Unbiased, Effective, and Efficient Distillation from Heterogeneous Models for Recommender Systems
ACM Transactions on Recommender Systems(2023)
摘要
In recent years, recommender systems have achieved remarkable performance by using ensembles of heterogeneous models. However, this approach is costly due to the resources and inference latency proportional to the number of models, creating a bottleneck for production. Our work aims to transfer the ensemble knowledge of heterogeneous teachers to a lightweight student model using knowledge distillation (KD), reducing inference costs while maintaining high accuracy. We find that the efficacy of distillation decreases when transferring knowledge from heterogeneous teachers. To address this, we propose a new KD framework, named HetComp, that guides the student model by transferring easy-to-hard sequences of knowledge generated from teachers’ trajectories. HetComp uses dynamic knowledge construction to provide progressively difficult ranking knowledge and adaptive knowledge transfer to gradually transfer finer-grained ranking information. Although HetComp improves accuracy, it exacerbates popularity bias, resulting in a high popularity lift. To mitigate this issue, we introduce two strategies that leverage models’ disagreement knowledge (i.e., dissensus) for heterogeneous comparison. Our experiments demonstrate that HetComp significantly enhances distillation quality and the student model’s generalization capabilities. Furthermore, we provide extensive experimental results supporting the effectiveness of our dissensus-based debiasing techniques in mitigating the popularity lift caused by HetComp.
更多查看译文
关键词
Recommender system,Knowledge distillation,Model compression,Easy-to-hard learning,Popularity amplification,Dissensus learning
AI 理解论文
溯源树
样例
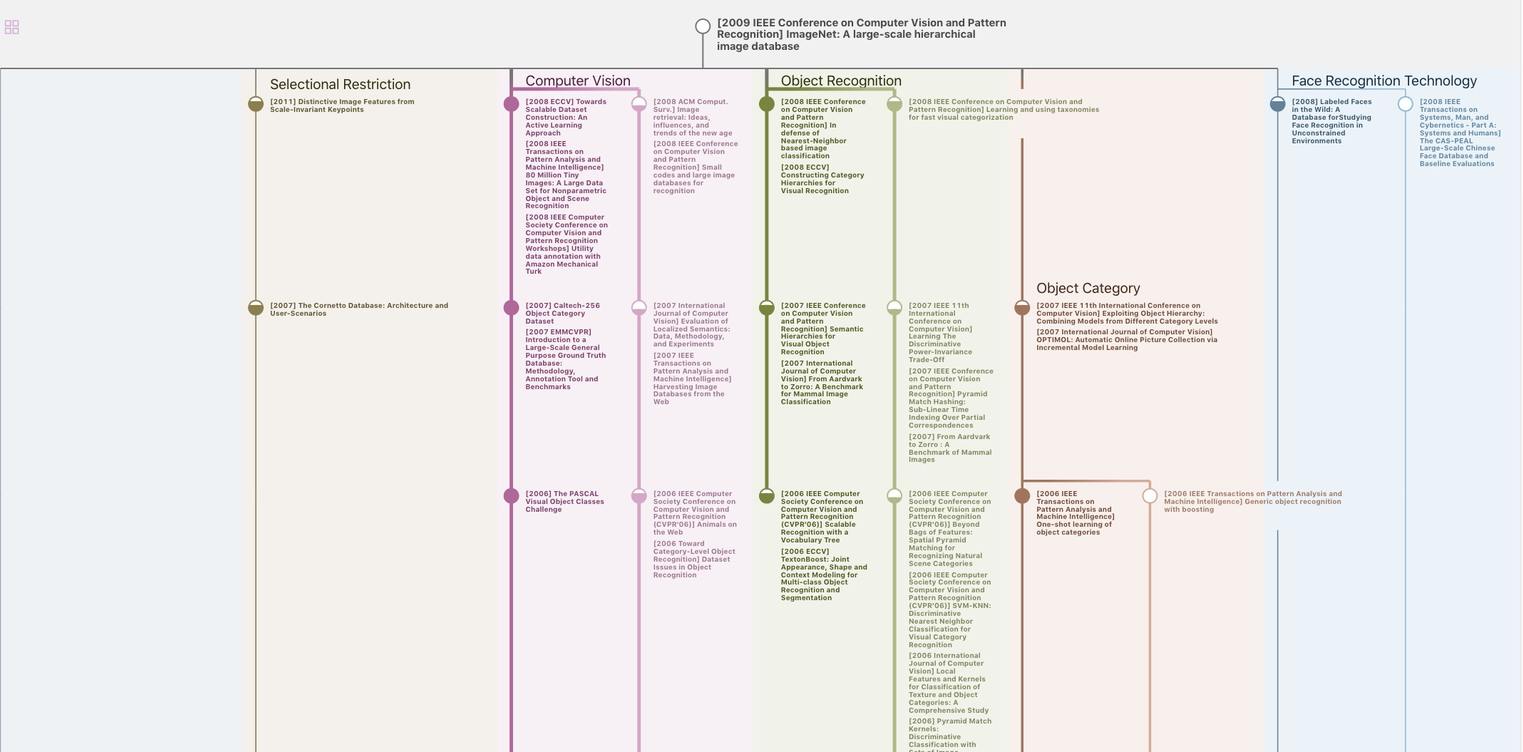
生成溯源树,研究论文发展脉络
Chat Paper
正在生成论文摘要