Context Sketching for Memory -efficient Graph Representation Learning
23RD IEEE INTERNATIONAL CONFERENCE ON DATA MINING, ICDM 2023(2023)
摘要
Graph representation learning (GRL) is fundamental in multi-graph applications like molecular property prediction. Graph neural networks (GNNs) have emerged as a popular method for GRL. However, existing GRL methods primarily focus on designing GNN models with enhanced expressiveness while overlooking the memory efficiency of algorithms during training. The memory inefficiency problem is caused by a contextual constraint imposed on node representations, which requires each node to be context-dependent on its input graph. In this paper, we propose a novel method, called context sketching (COS), for memory -efficient graph representation learning in multi-graph scenarios. We first formally define the contextual constraint based on the enclosed,Dc infinity-hop subgraphs of nodes. Subsequently, we propose to relax the original contextual constraint by requiring each node to be context-dependent on its enclosed k -hop subgraph (k,<<,infinity) which is a contextual sketch of the enclosed (Do infinity-hop subgraph. Lastly, we prove that COS constructs an optimal solution to a memory-related objective associated with graph coarsening. Experiments on four widely used benchmark datasets demonstrate that COS can reduce the memory footprint of baselines by a large margin with almost no accuracy loss.
更多查看译文
关键词
Graph Representation learning,Graph Neural,Networks,Graph]Deep Learning,Memory -efficient Learning.
AI 理解论文
溯源树
样例
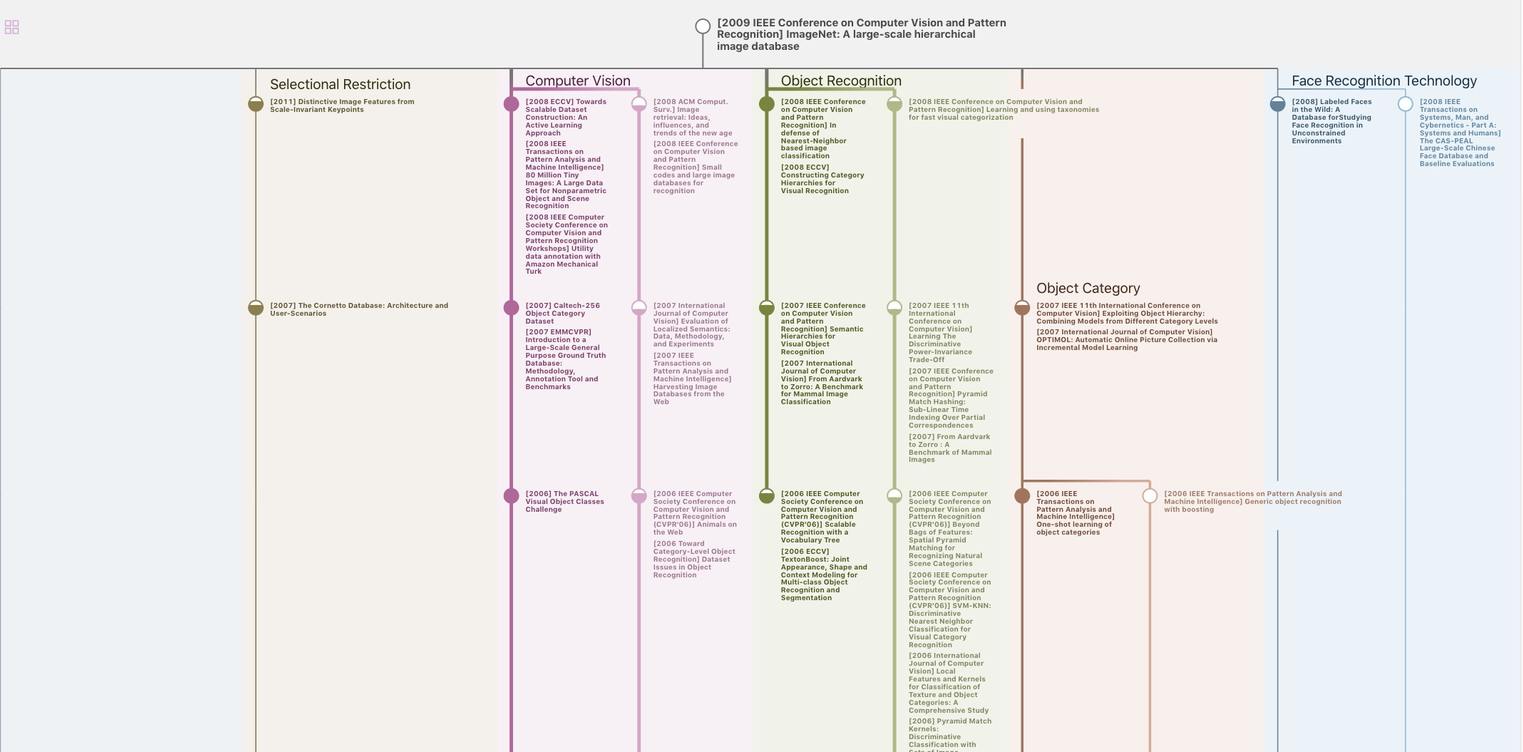
生成溯源树,研究论文发展脉络
Chat Paper
正在生成论文摘要