Two -Level Graph Representation Learning with Community -as -a -Node Graphs
23RD IEEE INTERNATIONAL CONFERENCE ON DATA MINING, ICDM 2023(2023)
摘要
In this paper, we propose a novel graph representation learning (GRL) model that aims to improve both representation accuracy and learning efficiency. We design a Two-Level GRL architecture based on the graph partitioning: 1) local GRL on nodes within each partitioned subgraph and 2) global GRL on subgraphs. By partitioning the graph through community detection, we enable elaborate node learning in the same community. Based on Two-Level GRL, we introduce an abstracted graph, Community -as-a -Node Graph (CaaN), to effectively maintain the high-level structure with a significantly reduced graph. By applying the CaaN graph to local and global GRL, we propose Two -Level GRL with Community-as-a -Node (CaaN 2L) that effectively maintains the global structure of the entire graph while accurately representing the nodes in each community. A salient point of the proposed model is that it can be applied to any existing GRL model by adopting it as the base model for local and global GRL. Through extensive experiments employing seven popular GRL models, we show that our model outperforms them in both accuracy and efficiency.
更多查看译文
关键词
graph representation learning,community detection,representation accuracy,learning efficiency
AI 理解论文
溯源树
样例
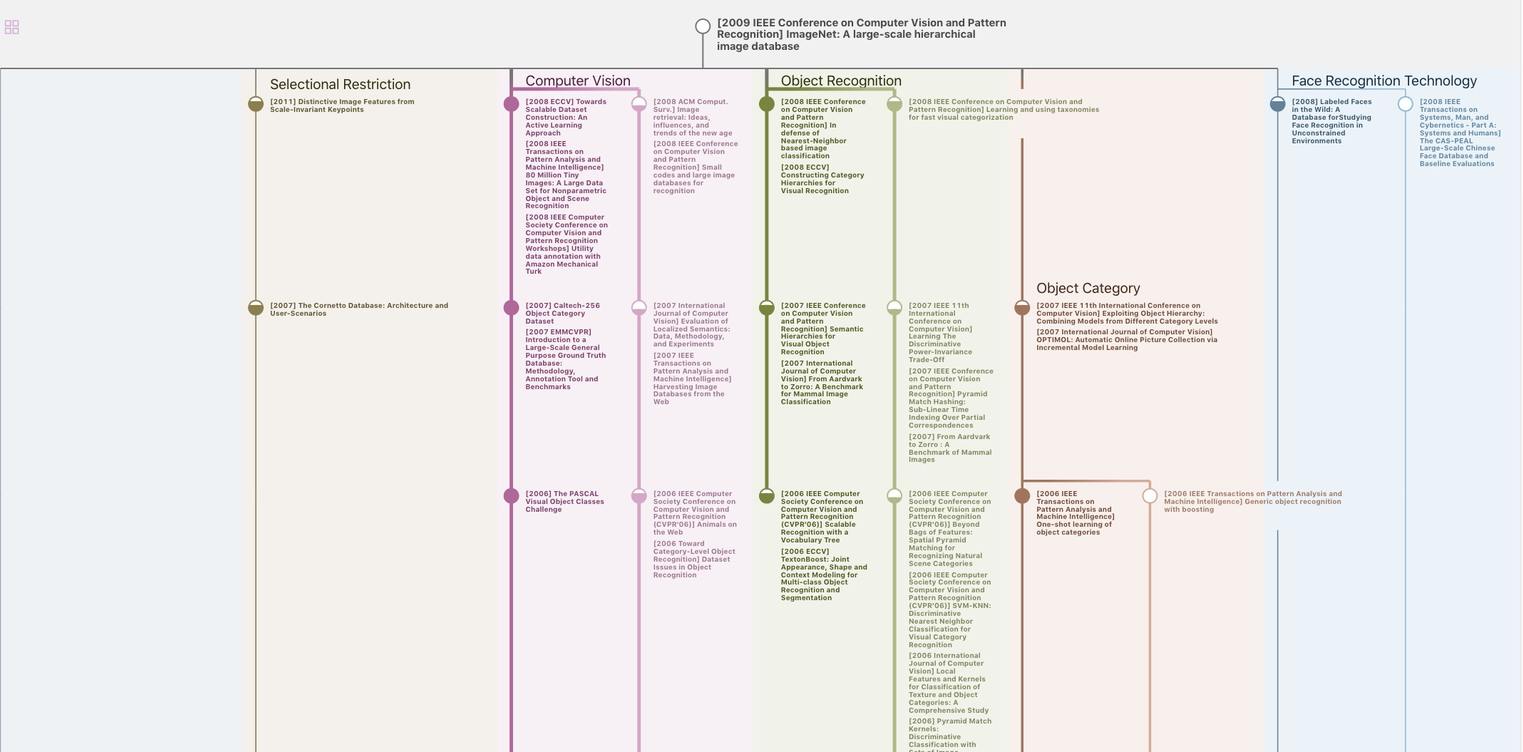
生成溯源树,研究论文发展脉络
Chat Paper
正在生成论文摘要