Game Analysis and Incentive Mechanism Design for Differentially Private Cross-silo Federated Learning
IEEE Transactions on Mobile Computing(2024)
摘要
Cross-silo federated learning (FL) is a distributed learning method where clients collaboratively train a global model without exchanging local data. However, recent works reveal that potential privacy leakage occurs when clients upload their local updates. Although some works have studied privacy-preserving mechanisms in FL, the selfish privacy-preserving behaviors of clients (who are usually cost-sensitive companies or organizations) are yet to be explored. In this paper, we formulate clients' privacy-preserving behaviors in cross-silo FL as a multi-stage privacy preservation game, where each stage game corresponds to one training iteration. Specifically, clients selfishly perturb their local updates in each training iteration to trade off between convergence performance and privacy loss. To analyze the game, we first derive a novel theoretical bound to characterize the impact of clients' local perturbations on the convergence of FL through analyzing the corrective effect of gradient descent in model training. With the novel convergence bound, we prove that each stage game is a potential game with a unique Nash equilibrium (NE) and the multi-stage privacy preservation game admits a unique subgame perfect Nash equilibrium (SPNE). We show that at the SPNE, the magnitude of each client's local perturbation decreases geometrically with training iterations. We then characterize the efficiency of the SPNE in terms of social cost by the price of anarchy (PoA), and show that the efficiency decreases with the number of clients in some cases. To tackle this problem, we propose a socially efficient incentive mechanism that allows monetary transfer among clients and guarantees individual rationality, budget balance, and social efficiency. To further elicit the private information from the selfish clients, we propose a truthful mechanism that achieves approximate social efficiency. Simulation results show that our proposed mechanisms are effective even when clients are highly heterogeneous, and can decrease clients' total cost by up to 58.08% compared with that at the SPNE.
更多查看译文
关键词
federated learning,differential privacy,game analysis,incentive mechanism
AI 理解论文
溯源树
样例
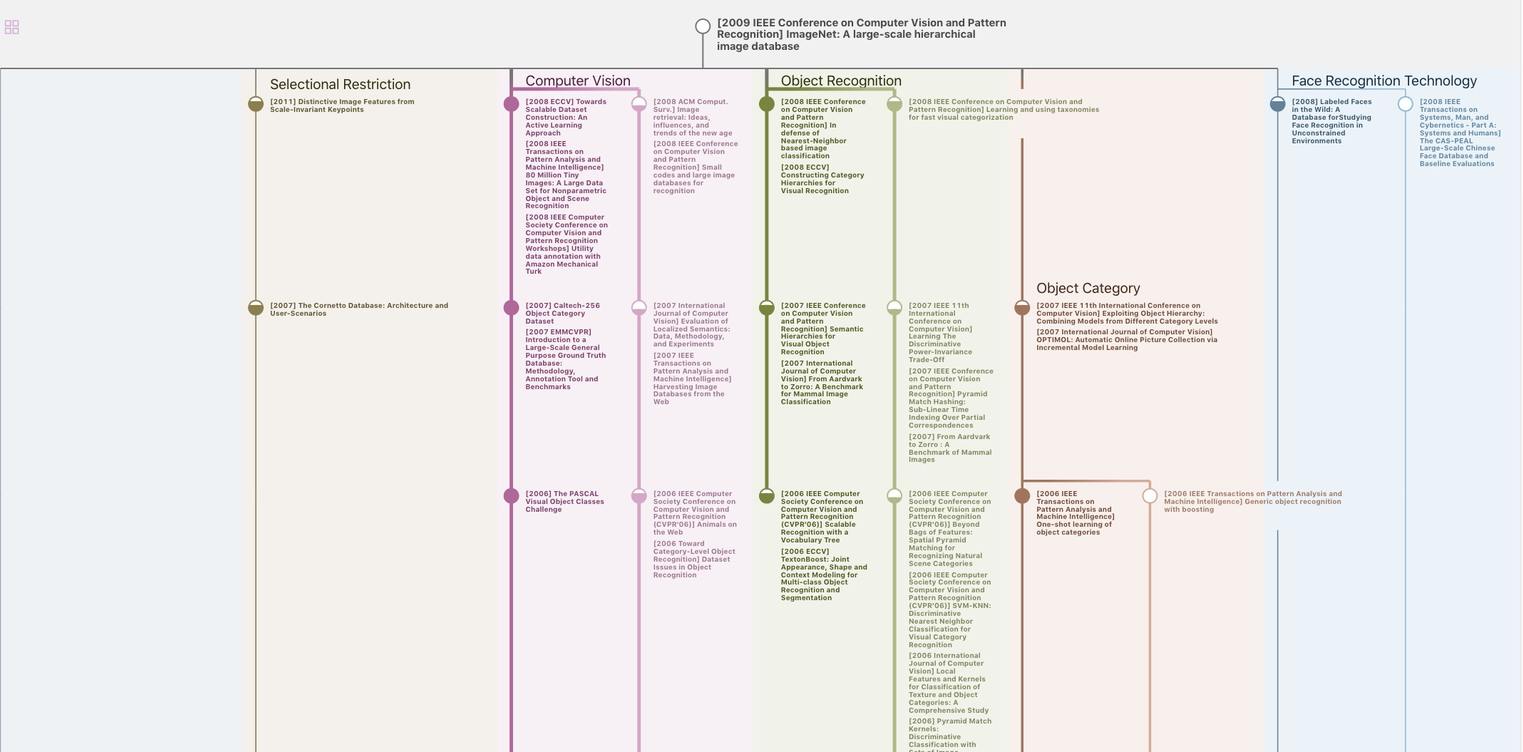
生成溯源树,研究论文发展脉络
Chat Paper
正在生成论文摘要