FASM and FAST-YB: Significant Pattern Mining with False Discovery Rate Control
23RD IEEE INTERNATIONAL CONFERENCE ON DATA MINING, ICDM 2023(2023)
摘要
In significant pattern mining, i.e. the task of discovering structures in data that exhibit a statistically significant association with class labels, it is often needed to have guarantees on the number of patterns that are erroneously deemed as statistically significant by the testing procedure. A desirable property, whose study in the context of pattern mining has been limited, is to control the expected proportion of false positives, often called the false discovery rate (FDR). In this paper, we develop two novel algorithms for mining statistically significant patterns under FDR control. The first one, FASM, builds upon the Benjamini-Yekutieli procedure and exploits the discrete nature of the test statistics to increase its computational efficiency and statistical power. The second one, FAST-YB, incorporates the Yekutieli-Benjamini permutation testing procedure to account for interdependencies among patterns, which allows for a further increase in statistical power. We performed an experimental evaluation on both synthetic and real -world datasets, and the comparisons with state-of-the-art algorithms show that the gains in statistical power are substantial.
更多查看译文
关键词
Data mining,significant pattern mining,false,discovery rate
AI 理解论文
溯源树
样例
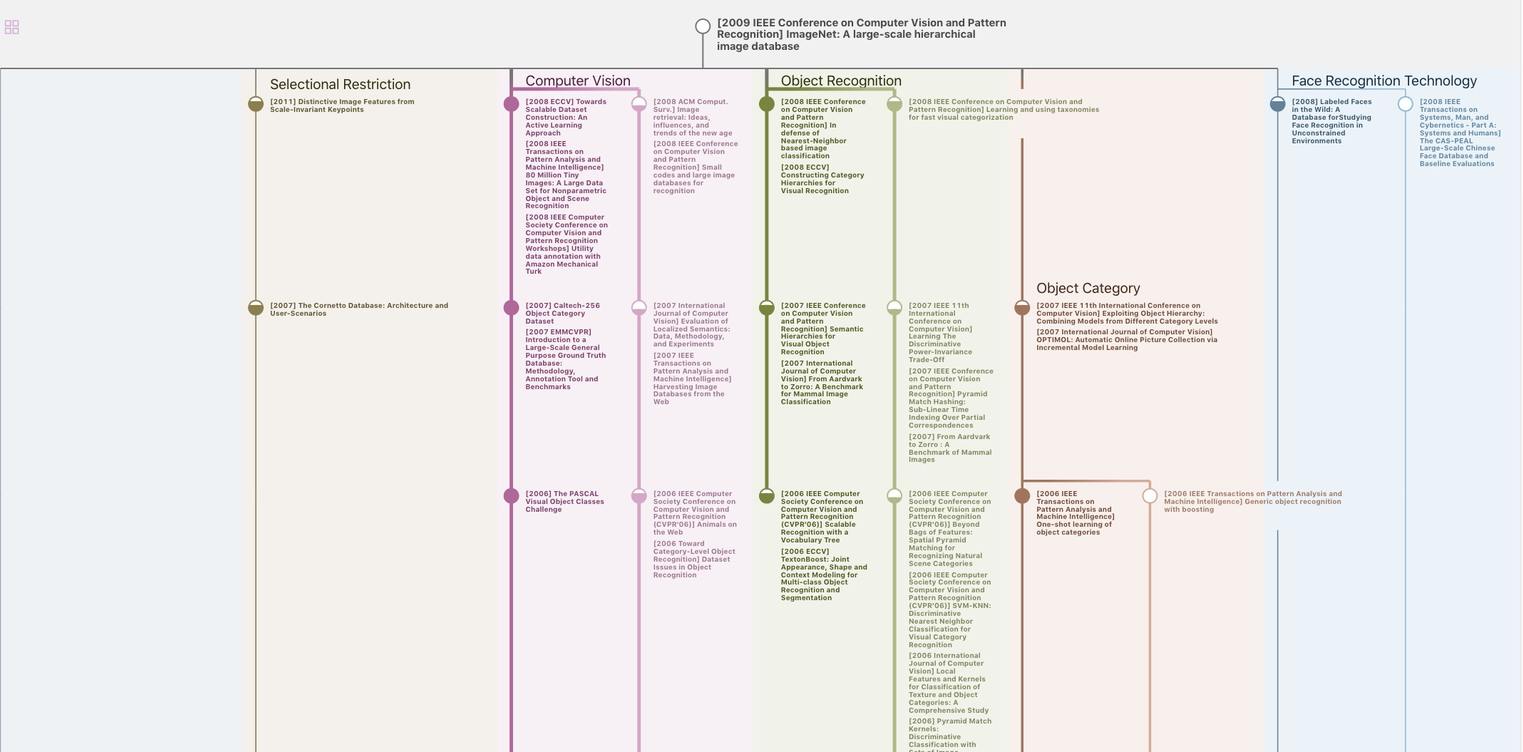
生成溯源树,研究论文发展脉络
Chat Paper
正在生成论文摘要