Applied Data Science for Leasing Score Prediction.
2023 IEEE International Conference on Big Data (BigData)(2023)
摘要
We describe the design, the architecture, and the evaluation of the Leasing Score Prediction (LSP) system - a credit scoring and credit rating system for the leasing sector deployed at the Italian association of leasing companies. Due to its challenging objectives, the design and complexity of the LSP system represent a unique contribution to the best practices in the field. We cover requirements by managers, users, and regulations about rigorous backtesting, statistical validation, calibration, explainability, robustness and uncertainty self-assessment. LSP relies on a machine learning model trained on a mixture of data distributions contributed by many associated leasing companies. We describe the technical solutions adopted and report on their performance evaluation, including the management of the data shifts due to the COVID-19 pandemic.
更多查看译文
关键词
Scoring System,Field Of Practice,Credit Rating,Credit Scoring,Italian Association,Backtesting,Training Set,Risk Score,Score Model,Test Period,Base Classifiers,Credit Risk,Data Warehouse,Financial Indicators,True Results,Operating Region,Shapley Value,Quality Of Input,Low-risk Score,LightGBM,Default Probability,CatBoost,Output Score,Credit Information,Input Instance,Target Market,Natural Person,Quality Labels,Calibration Technique
AI 理解论文
溯源树
样例
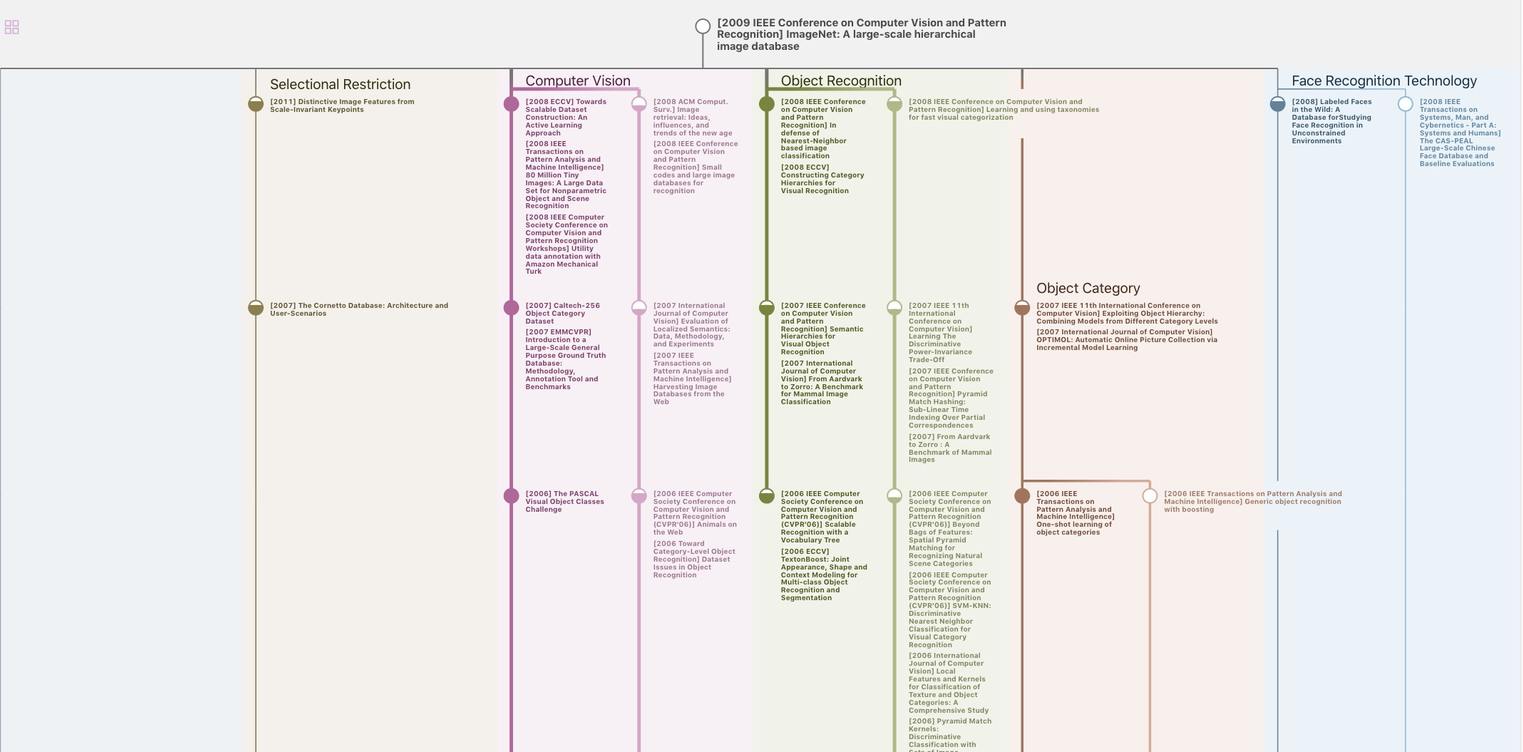
生成溯源树,研究论文发展脉络
Chat Paper
正在生成论文摘要