Boosting Speech Enhancement with Clean Self-Supervised Features Via Conditional Variational Autoencoders
ICASSP 2024 - 2024 IEEE International Conference on Acoustics, Speech and Signal Processing (ICASSP)(2024)
摘要
Recently, Self-Supervised Features (SSF) trained on extensive speech datasets have shown significant performance gains across various speech processing tasks. Nevertheless, their effectiveness in Speech Enhancement (SE) systems is often suboptimal due to insufficient optimization for noisy environments. To address this issue, we present a novel methodology that directly utilizes SSFs extracted from clean speech for enhancing SE models. Specifically, we leverage the clean SSFs for latent space modeling within the Conditional Variational Autoencoder (CVAE) framework. Consequently, we enable our model to fully leverage the knowledge existing in the clean SSFs without the interference of noise. In experiments, our approach yields clear improvements over existing methods that use SSFs across six evaluation metrics. Furthermore, we provide comprehensive analyses to validate the effectiveness of 1) incorporating clean SSFs within the CVAE framework and 2) the training techniques used to achieve optimal performance from our approach in SE systems. Code and audio samples are available at https://github.com/YoonhyungLee94/SSFCVAE
更多查看译文
关键词
Speech enhancement,self-supervised features,conditional variational autoencoder,posterior collapse
AI 理解论文
溯源树
样例
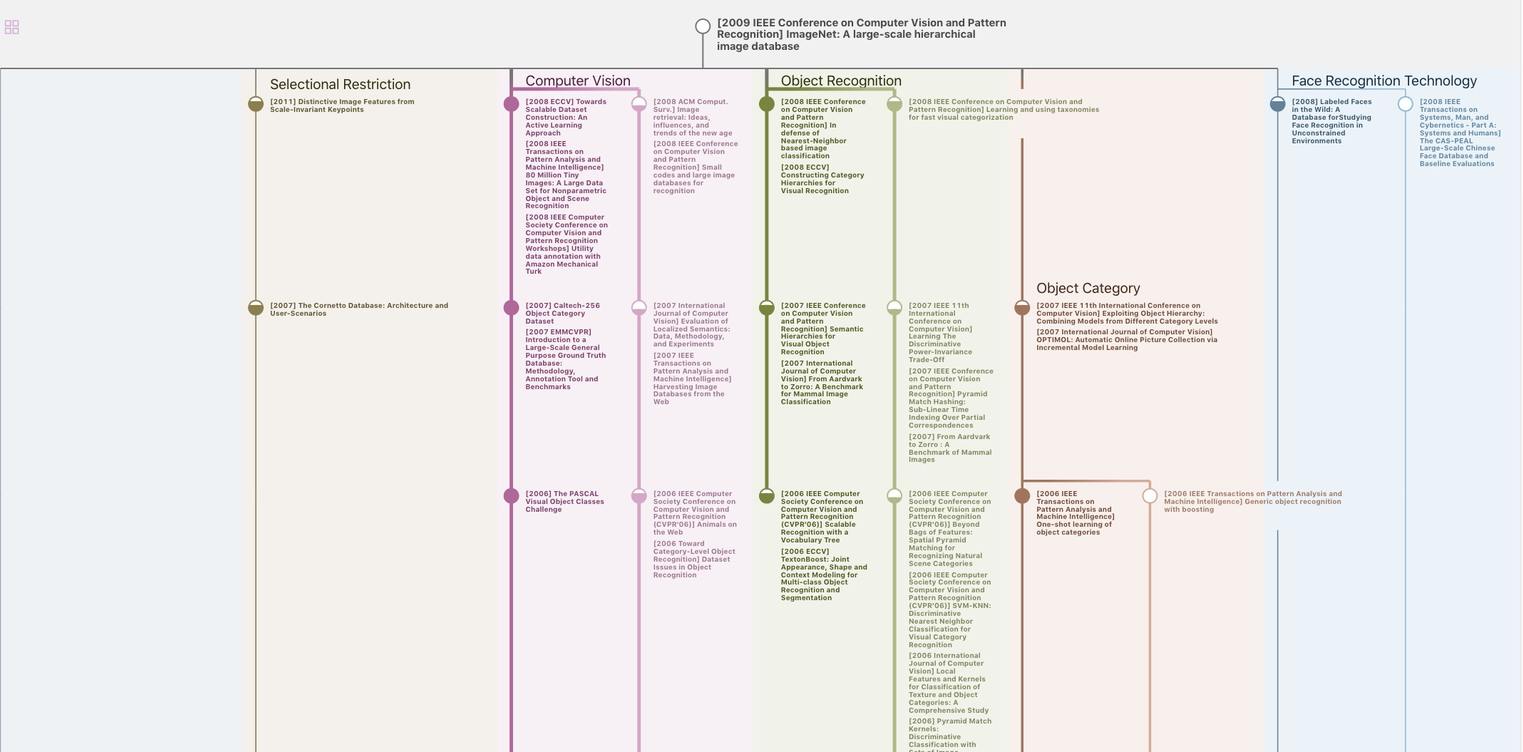
生成溯源树,研究论文发展脉络
Chat Paper
正在生成论文摘要