Multi-modal Data and Model Reduction for Enabling Edge Fusion in Connected Vehicle Environments
IEEE Transactions on Vehicular Technology(2024)
摘要
The emergence of edge computing in Intelligent Transportation Systems (ITS) has shown promise in enabling real-time sensor fusion applications. In this paper, we explore how to utilize edge computing to aid in collaborative vehicular perception, an emerging topic within ITS which involves vehicles sharing sensor data with one another to extend each vehicle's perception beyond what its individual sensors can see. However, achieving real time collaborative perception is a challenge even with the utilization of edge computing; due to the large amount of multi-modal sensor data produced by modern intelligent vehicles, the amount of data transmitted over wireless channels and the complexity of computational tasks will need to be managed dynamically based on the wireless network conditions and available computing resources. As such we propose REFO, a Real-time Edge Fusion Optimization method that combines task partitioning with data reduction and model compression to maximize sensor fusion accuracy while adhering to Quality of Service (QoS) requirements. We define a performance metric termed effective mean average precision (EmAP), which incorporates both QoS and fusion accuracy, and show that our proposed neural network based REFO action decision framework can outperform the best comparison models by approximately 10% in terms of EmAP over 18 test combinations of network and computing conditions.
更多查看译文
关键词
Connected Vehicles,Data Reduction,Model Compression,Task Partitioning,Machine Learning
AI 理解论文
溯源树
样例
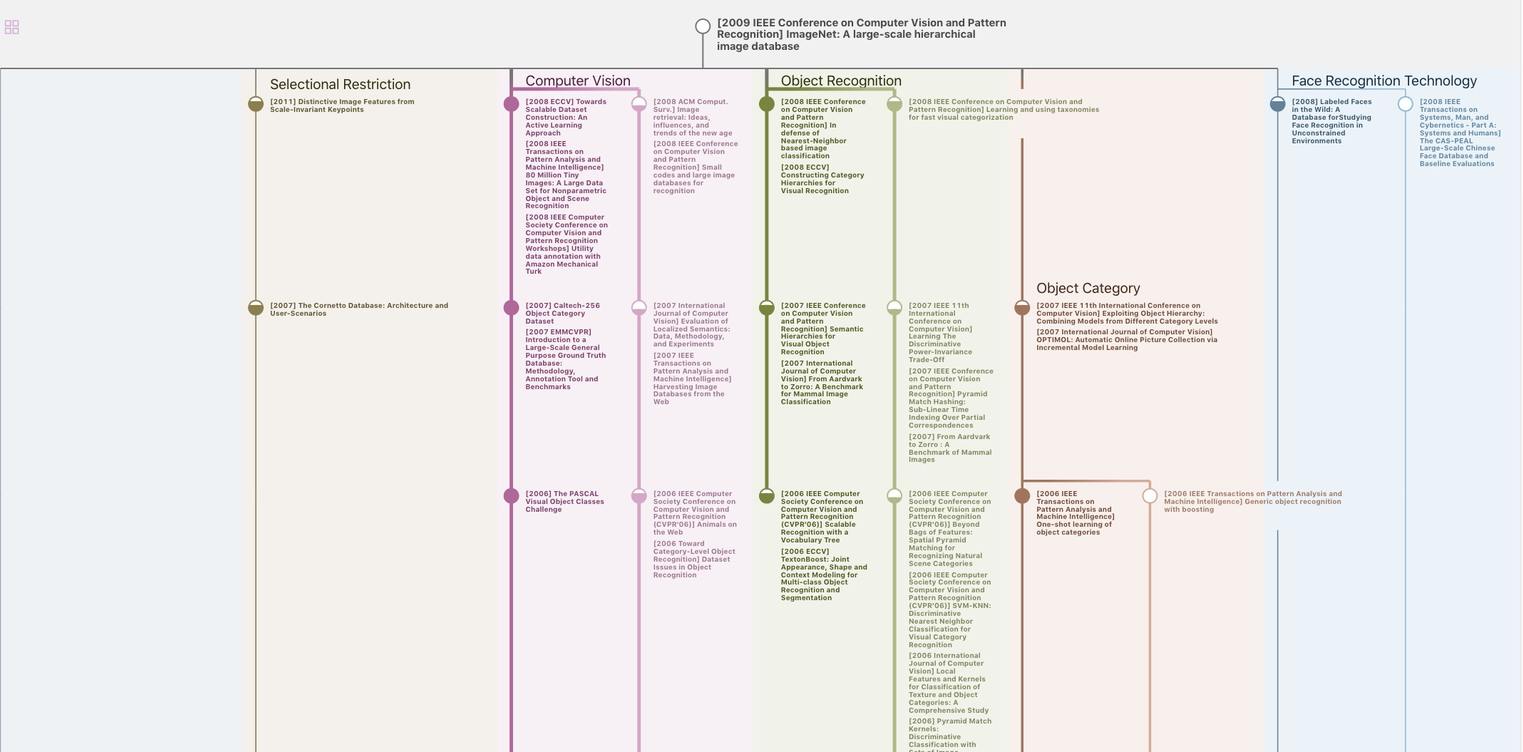
生成溯源树,研究论文发展脉络
Chat Paper
正在生成论文摘要