Graph-based social relation inference with multi-level conditional attention
Neural Networks(2024)
摘要
Social relation inference intrinsically requires high-level semantic understanding. In order to accurately infer relations of persons in images, one needs not only to understand scenes and objects in images, but also to adaptively attend to important clues. Unlike prior works of classifying social relations using attention on detected objects, we propose a MUlti-level Conditional Attention (MUCA) mechanism for social relation inference, which attends to scenes, objects and human interactions based on each person pair. Then, we develop a transformer-style network to achieve the MUCA mechanism. The novel network named as Graph-based Relation Inference Transformer (i.e., GRIT) consists of two modules, i.e., a Conditional Query Module (CQM) and a Relation Attention Module (RAM). Specifically, we design a graph-based CQM to generate informative relation queries for all person pairs, which fuses local features and global context for each person pair. Moreover, we fully take advantage of transformer-style networks in RAM for multi-level attentions in classifying social relations. To our best knowledge, GRIT is the first for inferring social relations with multi-level conditional attention. GRIT is end-to-end trainable and significantly outperforms existing methods on two benchmark datasets, e.g., with performance improvement of 7.8% on PIPA and 9.6% on PISC.
更多查看译文
关键词
Social relation inference,Multi-level conditional attention,Transformer
AI 理解论文
溯源树
样例
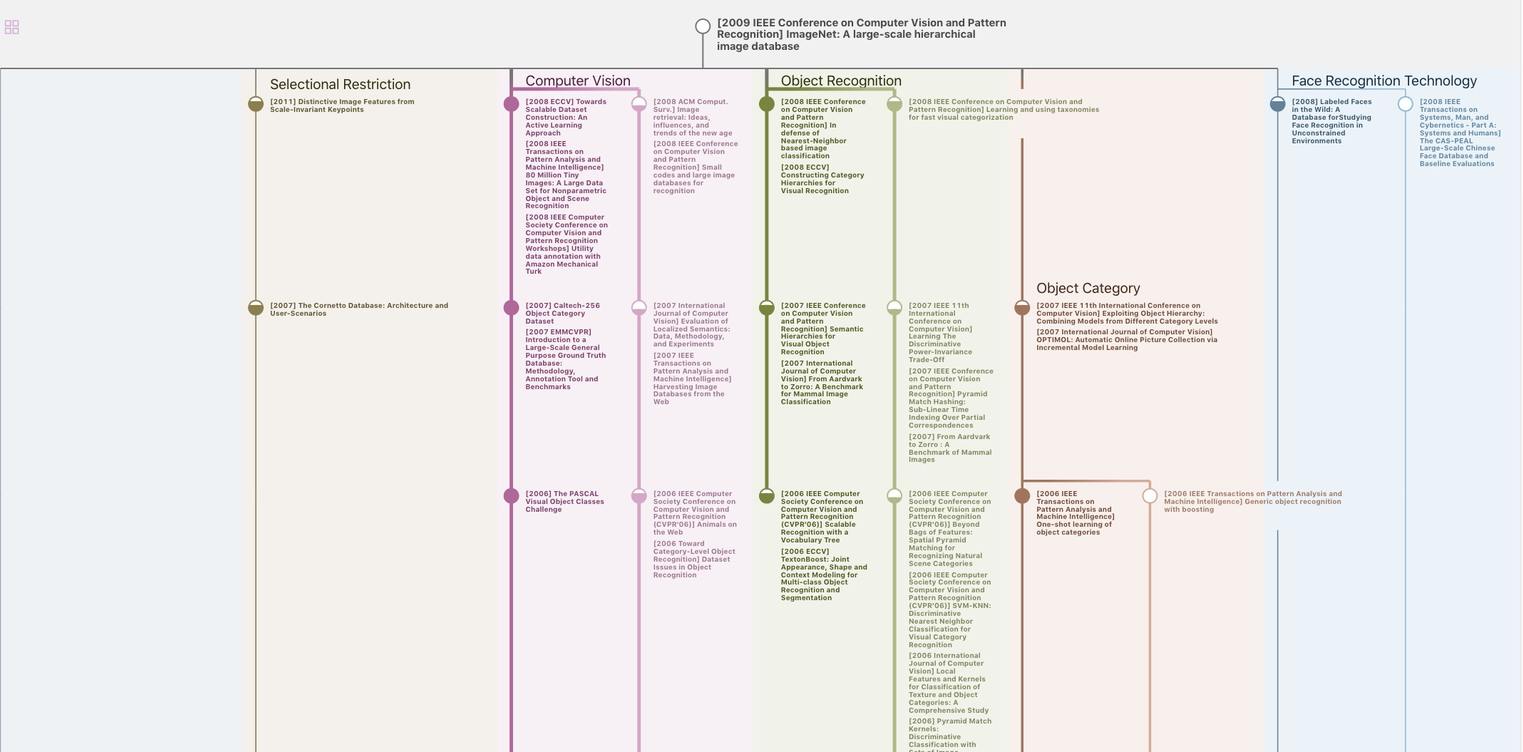
生成溯源树,研究论文发展脉络
Chat Paper
正在生成论文摘要