Best of Many in Both Worlds: Online Resource Allocation with Predictions under Unknown Arrival Model
CoRR(2024)
摘要
Online decision-makers today can often obtain predictions on future
variables, such as arrivals, demands, inventories, and so on. These predictions
can be generated from simple forecasting algorithms for univariate time-series,
all the way to state-of-the-art machine learning models that leverage multiple
time-series and additional feature information. However, the prediction quality
is often unknown to decisions-makers a priori, hence blindly following the
predictions can be harmful. In this paper, we address this problem by giving
algorithms that take predictions as inputs and perform robustly against the
unknown prediction quality.
We consider the online resource allocation problem, one of the most generic
models in revenue management and online decision-making. In this problem, a
decision maker has a limited amount of resources, and requests arrive
sequentially. For each request, the decision-maker needs to decide on an
action, which generates a certain amount of rewards and consumes a certain
amount of resources, without knowing the future requests. The decision-maker's
objective is to maximize the total rewards subject to resource constraints. We
take the shadow price of each resource as prediction, which can be obtained by
predictions on future requests. Prediction quality is naturally defined to be
the ℓ_1 distance between the prediction and the actual shadow price. Our
main contribution is an algorithm which takes the prediction of unknown quality
as an input, and achieves asymptotically optimal performance under both
requests arrival models (stochastic and adversarial) without knowing the
prediction quality and the requests arrival model beforehand. We show our
algorithm's performance matches the best achievable performance of any
algorithm had the arrival models and the accuracy of the predictions been
known. We empirically validate our algorithm with experiments.
更多查看译文
AI 理解论文
溯源树
样例
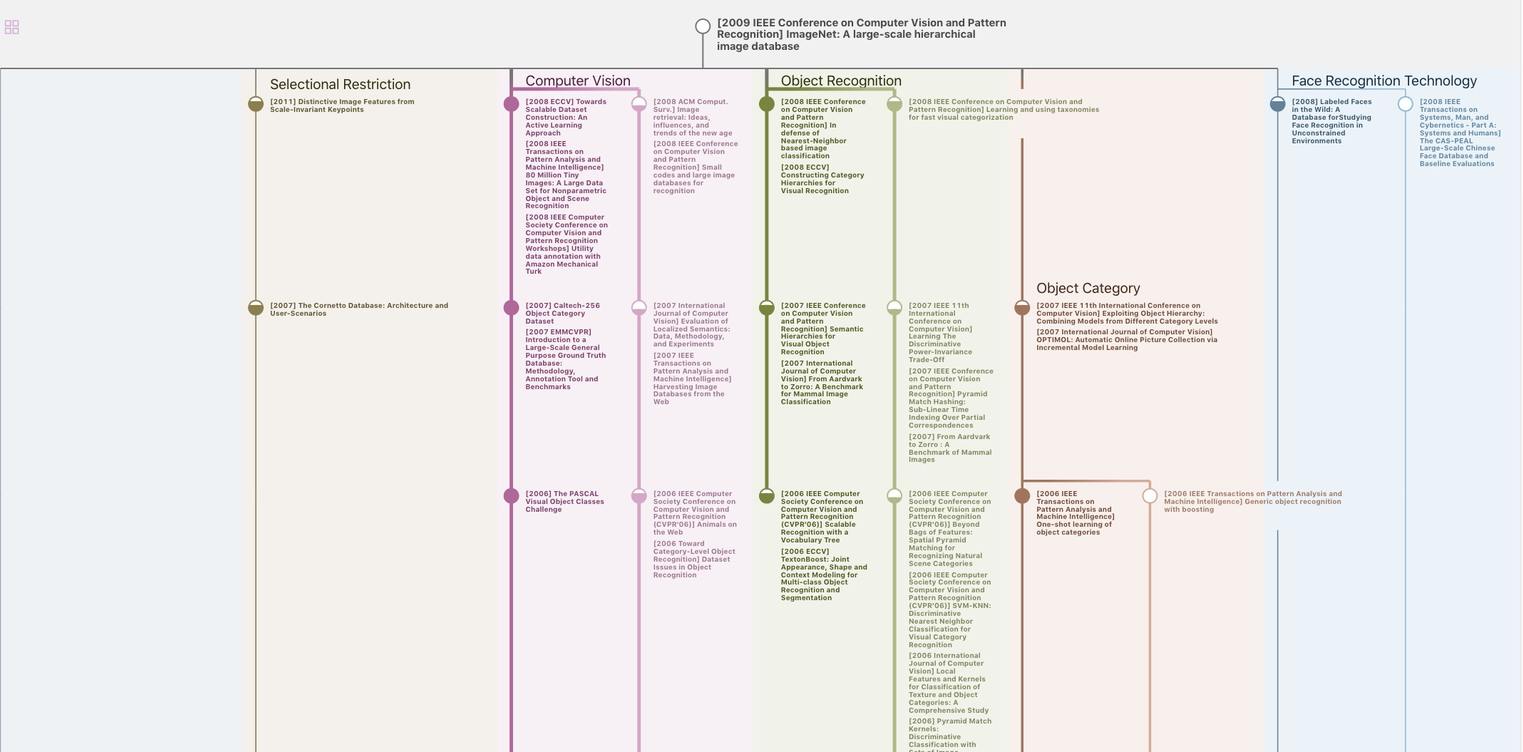
生成溯源树,研究论文发展脉络
Chat Paper
正在生成论文摘要