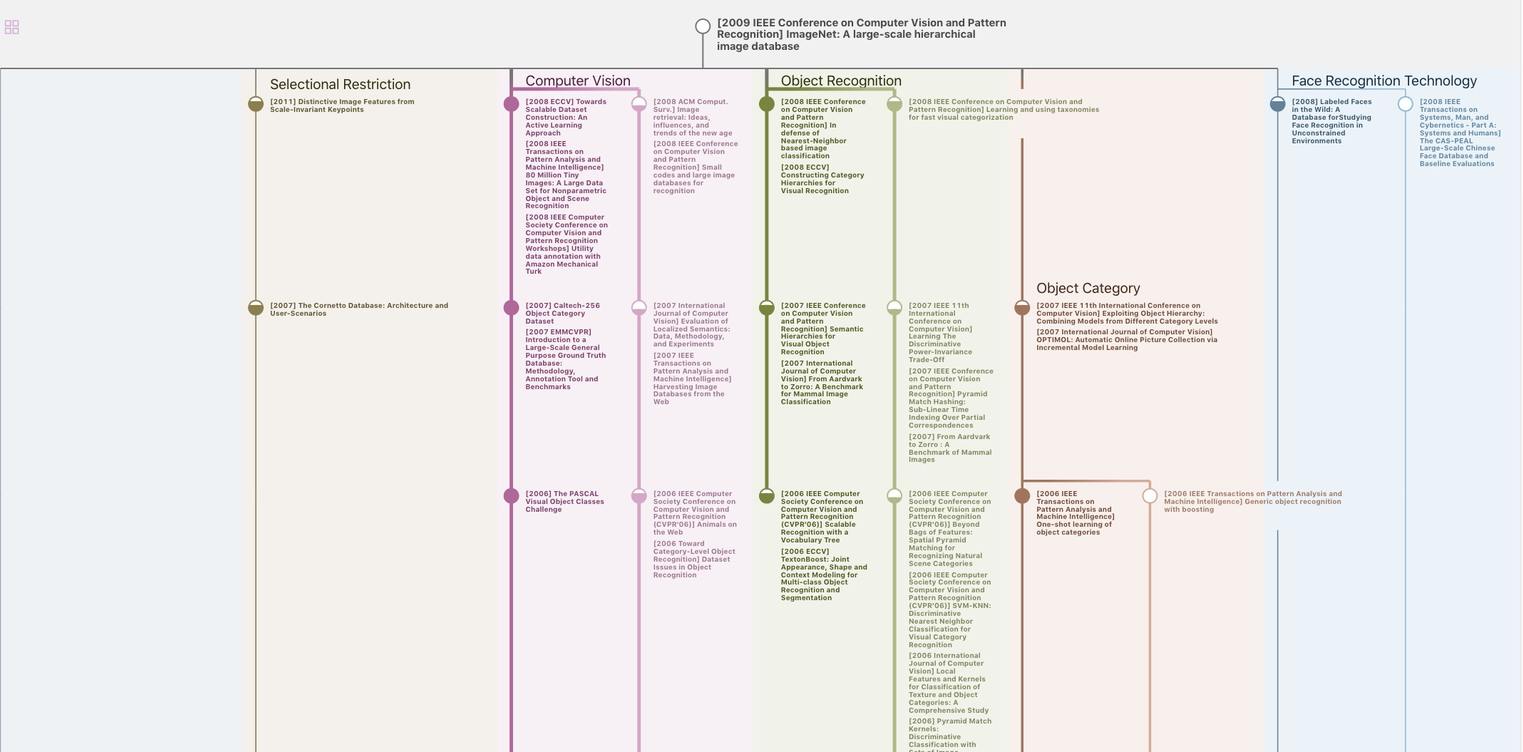
生成溯源树,研究论文发展脉络
Chat Paper
正在生成论文摘要