Adaptive Intrusion Detection Systems: Class Incremental Learning for IoT Emerging Threats.
2023 IEEE International Conference on Big Data (BigData)(2023)
摘要
In the evolving landscape of Internet of Things (IoT) security, the need for continuous adaptation of defenses is critical. Class Incremental Learning (CIL) can provide a viable solution by enabling Machine Learning (ML) and Deep Learning (DL) models to $( i)$ learn and adapt to new attack types (0-day attacks), $( ii)$ retain their ability to detect known threats, (iii) safeguard computational efficiency (i.e. no full re-training). In IoT security, where novel attacks frequently emerge, CIL offers an effective tool to enhance Intrusion Detection Systems (IDS) and secure network environments. In this study, we explore how CIL approaches empower DL-based IDS in IoT networks, using the publicly-available IoT-23 dataset. Our evaluation focuses on two essential aspects of an IDS: $( a)$ attack classification and $( b)$ misuse detection. A thorough comparison against a fully-retrained IDS, namely starting from scratch, is carried out. Finally, we place emphasis on interpreting the predictions made by incremental IDS models through eXplainable AI (XAI) tools, offering insights into potential avenues for improvement.
更多查看译文
关键词
Traffic Classification,Zero-day attacks,Internet of Things,Class Incremental Learning
AI 理解论文
溯源树
样例
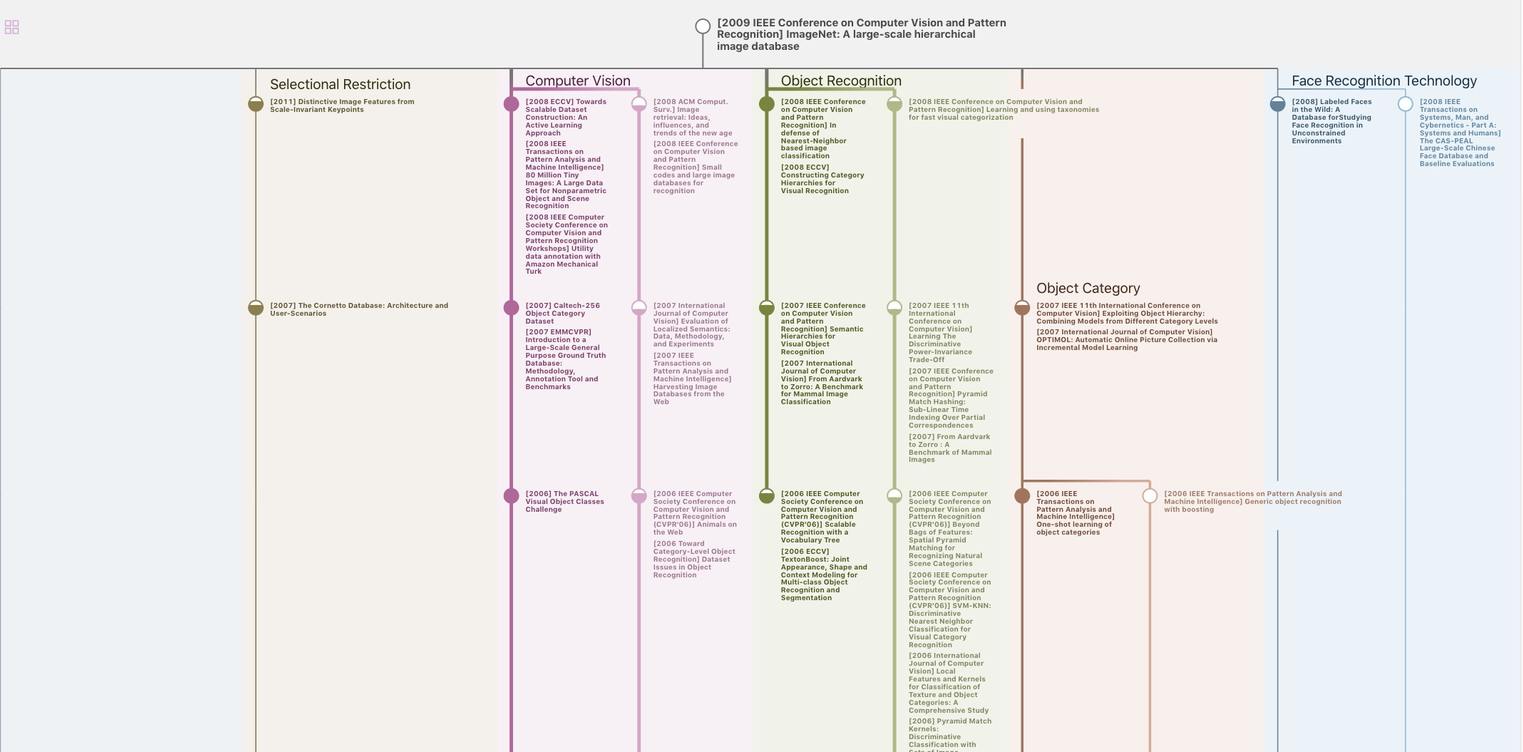
生成溯源树,研究论文发展脉络
Chat Paper
正在生成论文摘要