Collaboration in Federated Learning With Differential Privacy: A Stackelberg Game Analysis
IEEE TRANSACTIONS ON PARALLEL AND DISTRIBUTED SYSTEMS(2024)
摘要
As a privacy-preserving distributed learning paradigm, federated learning (FL) enables multiple client devices to train a shared model without uploading their local data. To further enhance the privacy protection performance of FL, differential privacy (DP) has been successfully incorporated into FL systems to defend against privacy attacks from adversaries. In FL with DP, how to stimulate efficient client collaboration is vital for the FL server due to the privacy-preserving nature of DP and the heterogeneity of various costs (e.g., computation cost) of the participating clients. However, this kind of collaboration remains largely unexplored in existing works. To fill in this gap, we propose a novel analytical framework based on Stackelberg game to model the collaboration behaviors among clients and the server with reward allocation as incentive in FL with DP. We first conduct rigorous convergence analysis of FL with DP and reveal how clients' multidimensional attributes would affect the convergence performance of FL model. Accordingly, we solve the Stackelberg game and derive the collaboration strategies for both clients and the server. We further devise an approximately optimal algorithm for the server to efficiently conduct the joint optimization of the client set selection, the number of global iterations, and the reward payment for the clients. Numerical evaluations using real-world datasets validate our theoretical analysis and corroborate the superior performance of the proposed solution.
更多查看译文
关键词
Federated learning,difference privacy,stackelberg game,discrimination rule
AI 理解论文
溯源树
样例
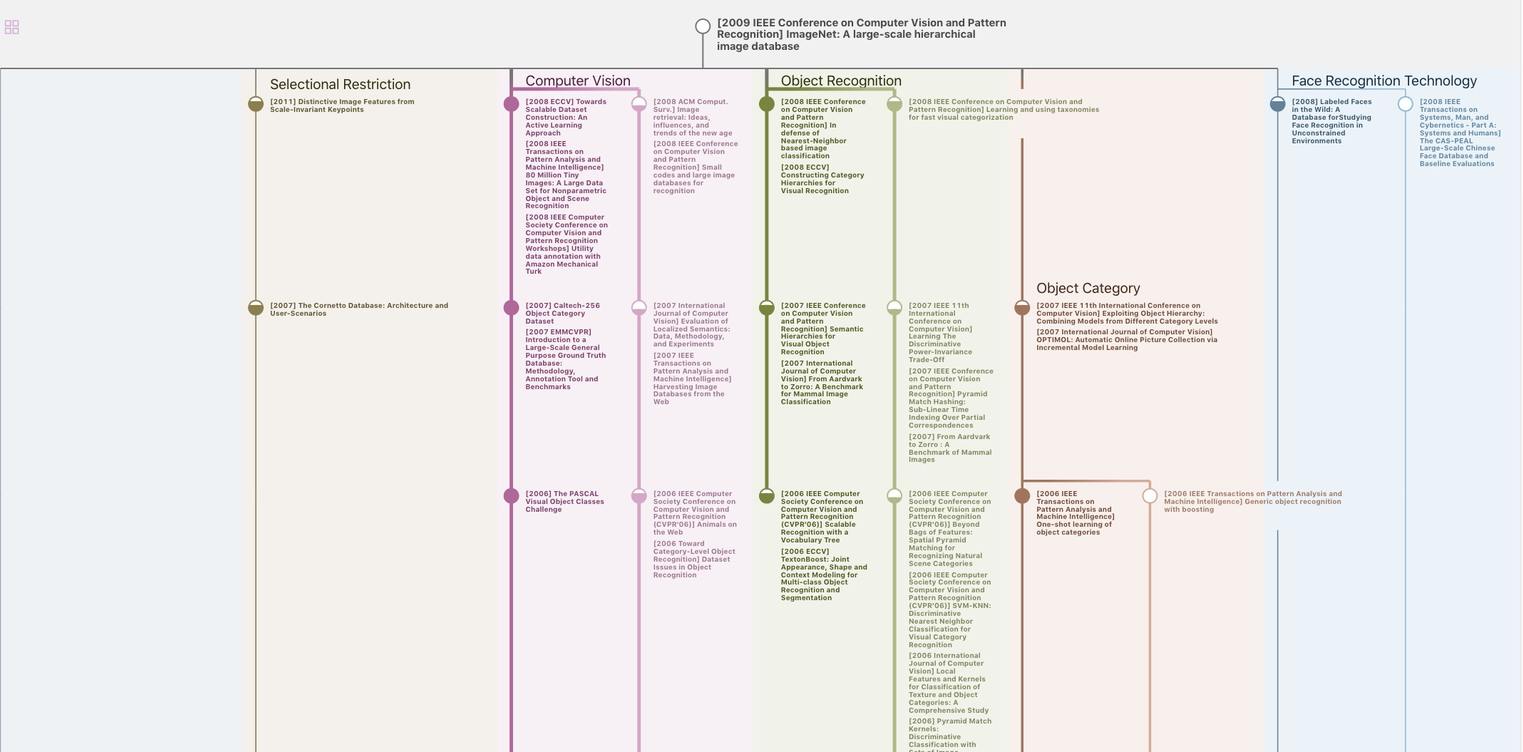
生成溯源树,研究论文发展脉络
Chat Paper
正在生成论文摘要