WindTrans: Transformer-Based Wind Speed Forecasting Method for High-Speed Railway
IEEE TRANSACTIONS ON INTELLIGENT TRANSPORTATION SYSTEMS(2024)
摘要
Wind speed forecasting provides the upcoming wind information and is important to the safe operation of High-Speed Railway (HSR). However, it remains a challenge due to the stochastic and highly varying characteristics of wind. In this paper, we propose a novel Transformer-based method for short-term wind speed forecasting, named WindTrans. Two major cruxes are addressed. First, the task is performed on fine-grained wind speed gathered from multiple sensors. These data present dynamic intra-series and inter-series correlations, which are hard for previous methods to recover. We advance a Transformer-based deep learning model, which has two distinctive characteristics: (1) a graph encoder, which captures the dynamic spatial correlation among wind speeds at different locations, and (2) a temporal decoder to model long sequence wind speed time series, which is resistant to noise in time series. Second, wind speed patterns gradually evolve in long-term periods, thus deactivating prediction models trained on historical data. To tackle this bottleneck, we put forward an experience replay-based scheme to renew the model regularly. To ensure that the renewed model still dominates historical wind patterns, we store and replay only a small portion of historical data named episodic memory. A simple but efficient strategy is designed to constitute episodic memory and thus relieve the computation burden. Experiments conducted on two real-world datasets demonstrate the superiority of our method over existing approaches. Particularly, WindTrans surpasses state-of-the-art methods by up to 36.7%, 29.3% and 13.3% improvement in MAPE measure for 1 hour ahead prediction on 10-minute, 5-minute, and 1-minute-based tasks, respectively. Furthermore, via our continual learning scheme, the model retains competitive performance with only 6.9% datum stored and retrained on.
更多查看译文
关键词
Wind speed,Predictive models,Forecasting,Autoregressive processes,Transformers,Time series analysis,Sensors,High-speed railway,wind speed prediction,continual learning
AI 理解论文
溯源树
样例
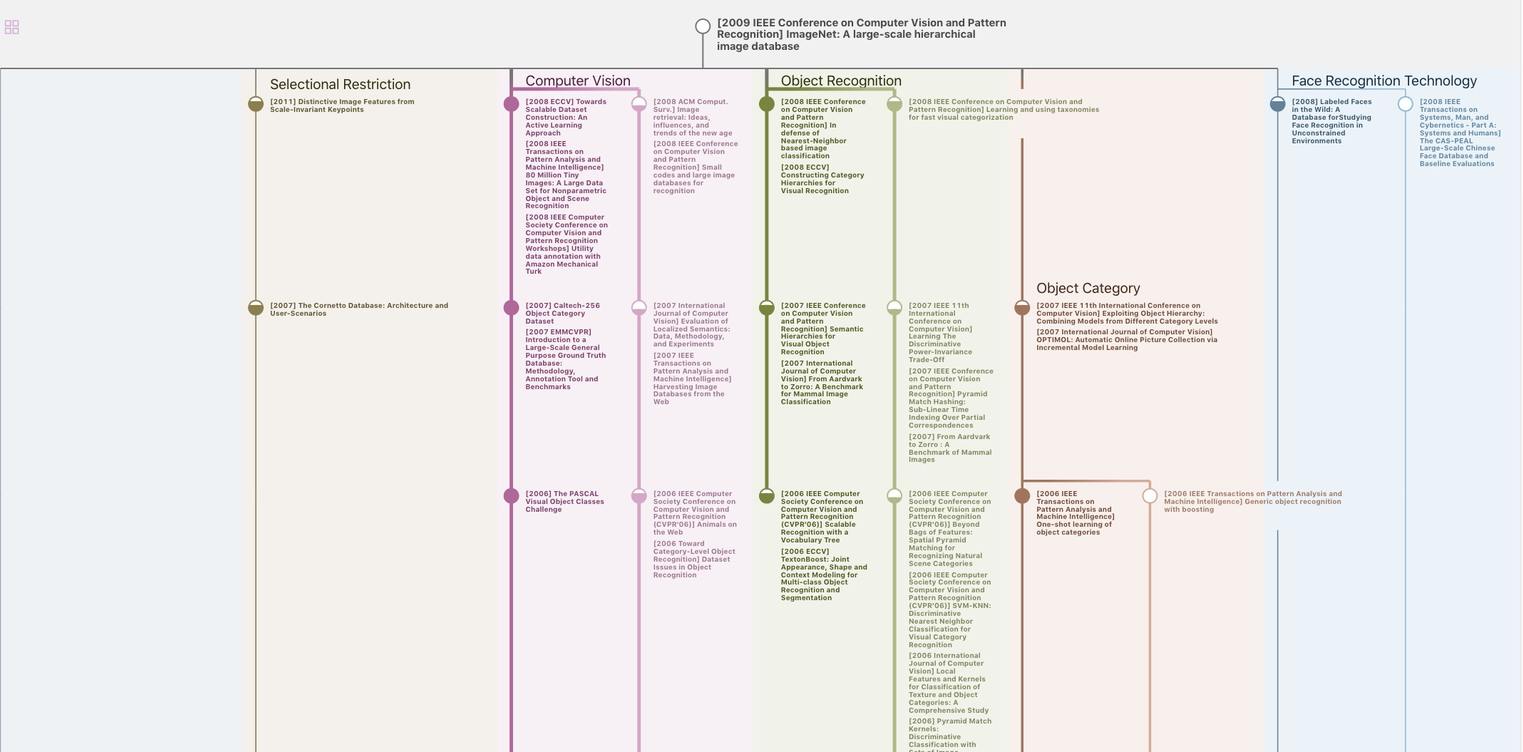
生成溯源树,研究论文发展脉络
Chat Paper
正在生成论文摘要