Improved Lower Bounds for Approximating Parameterized Nearest Codeword and Related Problems under ETH
CoRR(2024)
摘要
In this paper we present a new gap-creating randomized self-reduction for
parameterized Maximum Likelihood Decoding problem over 𝔽_p
(k-MLD_p). The reduction takes a k-MLD_p instance with k· n
vectors as input, runs in time f(k)n^O(1) for some computable function f,
outputs a (3/2-ε)-Gap-k'-MLD_p instance for any
ε>0, where k'=O(k^2log k). Using this reduction, we show that
assuming the randomized Exponential Time Hypothesis (ETH), no algorithms can
approximate k-MLD_p (and therefore its dual problem k-NCP_p) within
factor (3/2-ε) in f(k)· n^o(√(k/log k)) time for any
ε>0.
We then use reduction by Bhattacharyya, Ghoshal, Karthik and Manurangsi
(ICALP 2018) to amplify the (3/2-ε)-gap to any constant. As a
result, we show that assuming ETH, no algorithms can approximate k-NCP_p
and k-MDP_p within γ-factor in f(k)n^o(k^ε_γ)
time for some constant ε_γ>0. Combining with the
gap-preserving reduction by Bennett, Cheraghchi, Guruswami and Ribeiro (STOC
2023), we also obtain similar lower bounds for k-MDP_p, k-CVP_p and
k-SVP_p.
These results improve upon the previous f(k)n^Ω(𝗉𝗈𝗅𝗒log
k) lower bounds for these problems under ETH using reductions by
Bhattacharyya et al. (J.ACM 2021) and Bennett et al. (STOC 2023).
更多查看译文
AI 理解论文
溯源树
样例
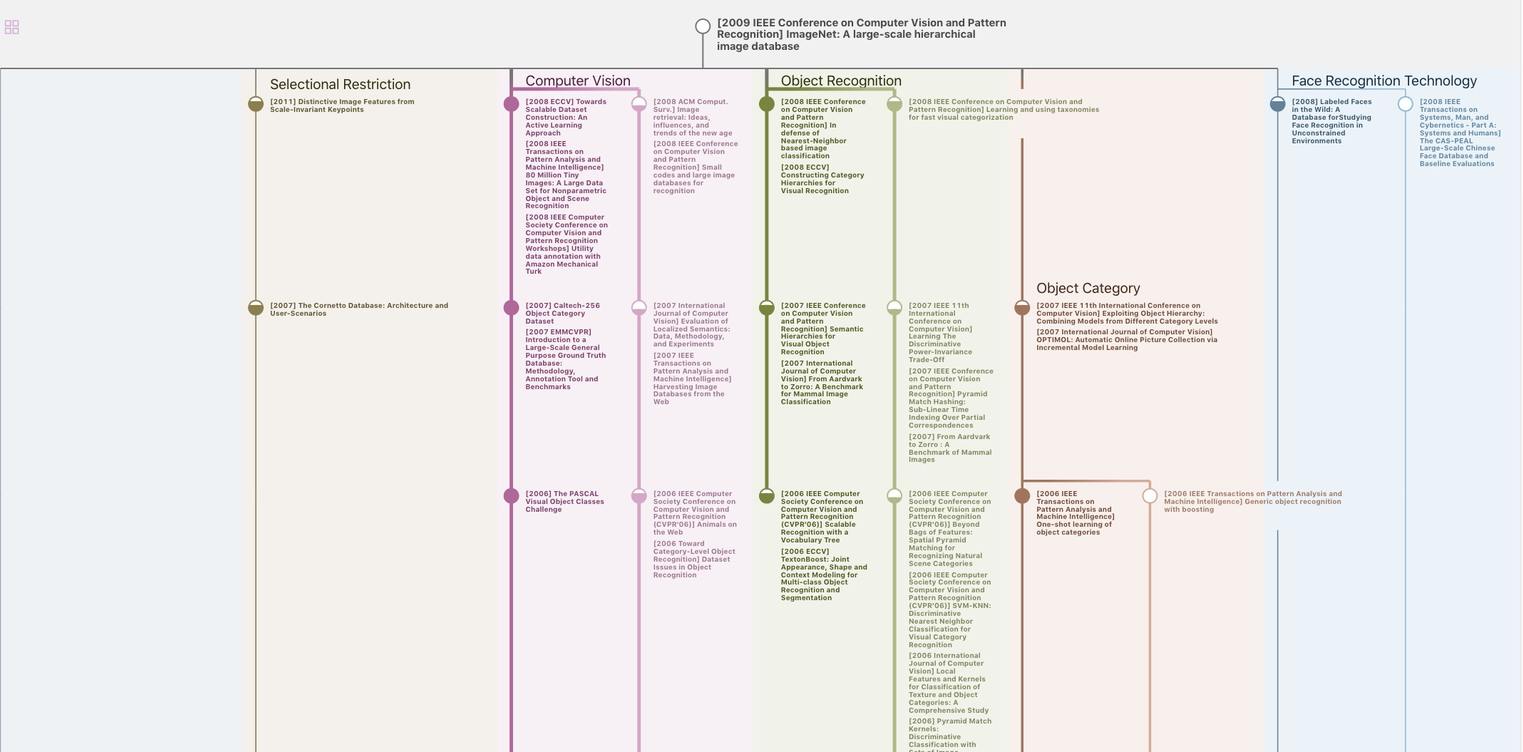
生成溯源树,研究论文发展脉络
Chat Paper
正在生成论文摘要