Learning for Spatio-temporal and Relational Data.
2023 IEEE International Conference on Big Data (BigData)(2023)
摘要
The vast amounts of spatio-temporal data generated by a variety of devices are best utilized in conjunction with relational, tabular data rather than being referenced separately. To enhance the efficiency of data analysis, learned models are frequently used to approximate query results by increasing responsiveness at the cost of some accuracy. Machine learning techniques exist for spatio-temporal data and tabular data separately, but it is not straightforward to represent the combined data in a unified learned model. This paper explores the challenge of learning from heterogeneous data, particularly trajectory and tabular data, and proposes approaches for representation learning using probabilistic circuits and deep neural networks.
更多查看译文
关键词
Approximate Query Processing,Big Data Analysis,Probabilistic Circuits,Sum-Product Networks,SPNs
AI 理解论文
溯源树
样例
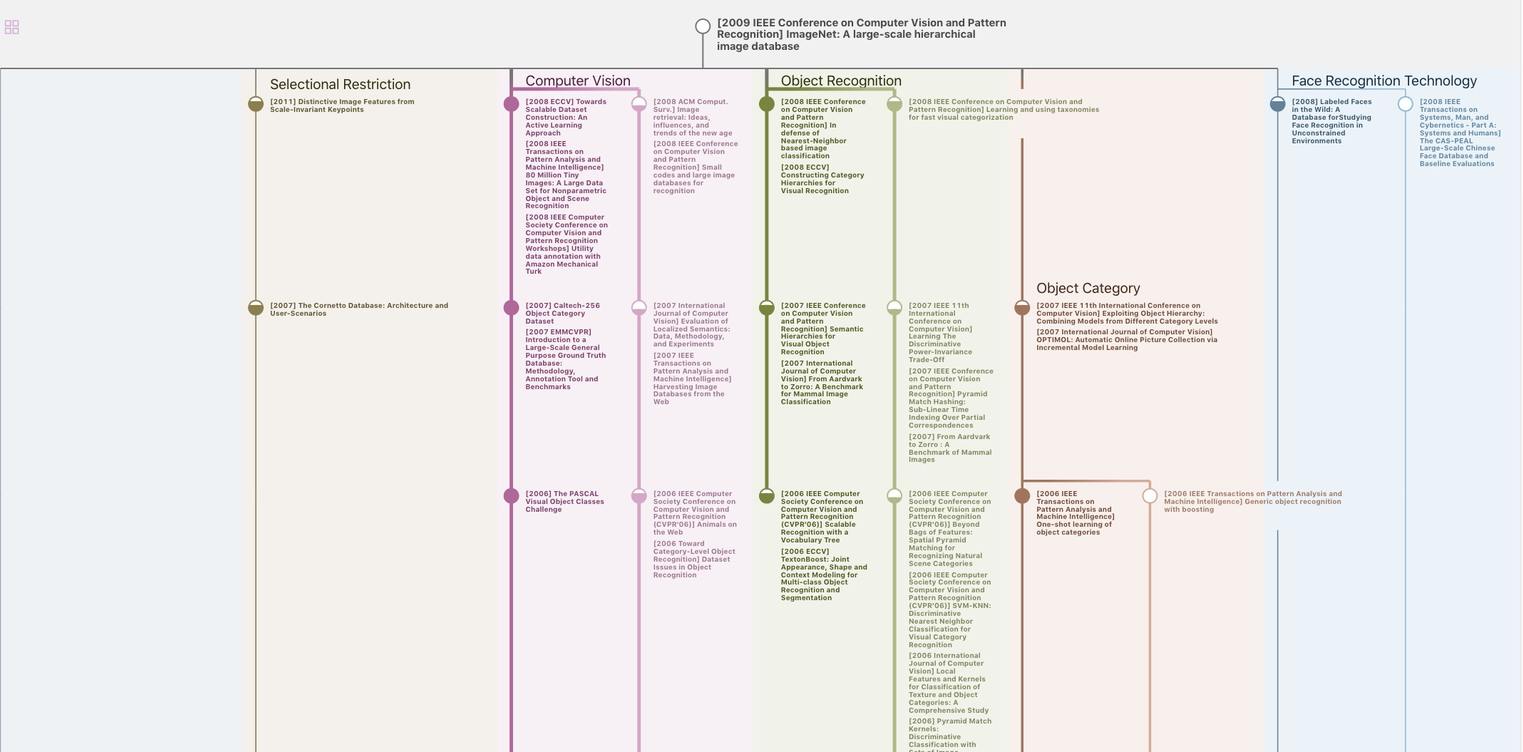
生成溯源树,研究论文发展脉络
Chat Paper
正在生成论文摘要