Efficient Architecture Search for Real-Time Instance Segmentation
ICASSP 2024 - 2024 IEEE International Conference on Acoustics, Speech and Signal Processing (ICASSP)(2024)
摘要
Traditional CNN-based training for instance segmentation is time-consuming owing to large datasets and complex network modules, making direct searching of architecture challenging. In this paper, we introduce an efficient framework, named EASInst. It can discover practical backbone and encoder architectures for the improved sparse activation instance segmentation model. Specifically, we construct a supernet for both backbone and encoder modules of SparseInst based on a differentiable method. In addition, kernel sharing mask and channel pruning technology are employed. Moreover, Taylor-Loss and a novel DY-Loss are devised for instance segmentation to improve the accuracy. Experiments show that the searched architectures outperform the existing Resnet-based real-time instance segmentation methods, which achieve 38.5 mAP with 39.5 FPS on COCO test-dev set.
更多查看译文
关键词
instance segmentation,neural architecture search
AI 理解论文
溯源树
样例
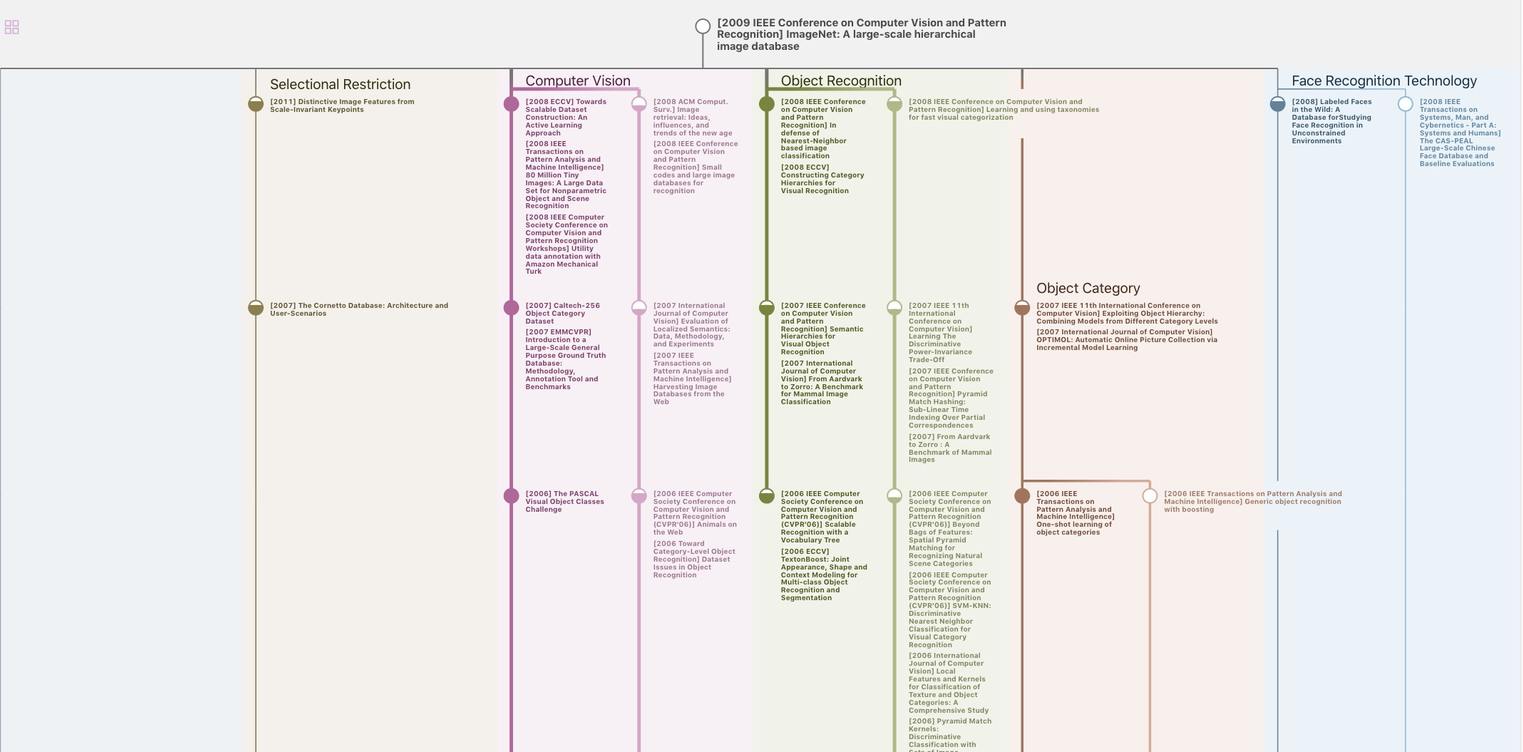
生成溯源树,研究论文发展脉络
Chat Paper
正在生成论文摘要