Fooling Decision-Based Black-Box Automotive Vision Perception Systems in Physical World
IEEE TRANSACTIONS ON INTELLIGENT TRANSPORTATION SYSTEMS(2024)
摘要
Autonomous vehicles use deep neural networks (DNNs) to build powerful vision perception systems, which provide a theoretical foundation for automated vehicle control. Due to the inherent vulnerability of DNNs, many research works have implemented white-box attacks against automotive vision perception systems in the physical world. However, successful black-box attacks (especially decision-based) in the physical world are rarely mentioned because it is difficult to implement a physical-world adversarial attack without internal knowledge about the vision perception systems. In this paper, we propose PRAD, an end-to-end framework that transfers the existing decision-based black-box adversarial attack algorithms (as the backbone of the framework) targeting the digital domain to the physical world for the first time. Specifically, T(center dot ) is first introduced to simulate the real environment changes, e.g., angle, distance, slight shaking, illumination, etc. Then, and crucially, PRAD bridges the non-differentiable black-box attack and the differentiable T(center dot ) by the L-1 loss function. We use the traffic sign recognition system in the vision perception system as an object to conduct comprehensive experiments, including different environmental conditions, black-box attack backbones, models, and datasets. The results demonstrate that the generated adversarial examples in the decision-based black-box setting can fool the commercial traffic sign recognition system into outputting designated misclassifications with high success rates and strong robustness in the physical world (average 90% in target attacks and nearly 100% in non-target attacks), which outperforms the state-of-the-art homogeneous attack methods.
更多查看译文
关键词
Vision perception,black-box attacks,physical world attacks,deep neural networks,autonomous vehicles
AI 理解论文
溯源树
样例
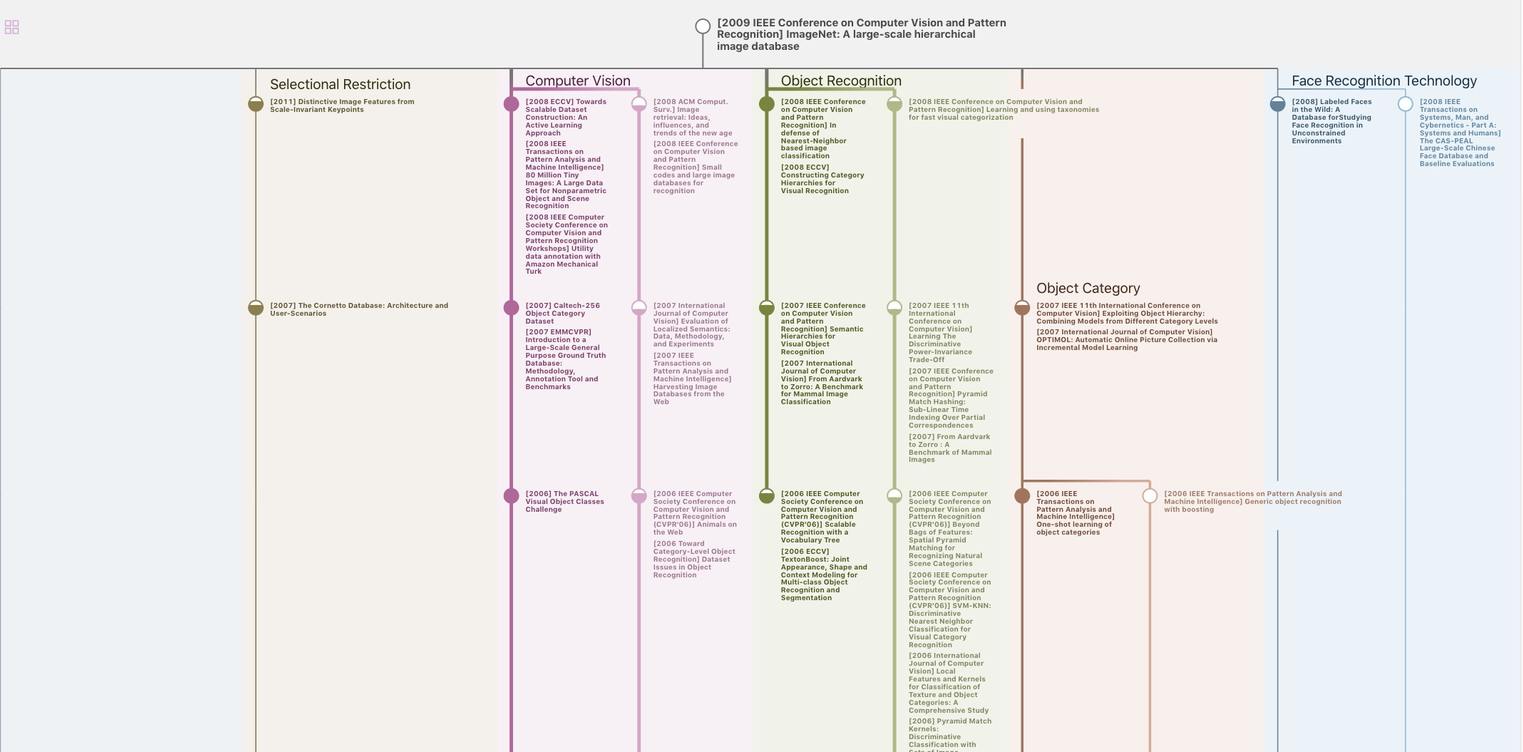
生成溯源树,研究论文发展脉络
Chat Paper
正在生成论文摘要