Improving Open-Set Recognition with Bayesian Metric Learning
ICASSP 2024 - 2024 IEEE International Conference on Acoustics, Speech and Signal Processing (ICASSP)(2024)
摘要
Conventionally, it is often assumed that the training and testing data distributions are the same and that all classes in the test set are observed in the training set. However, this assumption may not be true in real-world tasks. In practice, there may be test samples from classes that were unknown during training. In such cases, one would like the adopted model to have the capacity to classify such samples into a "none of the above" class. This task is known as open-set recognition, and it has gained significant attention in recent years. In this paper, we propose a novel distance-based open-set recognition approach by constructing task-specific distance metrics with Gaussian processes. Our experimental results demonstrate that the proposed approach outperforms state-of-the-art methods on both synthetic and real-world datasets.
更多查看译文
关键词
open-set recognition,distance metric learning,multiclass classification,Gaussian processes,novelty detection
AI 理解论文
溯源树
样例
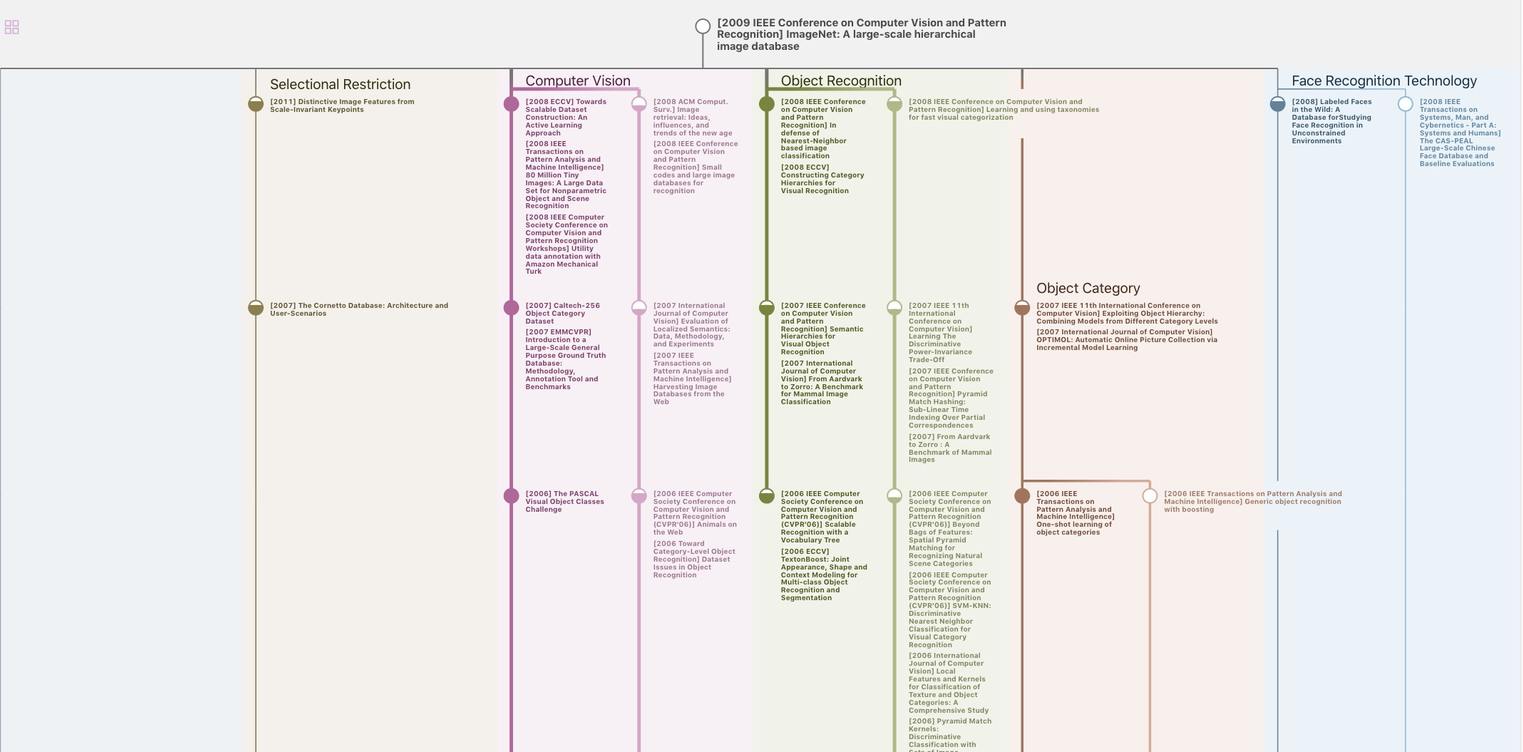
生成溯源树,研究论文发展脉络
Chat Paper
正在生成论文摘要