Bang for the Buck: Evaluating the cost-effectiveness of Heterogeneous Edge Platforms for Neural Network Workloads
2023 IEEE/ACM SYMPOSIUM ON EDGE COMPUTING, SEC 2023(2023)
摘要
Machine learning (ML) applications have experienced remarkable growth and integration into various domains. However, challenges with cloud-based deployments, such as latency, privacy, reliability, bandwidth and connectivity, have driven the popularity of deploying ML on edge devices. ML application deployment stack consists of various components such as neural network models, input frameworks, software runtime libraries and hardware architecture. Understanding the impact of different components in the ML stack on deployment effectiveness, particularly in terms of cost effectiveness, remains a challenge. In this work, we systematically analyze the diverse choices available for each component of the ML stack and their influence on deployment performance. We empirically evaluate eight heterogeneous edge platforms and eight software runtime libraries, considering various hardware components like CPUs, GPUs, NPUs, and VPUs for ML inference. Our findings contribute to a better understanding of optimizing cost effectiveness in ML deployments on edge platforms, aiding decision-making for application developers and stakeholders.
更多查看译文
关键词
Neural networks,edge computing,accelerators
AI 理解论文
溯源树
样例
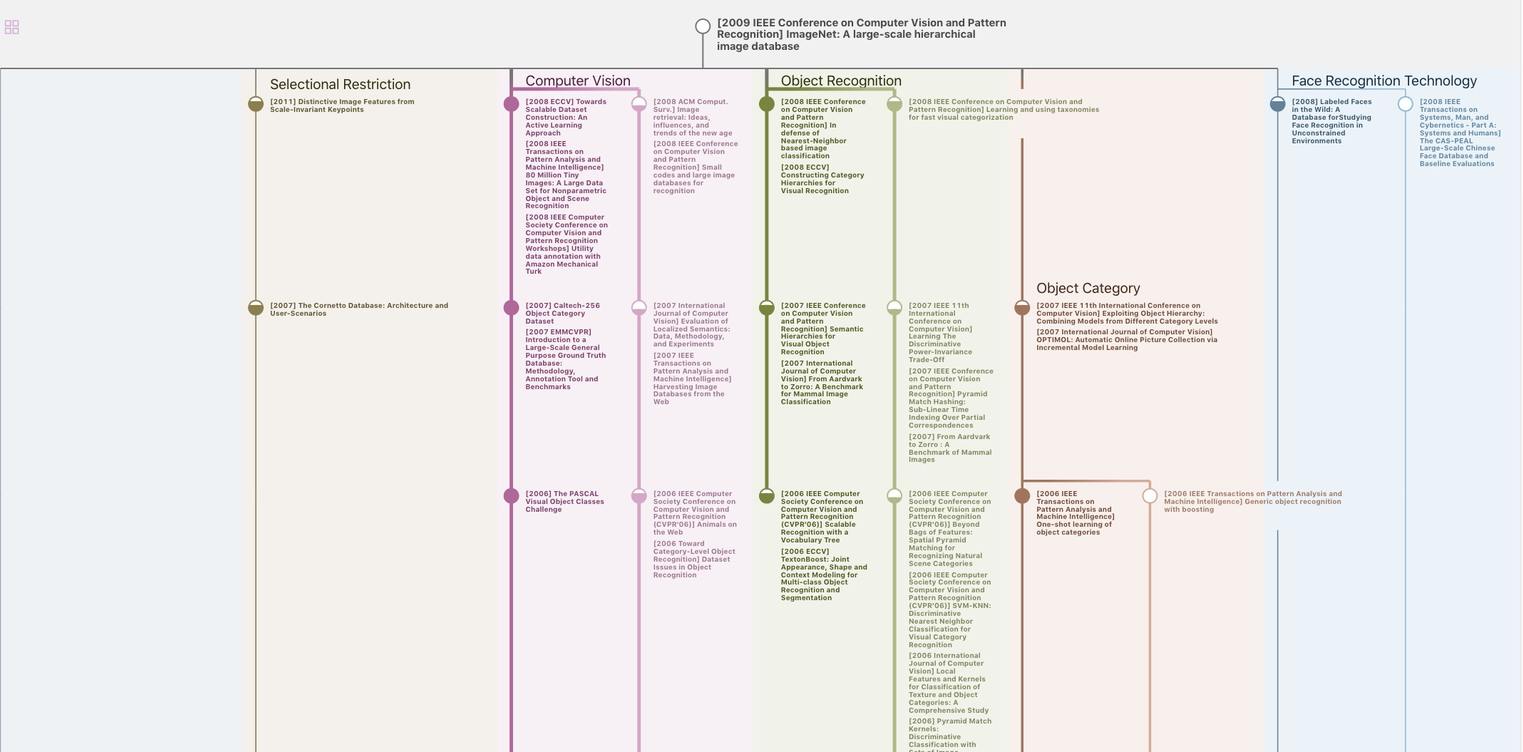
生成溯源树,研究论文发展脉络
Chat Paper
正在生成论文摘要