Learning to Understand: Identifying Interactions via the Mobius Transform
CoRR(2024)
摘要
One of the most fundamental problems in machine learning is finding
interpretable representations of the functions we learn. The Mobius transform
is a useful tool for this because its coefficients correspond to unique
importance scores on sets of input variables. The Mobius Transform is strongly
related (and in some cases equivalent) to the concept of Shapley value, which
is a widely used game-theoretic notion of importance. This work focuses on the
(typical) regime where the fraction of non-zero Mobius coefficients (and thus
interactions between inputs) is small compared to the set of all 2^n possible
interactions between n inputs. When there are K = O(2^n δ) with
δ≤1/3 non-zero coefficients chosen uniformly at random, our
algorithm exactly recovers the Mobius transform in O(Kn) samples and
O(Kn^2) time with vanishing error as K →∞, the first
non-adaptive algorithm to do so. We also uncover a surprising connection
between group testing and the Mobius transform. In the case where all
interactions are between at most t = Θ(n^α) inputs, for α <
0.409, we are able to leverage results from group testing to provide the first
algorithm that computes the Mobius transform in O(Ktlog n) sample complexity
and O(Kpoly(n)) time with vanishing error as K →∞.
Finally, we present a robust version of this algorithm that achieves the same
sample and time complexity under some assumptions, but with a factor depending
on noise variance. Our work is deeply interdisciplinary, drawing from tools
spanning across signal processing, algebra, information theory, learning theory
and group testing to address this important problem at the forefront of machine
learning.
更多查看译文
AI 理解论文
溯源树
样例
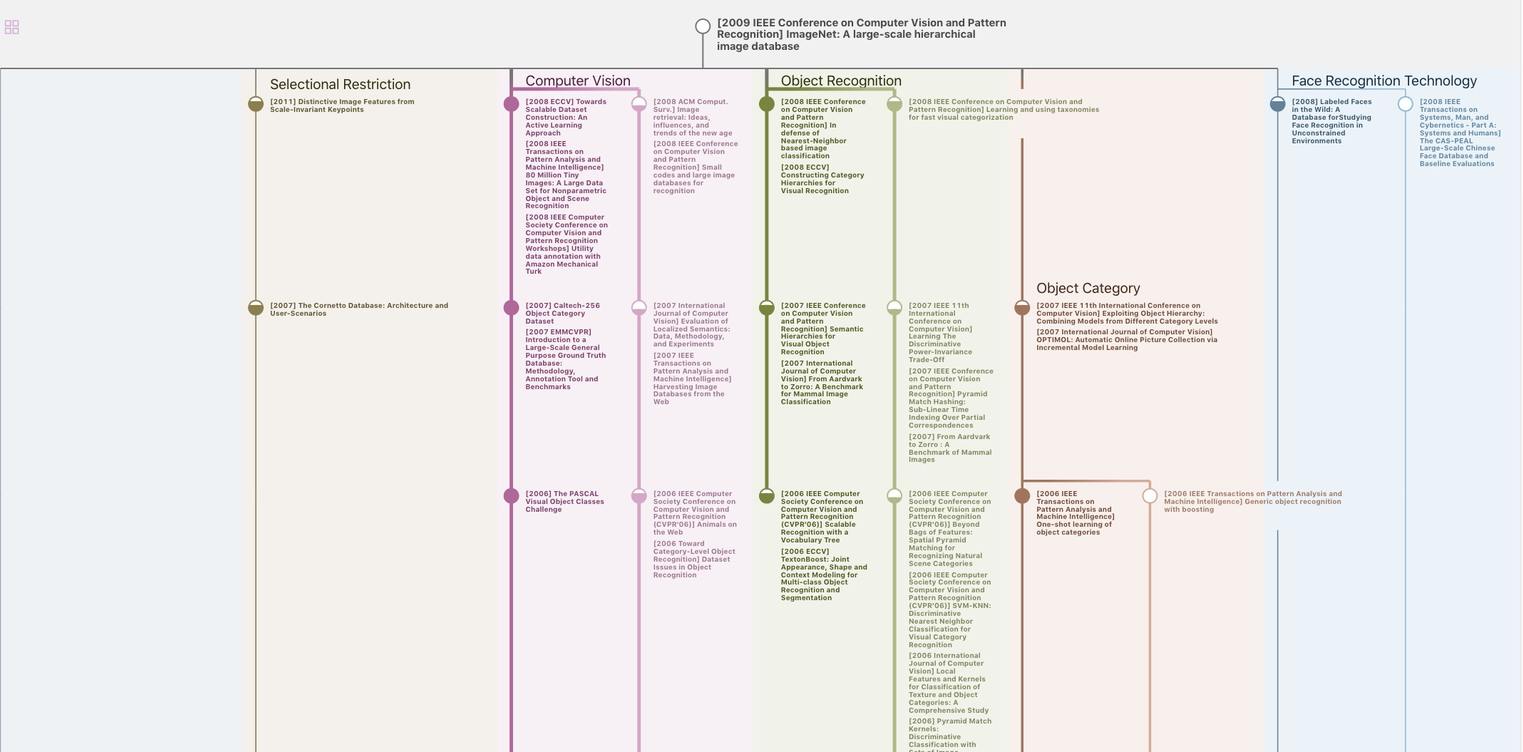
生成溯源树,研究论文发展脉络
Chat Paper
正在生成论文摘要