Certain and Approximately Certain Models for Statistical Learning
CoRR(2024)
摘要
Real-world data is often incomplete and contains missing values. To train
accurate models over real-world datasets, users need to spend a substantial
amount of time and resources imputing and finding proper values for missing
data items. In this paper, we demonstrate that it is possible to learn accurate
models directly from data with missing values for certain training data and
target models. We propose a unified approach for checking the necessity of data
imputation to learn accurate models across various widely-used machine learning
paradigms. We build efficient algorithms with theoretical guarantees to check
this necessity and return accurate models in cases where imputation is
unnecessary. Our extensive experiments indicate that our proposed algorithms
significantly reduce the amount of time and effort needed for data imputation
without imposing considerable computational overhead.
更多查看译文
关键词
data preparation,data quality,uncertainty quantification
AI 理解论文
溯源树
样例
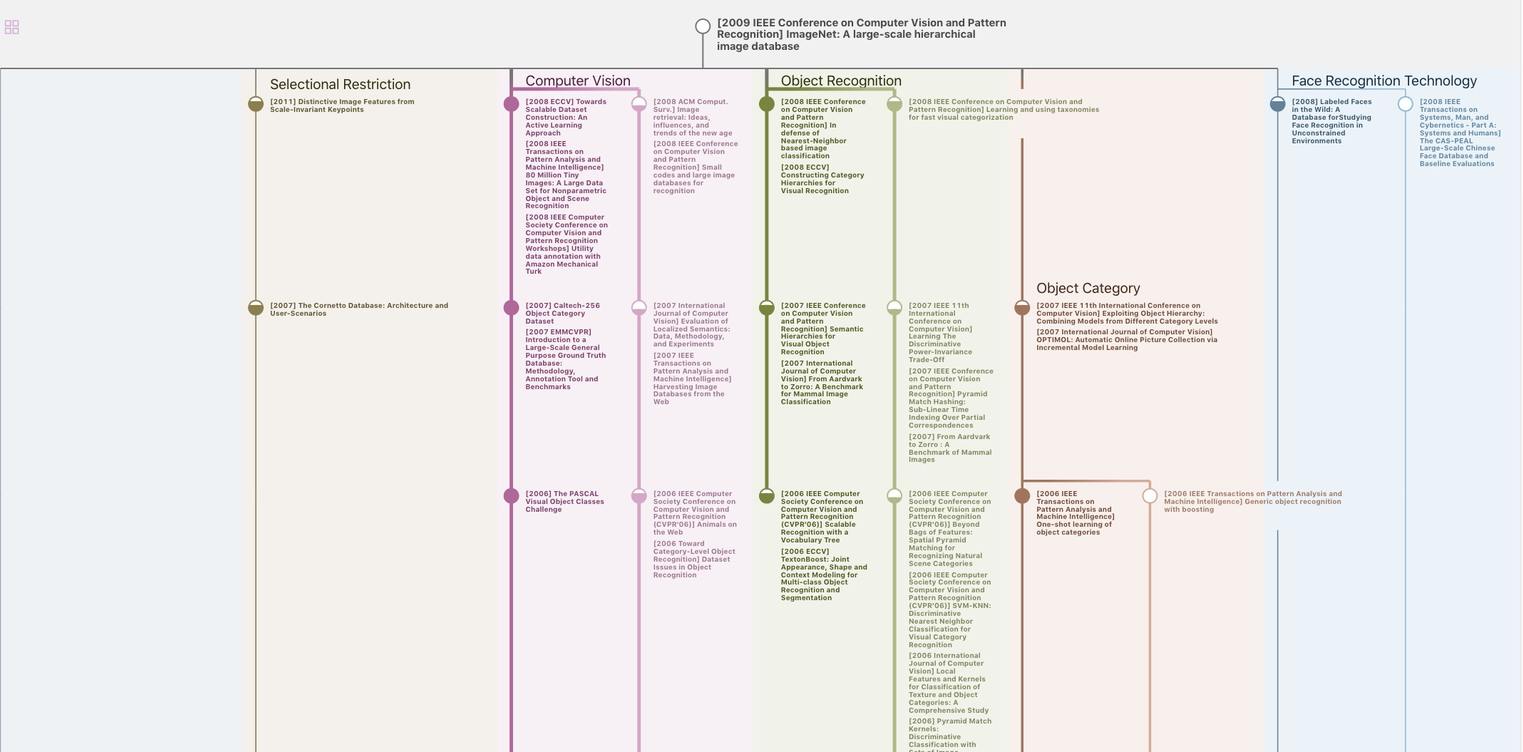
生成溯源树,研究论文发展脉络
Chat Paper
正在生成论文摘要