Efficient Video Transformers via Spatial-temporal Token Merging for Action Recognition
ACM TRANSACTIONS ON MULTIMEDIA COMPUTING COMMUNICATIONS AND APPLICATIONS(2024)
摘要
Transformer has exhibited promising performance in various video recognition tasks but brings a huge computational cost in modeling spatial-temporal cues. This work aims to boost the efficiency of existing video transformers for action recognition through eliminating redundancies in their tokens and efficiently learning motion cues of moving objects. We propose a lightweight and plug-and-play module, namely Spatial-temporal Token Merger (STTM), to merge the tokens belonging to the same object into a more compact representation. STTM first adaptively identifies crucial object clues underlying the video as meta tokens. Similarity scores between input tokens and meta tokens are hence computed and used to guide the fusion of similar tokens in both spatial and temporal domains, respectively. To compensate for motion cues lost in the merging procedure, we compute the linear aggregation of spatial-temporal positions of tokens as motion features. STTM hence outputs a compact set of tokens fusing both appearance and motion features of moving objects. This procedure substantially decreases the number of tokens that need to be processed by each Transformer block and boosts the efficiency. As a general module, STTM can be applied to different layers of various video Transformers. Extensive experiments on the action recognition datasets Kinectics-400 and SSv2 demonstrate its promising performance. For example, it reduces the computation complexity of ViT by 38% while maintaining a similar performance on Kinectics-400. It also brings 1.7% gains of top-1 accuracy on SSv2 under the same computational cost.
更多查看译文
关键词
Efficient video recognition,deep learning,transformer,spatial-temporal information
AI 理解论文
溯源树
样例
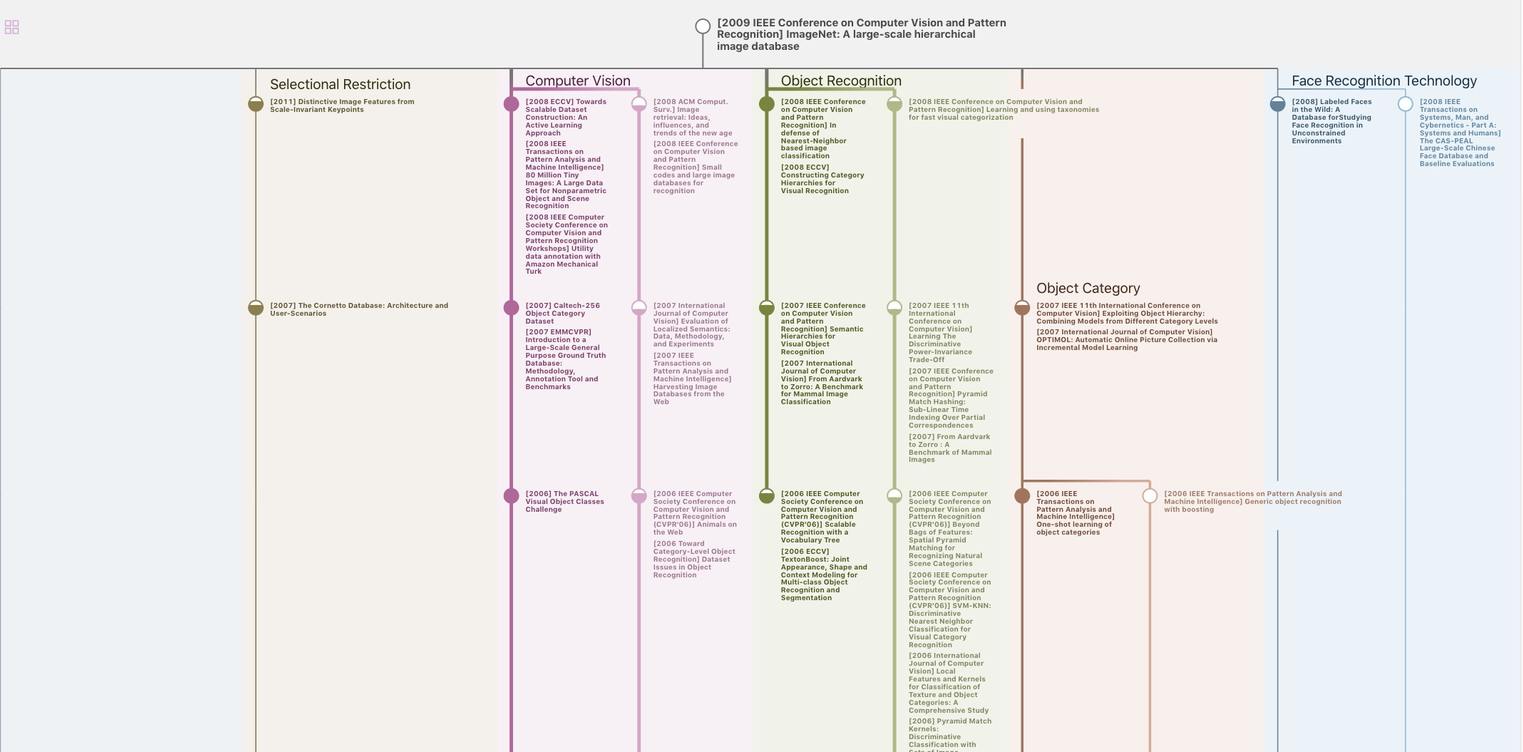
生成溯源树,研究论文发展脉络
Chat Paper
正在生成论文摘要