Unified Cross-Modal Attention: Robust Audio-Visual Speech Recognition and Beyond
IEEE-ACM TRANSACTIONS ON AUDIO SPEECH AND LANGUAGE PROCESSING(2024)
摘要
Audio-Visual Speech Recognition (AVSR) is a promising approach to improving the accuracy and robustness of speech recognition systems with the assistance of visual cues in challenging acoustic environments. In this paper, we present a novel audio-visual speech recognition architecture with unified cross-modal attention. Our approach concatenates the sequences temporally from different modalities and encodes the fused sequence in the unified feature space using a shared Conformer encoder. We then explicitly model additive noise and potential out-of-sync samples during training, and propose an auxiliary asynchronization-aware loss to improve the system performance on out-of-sync data. To enhance the efficacy of unified cross-modal attention, a manual attention alignment strategy is designed and applied to the model, bringing additional gains in both recognition accuracy and computation cost. As demonstrated by experiments on the large-scale audio-visual LRS3 dataset, our proposed approach reduces the word error rate (WER) by relatively 50% compared to the audio-only single-modal ASR system under noisy conditions, and relatively 25% compared to the previous audio-visual ASR baseline. The proposed audio-visual ASR system also shows superior robustness in more challenging conditions, such as audio-only data, visual corruption, audio-visual misalignment, and multi-talker interference. Moreover, the proposed Unified Cross-Modal Attention model exhibits a more general ability in multi-modality fusion, allowing for easy integration of additional modalities into the model with this framework to achieve a more accurate, robust, and safer multi-modal system.
更多查看译文
关键词
Audio-visual speech recognition,modality corruption,noise robustness,unified cross-modal attention
AI 理解论文
溯源树
样例
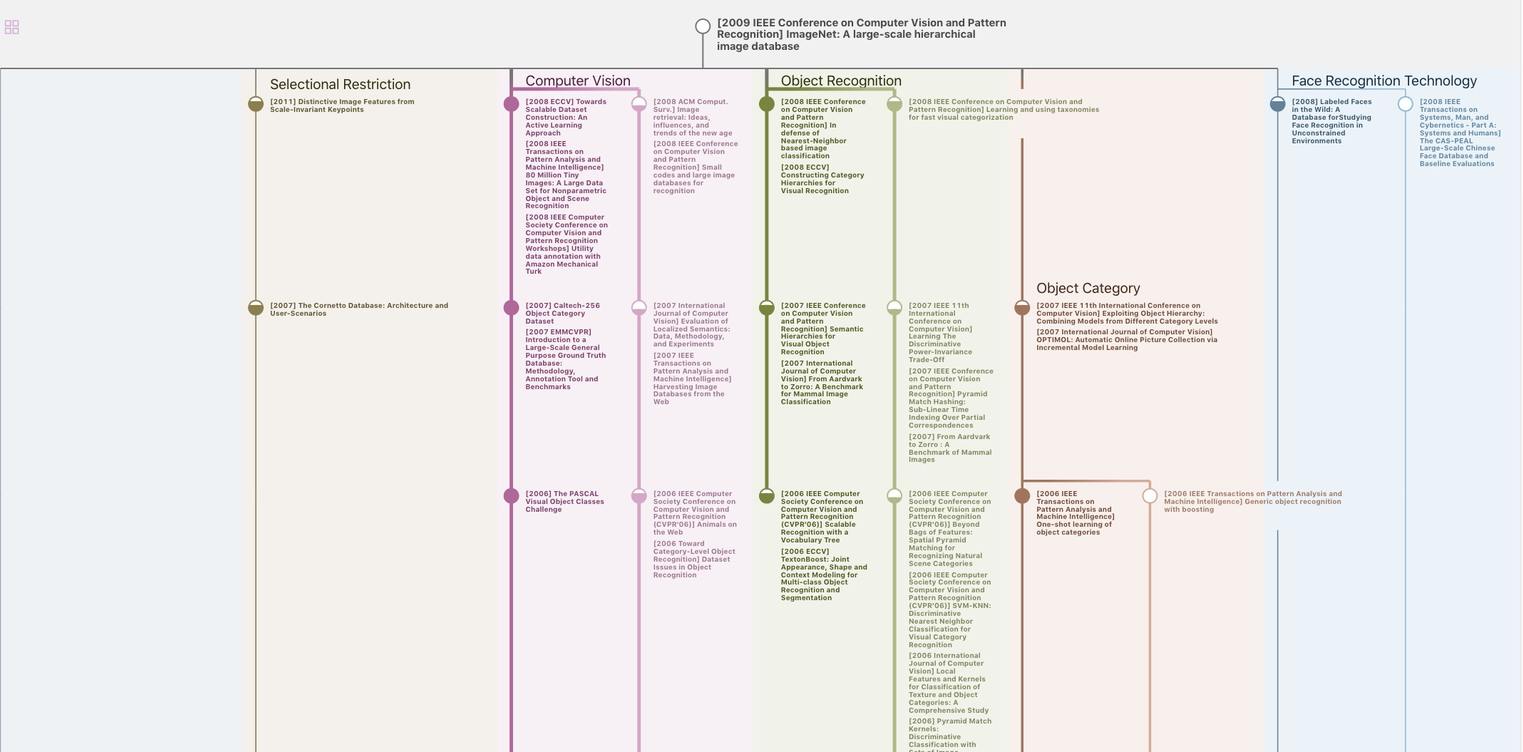
生成溯源树,研究论文发展脉络
Chat Paper
正在生成论文摘要