CookieGraph: Understanding and Detecting First-Party Tracking Cookies
PROCEEDINGS OF THE 2023 ACM SIGSAC CONFERENCE ON COMPUTER AND COMMUNICATIONS SECURITY, CCS 2023(2023)
摘要
As third-party cookie blocking is becoming the norm in mainstream web browsers, advertisers and trackers have started to use first-party cookies for tracking. To understand this phenomenon, we conduct a differential measurement study with versus without third-party cookies. We find that first-party cookies are used to store and exfiltrate identifiers to known trackers even when third-party cookies are blocked. As opposed to third-party cookie blocking, first-party cookie blocking is not practical because it would result in major breakage of website functionality. We propose CookieGraph, a machine learning-based approach that can accurately and robustly detect and block first-party tracking cookies. CookieGraph detects first-party tracking cookies with 90.18% accuracy, outperforming the stateof-the-art CookieBlock by 17.31%. We show that CookieGraph is robust against cookie name manipulation, while CookieBlock's accuracy drops by 15.87%. While blocking all first-party cookies results in major breakage on 32% of the sites with SSO logins, and CookieBlock reduces it to 10%, we show that CookieGraph does not cause any major breakage on these sites. Our deployment of CookieGraph shows that first-party tracking cookies are used on 89.86% of the top-million websites. We find that 96.61% of these first-party tracking cookies are in fact ghostwritten by third-party scripts embedded in the first-party context. We also dind evidence of first-party tracking cookies being set by fingerprinting scripts. The most prevalent first-party tracking cookies are set by major advertising entities such as Google, Facebook, and TikTok.
更多查看译文
关键词
cookies,machine learning,privacy,tracking,web security
AI 理解论文
溯源树
样例
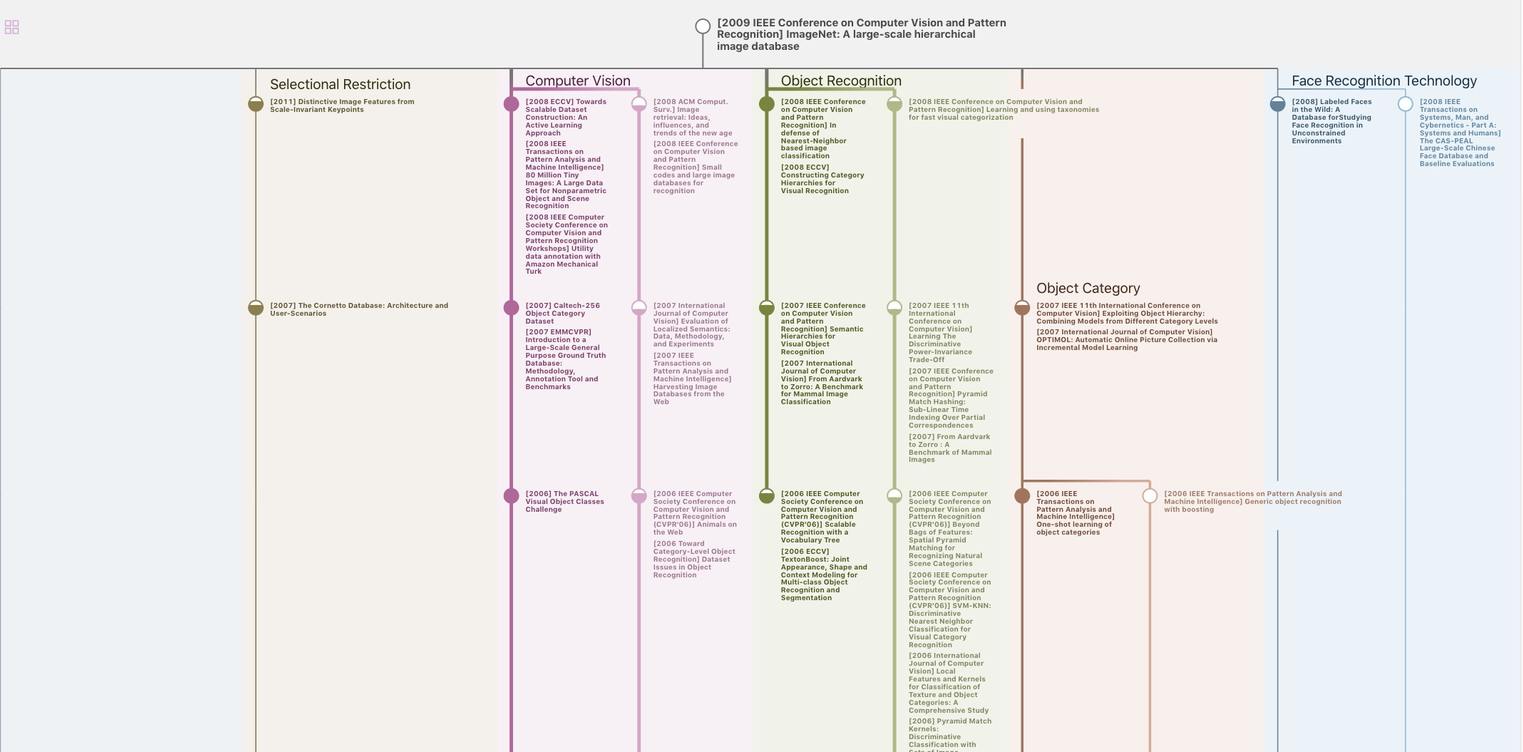
生成溯源树,研究论文发展脉络
Chat Paper
正在生成论文摘要