Multi-Switch Cooperative In-Network Aggregation for Distributed Deep Learning
IEEE CONFERENCE ON GLOBAL COMMUNICATIONS, GLOBECOM(2023)
摘要
Distributed deep learning (DDL) has recently been proposed to accelerate the training process of a deep learning model. The core idea is to have multiple workers collaboratively train a model in parallel. DDL, however, relies on synchronization among participating workers, which introduces significant communication overhead. To resolve this issue, recent research has demonstrated the effectiveness of in-network aggregation (INA), which reduces the bandwidth requirement of DDL training by allowing a programmable switch to combine the parameters of workers and only forward the aggregated parameter to the master. We, however, notice that existing approaches mainly focus on single-switch in-network aggregation and may overload a switch when the number of competing training jobs grows. In this work, we present Multi-Switch In-Network Aggregation (MS-INA), a system that efficiently offloads the aggregation load of DDL jobs across the switches of a network. To fully leverage the potential of all the available programmable switches for aggregation jobs, we assign each training job an aggregator switch that minimizes the end-to-end training latency. To this end, our MS-INA identifies the optimal switch that not only performs efficient aggregation but also introduces a short parameter forwarding latency. The trace-driven evaluation demonstrates that MS-INA effectively leverages the computational capability of all the switches and increases the number of successfully aggregated parameters by up to 5.3x as compared to conventional single-switch INA. The increasing aggregation capability contributes to bandwidth reduction by up to 64%.
更多查看译文
关键词
In-network Aggregation,SDN,Distributed Deep Learning
AI 理解论文
溯源树
样例
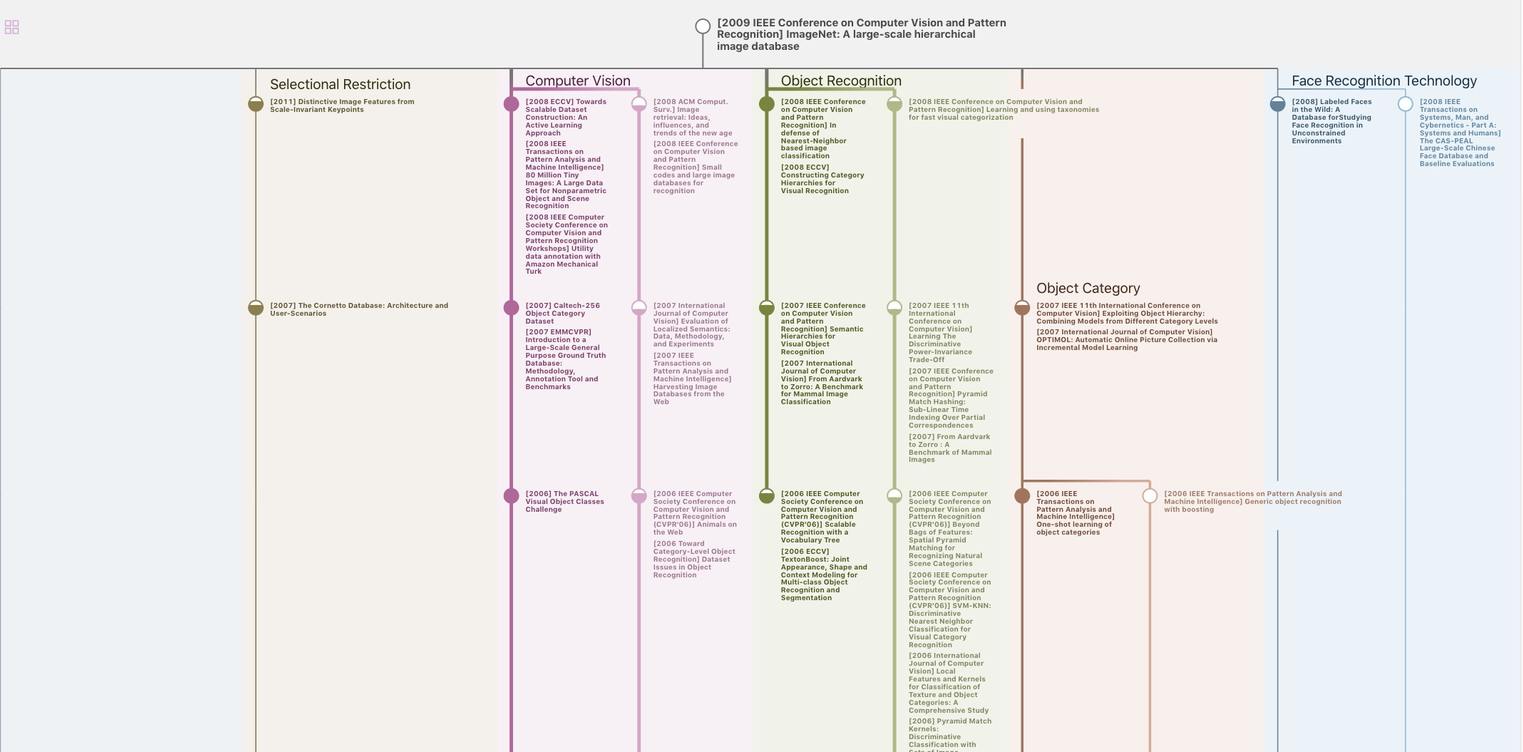
生成溯源树,研究论文发展脉络
Chat Paper
正在生成论文摘要