Improving Chinese Fact Checking via Prompt Based Learning and Evidence Retrieval
PROCEEDINGS OF THE 2023 IEEE/ACM INTERNATIONAL CONFERENCE ON ADVANCES IN SOCIAL NETWORKS ANALYSIS AND MINING, ASONAM 2023(2023)
摘要
Verifying the accuracy of information is a constant task as the prevalence of misinformation on the Web. In this paper, we focus on Chinese fact-checking (CHEF dataset) [1] and improve the performance through prompt-based learning in both evidence retrieval and claim verification. We adopted the Automated Prompt Engineering (APE) technique to generate the template and compared various prompt-based learning training strategies, such as prompt tuning and low-rank adaptation (LoRA) for claim verification. The research results show that prompt-based learning can improve the macro-F1 performance of claim verification by 2%-3% (from 77.62 to 80.29) using golden evidences and 110M BERT based model. For evidence retrieval, we use both the supervised SentenceBERT [2] and unsupervised PromptBERT [3] models to improve evidence retrieval performance. Experimental results show that the micro-F1 performance of evidence retrieval is significantly improved from 11.86% to 30.61% and 88.15% by PromptBERT and SentenceBERT, respectively. Finally, the overall fact-checking performance, i.e. the macro-F1 performance of claim verification, can be significantly improved from 61.94% to 80.16% when the semantic ranking-based evidence retrieval is replaced by SentenceBERT.
更多查看译文
关键词
fact checking,claim verification,supervised evidence retrieval,prompt based learning,sentenceBERT
AI 理解论文
溯源树
样例
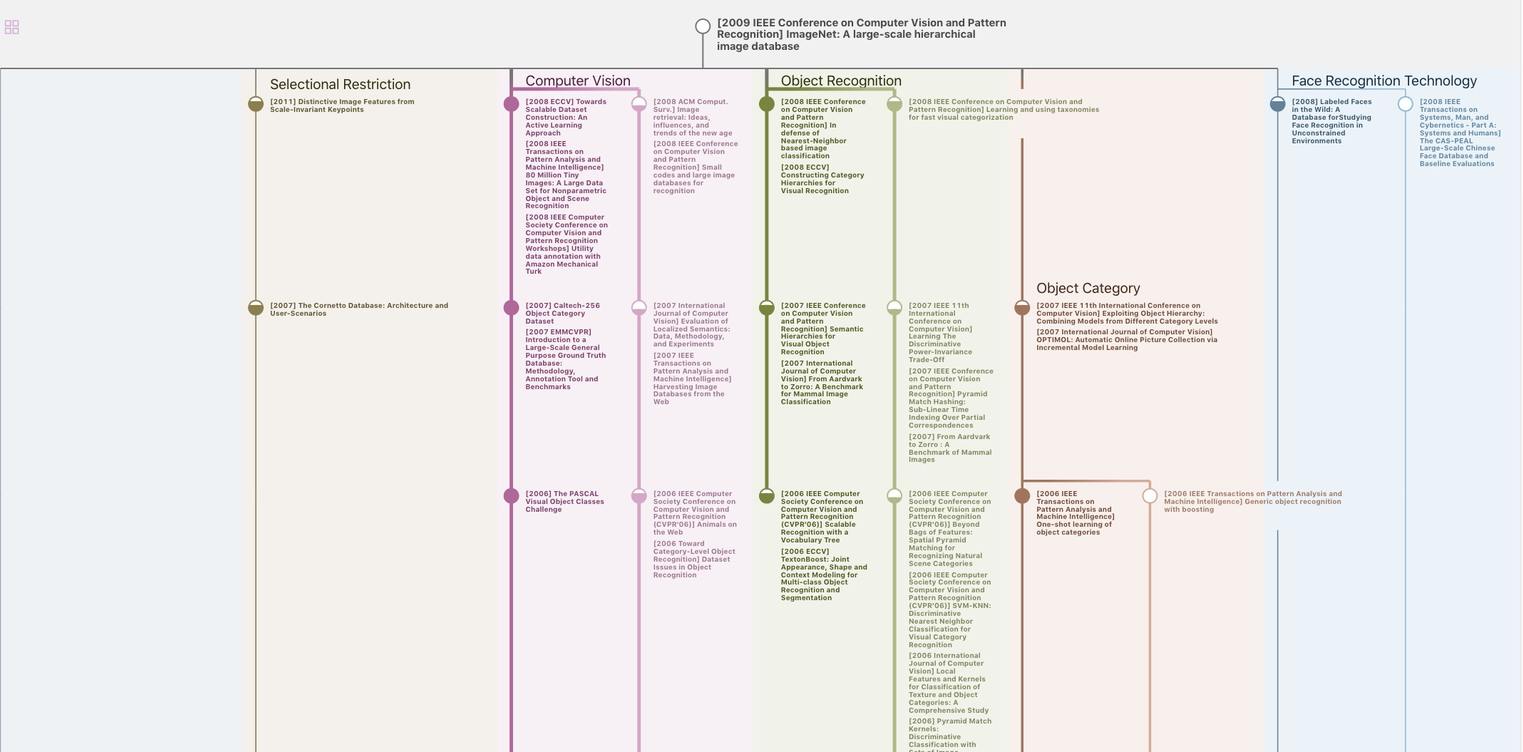
生成溯源树,研究论文发展脉络
Chat Paper
正在生成论文摘要