Using Area Under the Precision Recall Curve to Assess the Effect of Random Undersampling in the Classification of Imbalanced Medicare Big Data
INTERNATIONAL JOURNAL OF RELIABILITY QUALITY AND SAFETY ENGINEERING(2024)
摘要
In this paper, we investigate the impact of Random Undersampling (RUS) on a supervised Machine Learning task involving highly imbalanced Big Data. We present the results of experiments in Medicare Fraud detection. To the best of our knowledge, these experiments are conducted with the largest insurance claims datasets ever used for Medicare Fraud detection. We obtain two datasets from two Big Data repositories provided by the United States government's Centers for Medicare and Medicaid Services. The larger of the two datasets contains nearly 174 million instances, with a minority to majority class ratio of approximately 0.0039. Our contribution is to show that RUS has a detrimental effect on a Medicare Fraud detection task when performed on large scale, imbalanced data. The effect of RUS is apparent in the Area Under the Precision Recall Curve (AUPRC) scores recorded from experimental outcomes. We use four popular, open-source classifiers in our experiments to confirm the negative impact of RUS on their AUPRC scores.
更多查看译文
关键词
Bagging,Boosting,class imbalance,Big Data,Random Undersampling
AI 理解论文
溯源树
样例
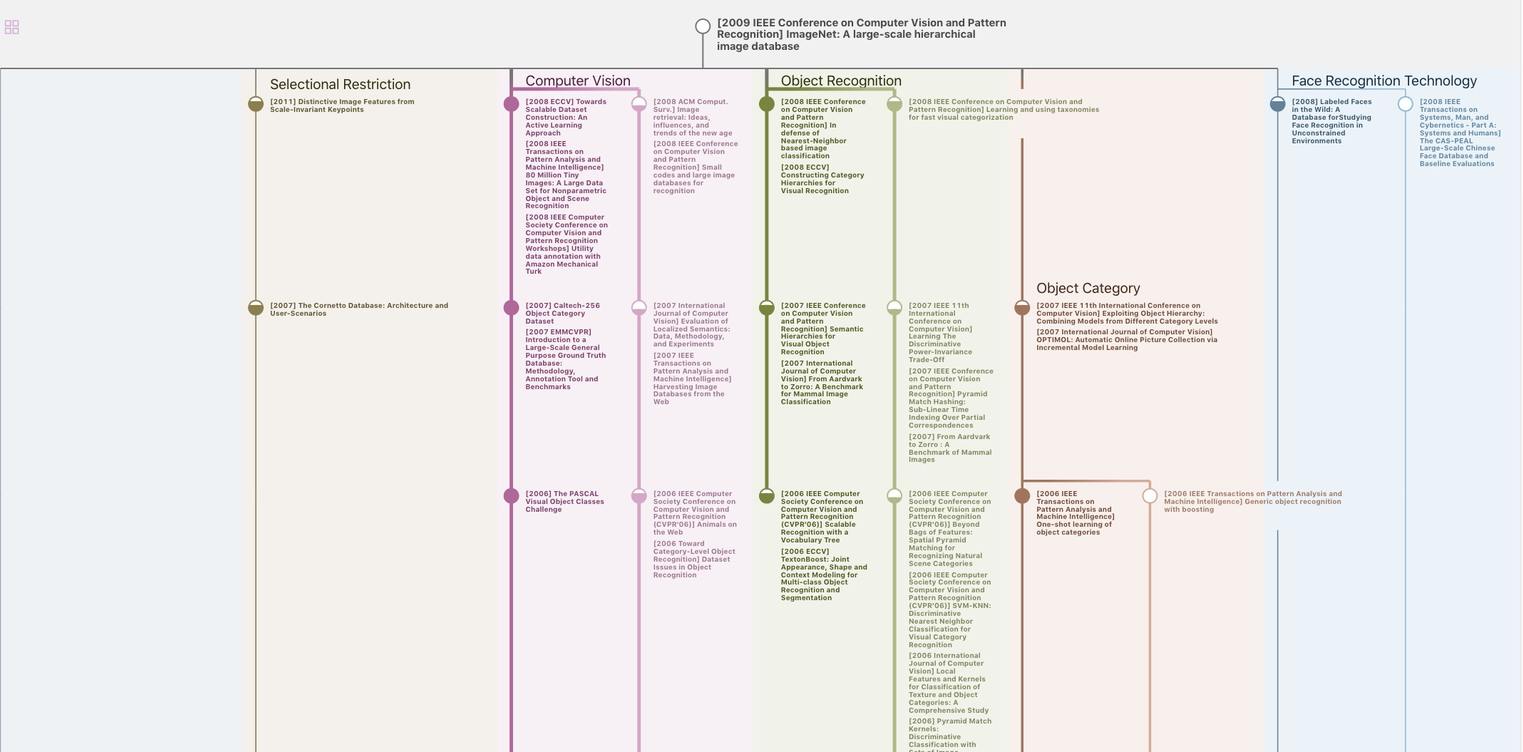
生成溯源树,研究论文发展脉络
Chat Paper
正在生成论文摘要