Bayesian Fault Detection and Localization Through Wireless Sensor Networks in Industrial Plants.
IEEE Internet Things J.(2024)
摘要
This work proposes a data fusion approach for quickest fault detection and localization within industrial plants via wireless sensor networks. Two approaches are proposed, each exploiting different network architectures. In the first approach, multiple sensors monitor a plant section and individually report their local decisions to a fusion center. The fusion center provides a global decision after spatial aggregation of the local decisions. A post-processing center subsequently processes these global decisions in time, which performs quick detection and localization. Alternatively, the fusion center directly performs a spatio-temporal aggregation directed at quickest detection, together with a possible estimation of the faulty item. Both architectures are provided with a feedback system where the network’s highest hierarchical level transmits parameters to the lower levels. The two proposed approaches model the faults according to a Bayesian criterion and exploit the knowledge of the reliability model of the plant under monitoring. Moreover, adaptations of the well-known Shewhart and CUSUM charts are provided to fit the different architectures and are used for comparison purposes. Finally, the algorithms are tested via simulation on an active Oil and Gas subsea production system, and performances are provided.
更多查看译文
关键词
Data fusion,fault detection,Industry 4.0,localization,monitoring,quickest detection,reliability,wireless sensor network
AI 理解论文
溯源树
样例
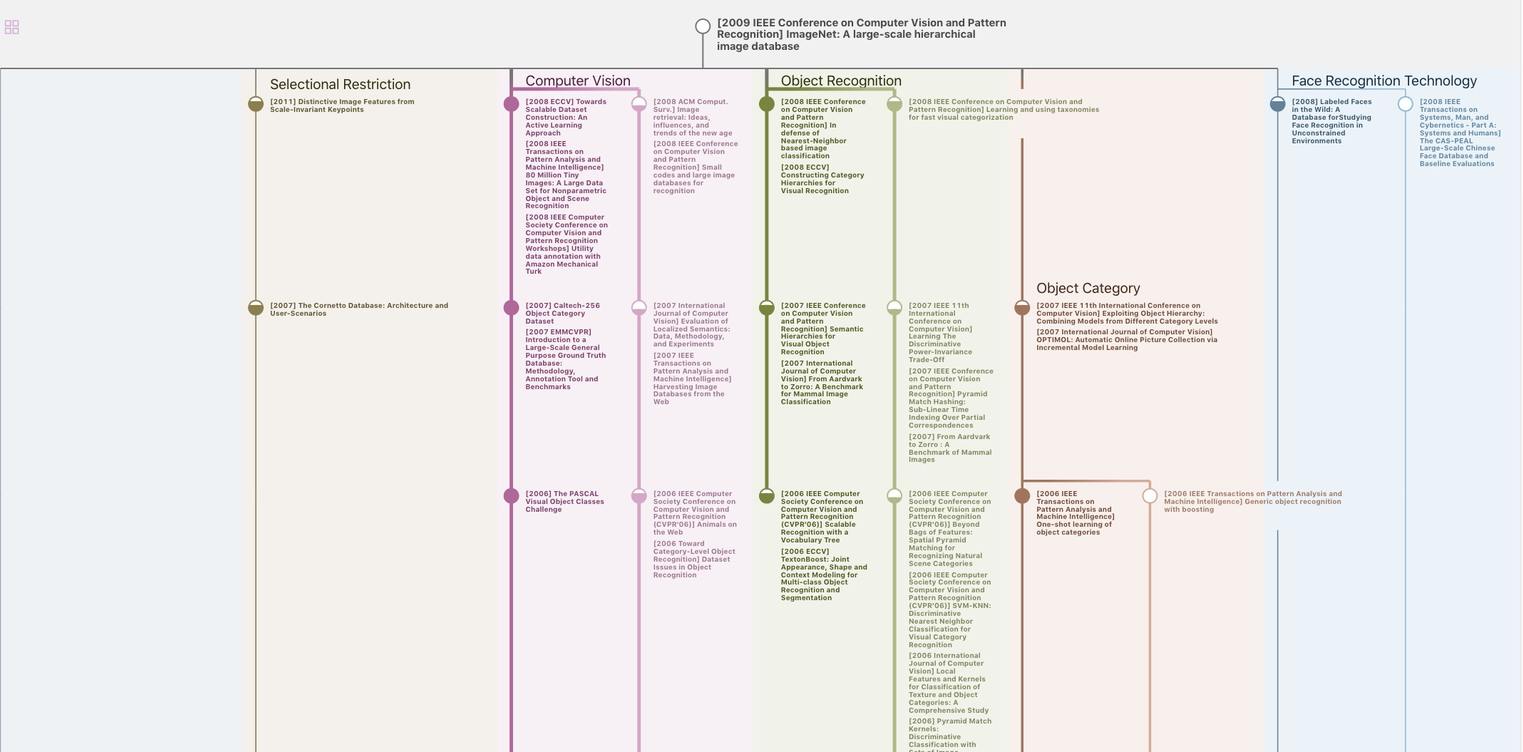
生成溯源树,研究论文发展脉络
Chat Paper
正在生成论文摘要