EMPNet: An extract-map-predict neural network architecture for cross-domain recommendation
World Wide Web(2024)
摘要
Cross-domain recommendation leverages a user’s historical interactions in the auxiliary domain to suggest items within the target domain, particularly for cold-start users with no prior activity in the target domain. Existing cross-domain recommendation models often overlook key aspects such as the complexities of transferring user interests between domains and the biases inherent in user behavior patterns. In contrast, our Extract-Map-Predict Neural Network Architecture (EMPNet) employs a disentanglement approach to map fine-grained user interests and utilize the biases inherent in the cross-domain recommendation. In feature extraction, we use the Bidirectional Encoder Representations from Transformers (BERT) and Identity-Enhanced Multi-Head Attention Mechanism to obtain the user and item feature vectors. In cross-domain user mapping, we disentangle the user feature vector into domain-shared and domain-specific interests for fine-grained cross-domain mapping to obtain the feature vector of cold-start users in the target domain. In rating prediction, we design a biased Attentional Factorization Machine (AFM) to utilize biases extracted from user and item features. We experimentally evaluate EMPNet on the Amazon dataset. The results show that it clearly outperforms the selected baselines.
更多查看译文
关键词
Cross-domain recommendation,Multi-head attention mechanism,VAE,Disentangle,Rating prediction
AI 理解论文
溯源树
样例
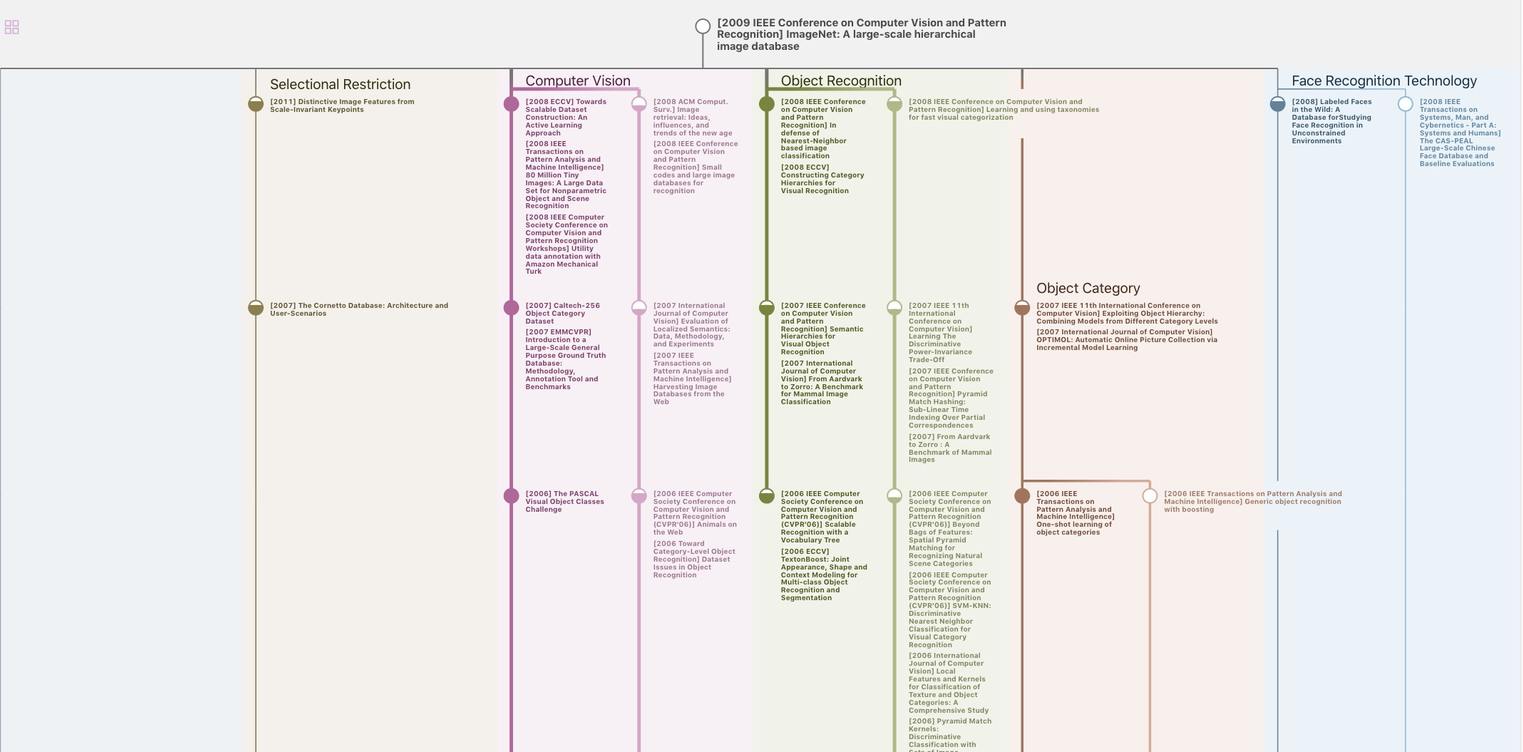
生成溯源树,研究论文发展脉络
Chat Paper
正在生成论文摘要