MinMaxMin Q-learning
CoRR(2024)
摘要
MinMaxMin Q-learning is a novel optimistic Actor-Critic
algorithm that addresses the problem of overestimation bias
(Q-estimations are overestimating the real Q-values) inherent in
conservative RL algorithms. Its core formula relies on the
disagreement among Q-networks in the form of the min-batch MaxMin
Q-networks distance which is added to the Q-target and used as the priority
experience replay sampling-rule. We implement MinMaxMin on top of TD3
and TD7, subjecting it to rigorous testing against state-of-the-art
continuous-space algorithms-DDPG, TD3, and TD7-across popular MuJoCo and Bullet
environments. The results show a consistent performance improvement of
MinMaxMin over DDPG, TD3, and TD7 across all tested tasks.
更多查看译文
AI 理解论文
溯源树
样例
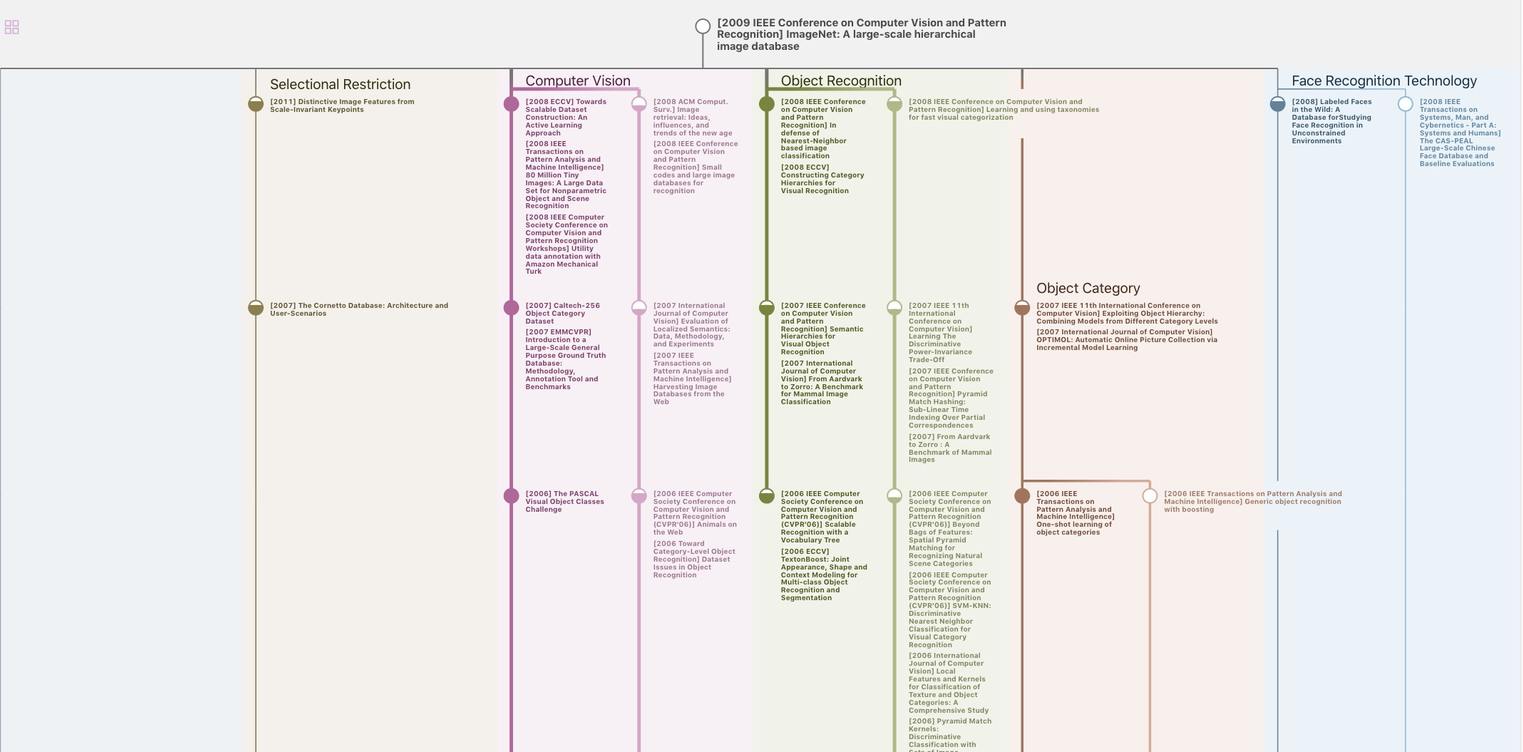
生成溯源树,研究论文发展脉络
Chat Paper
正在生成论文摘要