Dehazing Network: Asymmetric Unet Based on Physical Model
IEEE TRANSACTIONS ON GEOSCIENCE AND REMOTE SENSING(2024)
摘要
Optical remote sensing has emerged as a crucial technique for earth observation. However, interference of clouds and fog can adversely affect the spatial and spectral information of the images, presenting significant challenges in interpreting remote sensing data and limiting its availability. Moreover, existing methods for addressing the issue of cloud occlusion primarily rely on either physical models or neural networks, lacking a comprehensive integration of the advantages offered by both approaches. So, we propose an end-to-end asymmetric Unet (AU-Net) dehazing network based on an atmospheric scattering model, which realizes the joint optimization of physical parameters. First, we utilize the Unet to estimate the relatively constant atmospheric light A . Simultaneously, AU-Net based on dark channel prior guidance is used to estimate more complex transmission map T. Next, the atmospheric scattering model is used to deduce the rough dehazing image J based on A and T. Finally, we refine J using AU-Net once again. More specifically, AU-Net incorporates a plug-and-play attention module, namely, self-attention with depth (SAD) and channel attention (CA). SAD enhances the fusion of deep semantic information and shallow detail features by expanding the network's depth, thereby effectively alleviating the dependence of the network on long-distance information. On the other hand, CA addresses the limitations of SAD in terms of channel information. To validate the effectiveness and applicability of proposed dehazing network and attention module, we have achieved state-of-the-art performance and visually appealing results on remote sensing and natural image datasets. The code will be made public on https://github.com/Dudujia160918/Dehazing_Network_AU-Net .
更多查看译文
关键词
Atmospheric modeling,Neural networks,Scattering,Remote sensing,Image restoration,Convolution,Clouds,Asymmetric Unet (AU-Net),dehazing network,plug-and-play attention module,remote sensing images
AI 理解论文
溯源树
样例
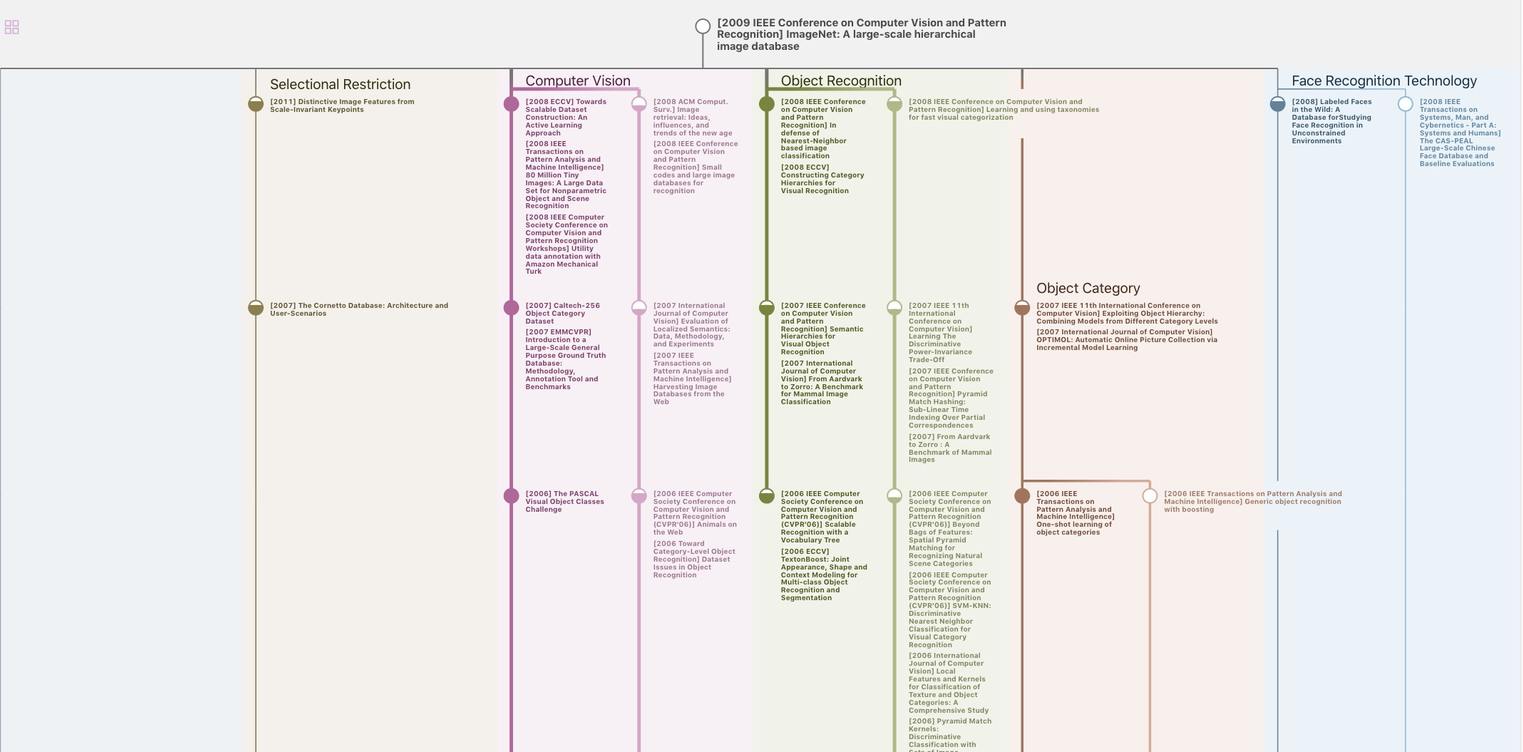
生成溯源树,研究论文发展脉络
Chat Paper
正在生成论文摘要