Error Concealment of Dynamic LiDAR Point Clouds for Connected and Autonomous Vehicles
IEEE CONFERENCE ON GLOBAL COMMUNICATIONS, GLOBECOM(2023)
摘要
Connected and Autonomous Vehicles (CAVs) often come with sensors, such as LiDARs, to improve road safety. Although dynamic LiDAR point clouds could be streamed over wireless networks from CAVs to edge servers for computationally-intensive classification tasks, the problem of incomplete point cloud frames caused by unreliable wireless networks has yet to be investigated in the literature. In this paper, we propose the very first LiDAR Error Concealment (LEC) algorithm, to conceal incomplete point cloud frames due to packet loss or late packets to minimize the distortion in Chamfer distance. Driven by machine learning techniques, our LEC algorithm adaptively performs Temporal Prediction (TP), Spatial Interpolation (SI), or Temporal Interpolation (TI) to conceal incomplete point cloud frames. We evaluate the performance of our LEC algorithms with a comprehensive co-simulator of the popular CARLA and NS-3 implemented by us and the KITTI Odometry dataset. Our simulation results reveal that the proposed LEC algorithm outperforms the TP, SI, and TI algorithms by up to 82.68% and 30.17% in Chamfer and Hausdorff distances, and terminates in 360-570 ms in a C-V2X network. Moreover, our LEC algorithm also outperforms other algorithms by up to 87.43% and 66.58% in Chamfer and Hausdorff distances in a DSRC network.
更多查看译文
关键词
Connected Vehicles,Autonomous Vehicles,LiDARs,Point Cloud,Streaming,Road Safety,Error Concealment
AI 理解论文
溯源树
样例
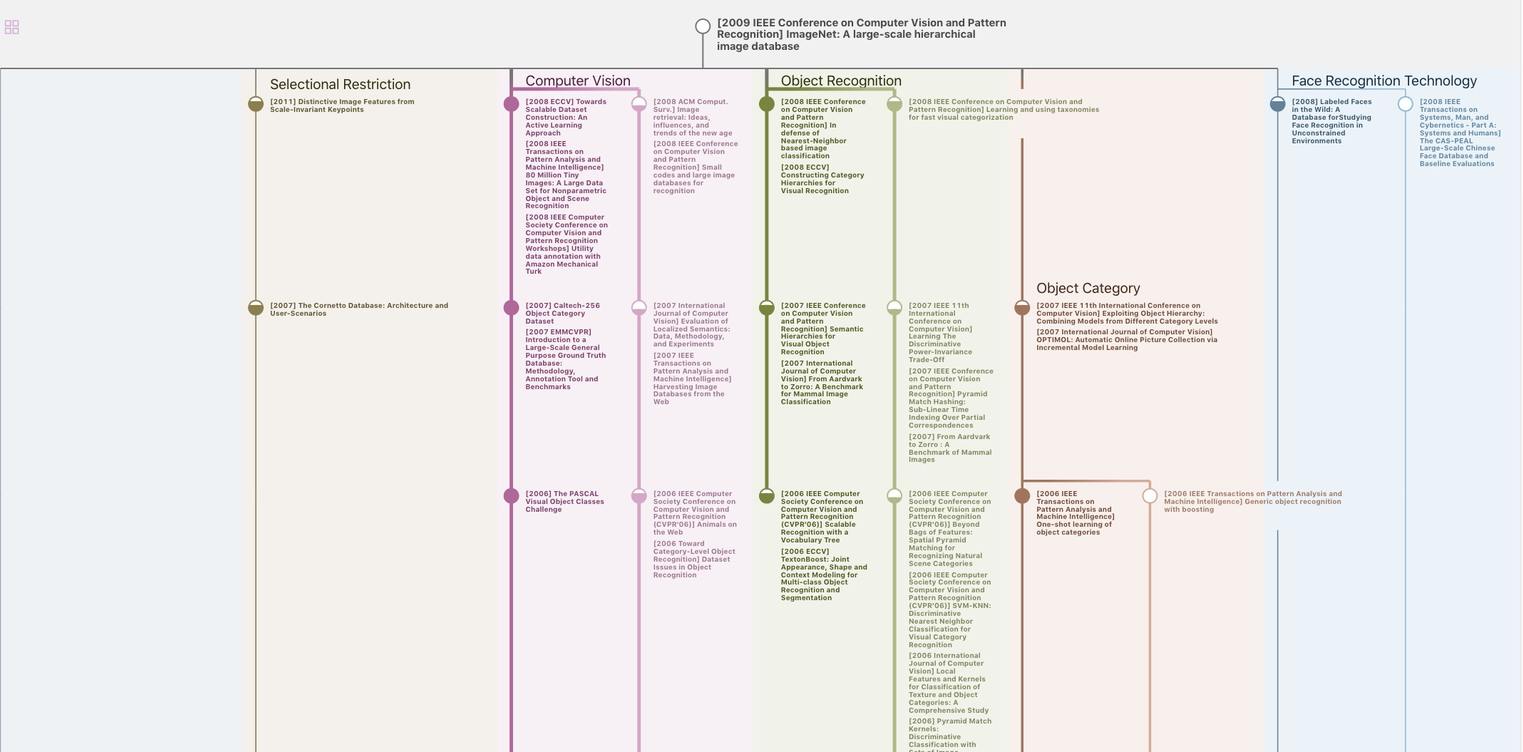
生成溯源树,研究论文发展脉络
Chat Paper
正在生成论文摘要