Multi-Modal Machine Learning for Navigating Noisy Objectives of Automotive Manufacturing Quality Inspection.
International Conference on Machine Learning and Applications(2023)
摘要
A significant portion of Machine Learning (ML) studies are toward automation of the training process for finding a robust ML model, while requiring the least amount of human effort. Although the introduced Automated ML (AutoML) tools show promising results with ongoing efforts for faster search, the primary beneficiaries are data scientists. Meanwhile, one of the most valuable data scientist roles is their experience in converting unclear, and noisy empirical problems into ML problems. In other words, the tool's performance is still relative to data scientists' consultation in crafting (business) requirements into ML pipelines. Such an entanglement to experts can avoid full automation of ML use for domain specialists with no background in programming and algorithms. This makes domain users, such as business owners, still dependent on the support of ML practitioners in every new use case. In this paper, we conduct a study to evaluate the mediator feature in which multi-modality simulates interchanging information from business users to data scientists in actual exchange meetings. We consider an industrial scenario, where noisy quality inspection business objectives and data scientists' past actions are used as a foundation for choosing follow-up ML tasks for future applications under known business requirements.
更多查看译文
关键词
Multi modal,Industrial ML
AI 理解论文
溯源树
样例
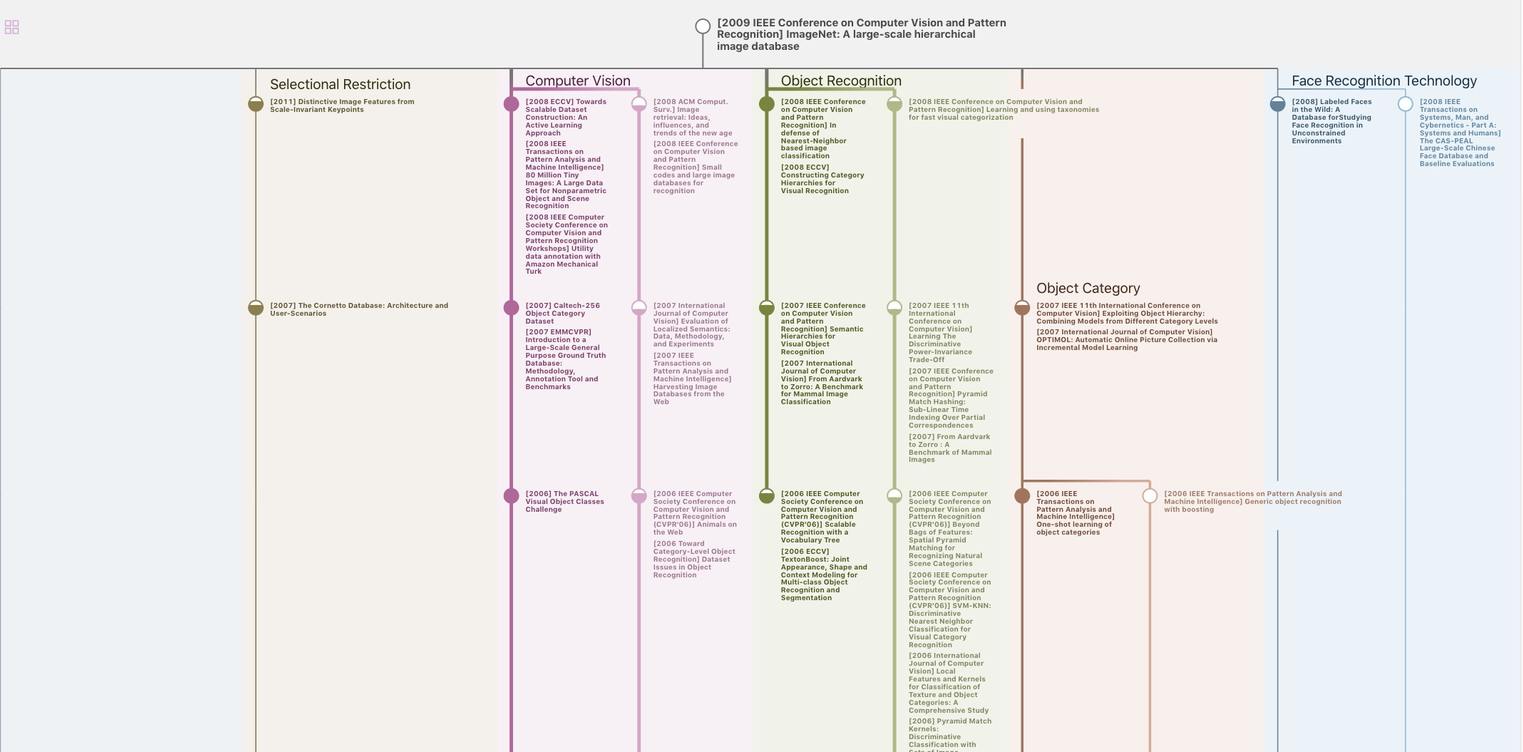
生成溯源树,研究论文发展脉络
Chat Paper
正在生成论文摘要