Near-Optimal Solutions of Constrained Learning Problems
ICLR 2024(2024)
摘要
With the widespread adoption of machine learning systems, the need to curtail
their behavior has become increasingly apparent. This is evidenced by recent
advancements towards developing models that satisfy robustness, safety, and
fairness requirements. These requirements can be imposed (with generalization
guarantees) by formulating constrained learning problems that can then be
tackled by dual ascent algorithms. Yet, though these algorithms converge in
objective value, even in non-convex settings, they cannot guarantee that their
outcome is feasible. Doing so requires randomizing over all iterates, which is
impractical in virtually any modern applications. Still, final iterates have
been observed to perform well in practice. In this work, we address this gap
between theory and practice by characterizing the constraint violation of
Lagrangian minimizers associated with optimal dual variables, despite lack of
convexity. To do this, we leverage the fact that non-convex, finite-dimensional
constrained learning problems can be seen as parametrizations of convex,
functional problems. Our results show that rich parametrizations effectively
mitigate the issue of feasibility in dual methods, shedding light on prior
empirical successes of dual learning. We illustrate our findings in fair
learning tasks.
更多查看译文
关键词
Constrained Learning,Convex Optimization,Duality,Constrained Optimization,Fairness
AI 理解论文
溯源树
样例
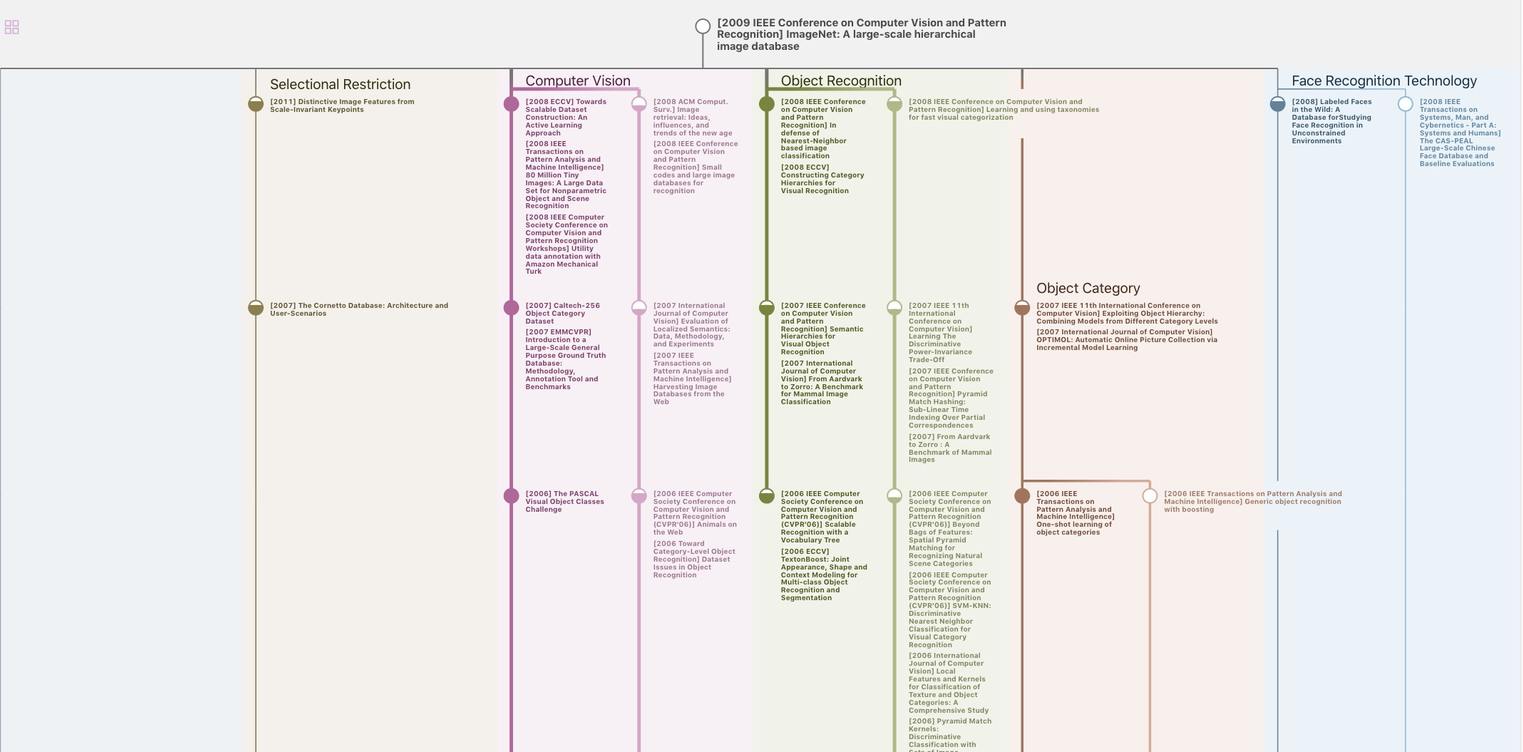
生成溯源树,研究论文发展脉络
Chat Paper
正在生成论文摘要