ProAug: Prototype-Based Augmentation for Long-Tailed Image Classification
ICASSP 2024 - 2024 IEEE International Conference on Acoustics, Speech and Signal Processing (ICASSP)(2024)
摘要
Real-world data often exhibit long-tailed distributions with heavy class imbalance, which deteriorates the generalization performance of the classifier. To mitigate this problem, we propose a novel Prototype-based Augmentation framework (ProAug) to address the data scarcity issue by augmenting the feature space for tail classes. Our ProAug consists of a prototype construction branch and a dynamic augmentation branch. The prototype-based dictionary is optimized with category-aware margin loss to learn multi-center and discriminative prototypes for each category. In the dynamic augmentation branch, we aim to produce high-quality tail-class features by dynamically composing context-similar prototypes with an attention mechanism. Moreover, to further improve the reliability of prototypes and the quality of augmented features, a meta-update strategy is adopted to calibrate two branches of ProAug to boost performance. Extensive empirical results on CIFAR-LT-10/100, ImageNet-LT, and iNaturalist 2018 demonstrate the effectiveness of our method.
更多查看译文
关键词
Long-tail classification,margin loss,prototype,meta-update strategy,deep learning
AI 理解论文
溯源树
样例
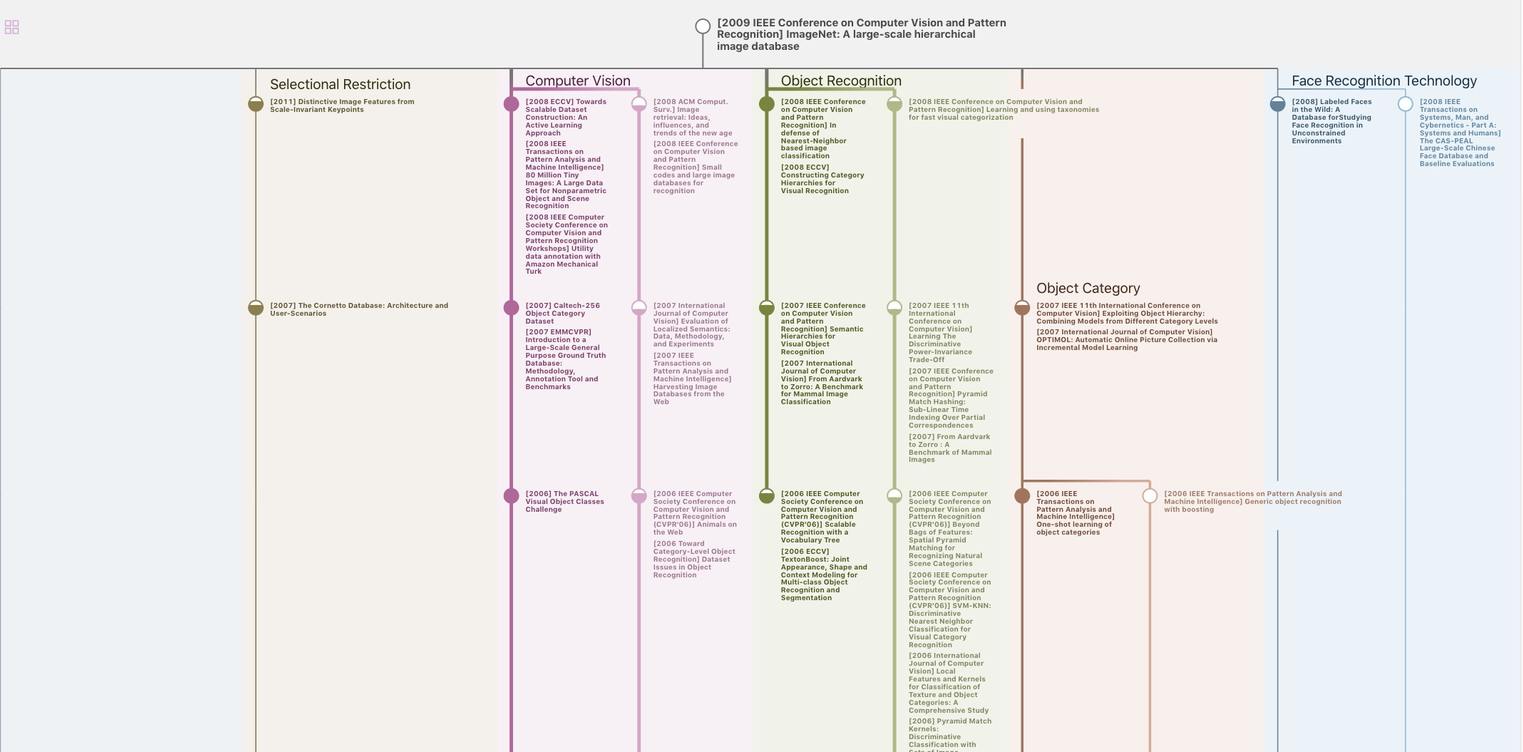
生成溯源树,研究论文发展脉络
Chat Paper
正在生成论文摘要