SPEAR:Exact Gradient Inversion of Batches in Federated Learning
arxiv(2024)
摘要
Federated learning is a popular framework for collaborative machine learning
where multiple clients only share gradient updates on their local data with the
server and not the actual data. Unfortunately, it was recently shown that
gradient inversion attacks can reconstruct this data from these shared
gradients. Existing attacks enable exact reconstruction only for a batch size
of b=1 in the important honest-but-curious setting, with larger batches
permitting only approximate reconstruction. In this work, we propose the
first algorithm reconstructing whole batches with b >1 exactly. This
approach combines mathematical insights into the explicit low-rank structure of
gradients with a sampling-based algorithm. Crucially, we leverage ReLU-induced
gradient sparsity to precisely filter out large numbers of incorrect samples,
making a final reconstruction step tractable. We provide an efficient GPU
implementation for fully connected networks and show that it recovers batches
of b ≲ 25 elements exactly while being tractable for large network
widths and depths.
更多查看译文
AI 理解论文
溯源树
样例
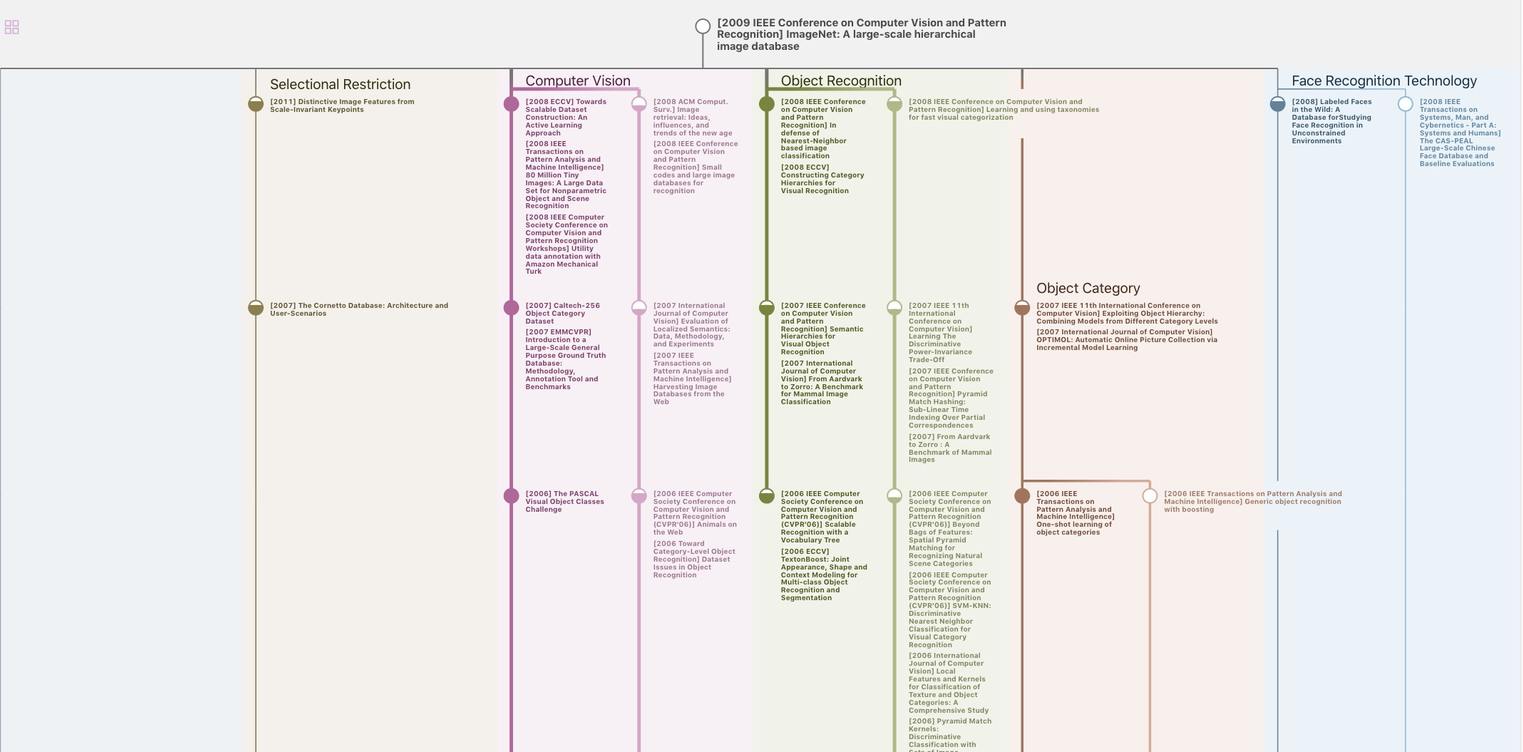
生成溯源树,研究论文发展脉络
Chat Paper
正在生成论文摘要